BE-Net:Boudary-Enhanced Networks for Accurate Gland Segmentation
2022 IEEE 11th Data Driven Control and Learning Systems Conference (DDCLS)(2022)
摘要
The morphology of glands is the gold standard for pathologists to assess the degree of benign and malignant adenocarcinoma in medical diagnosis. Accurate segmentation of glands is indispensable for a computer-aided diagnosis system. However, heterogeneity exists in glandular morphology between benign and malignant, and mutual adhesion of glands adds to the difficulties in determining glandular boundaries. This paper proposes a boundary-enhanced network (BE-Net) that repeatedly uses boundary information to segment glands accurately. The network adopts an encoder-decoder structure that uses the introduced cascaded feature integration module (CFIM) as a decoder to advance aggregate features for explicit boundary refinement. In addition, we also propose a context-augmented attention module (CAAM) to refine implicit boundary and segment the gland boundary accurately through the repeated use of boundary information to improve the overall model's segmentation performance. The experiments demonstrate that the proposed method significantly outperformed other state-of-the-art methods on the 2015 MICCAI Gland Challenge dataset.
更多查看译文
关键词
networks,be-net,boudary-enhanced
AI 理解论文
溯源树
样例
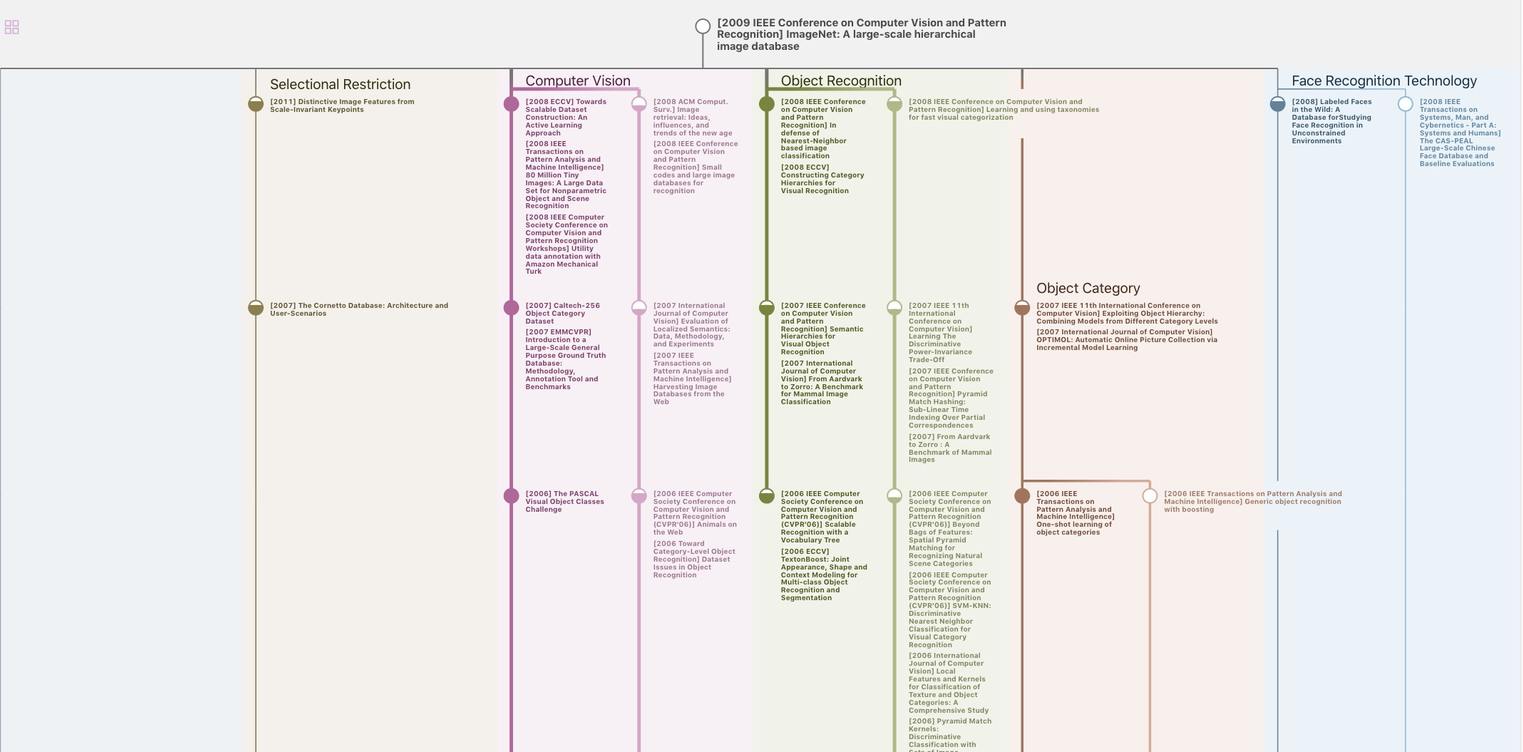
生成溯源树,研究论文发展脉络
Chat Paper
正在生成论文摘要