Morph-KGC: Scalable knowledge graph materialization with mapping partitions.
Semantic Web(2024)
摘要
Knowledge graphs are often constructed from heterogeneous data sources, using declarative rules that map them to a target ontology and materializing them into RDF. When these data sources are large, the materialization of the entire knowledge graph may be computationally expensive and not suitable for those cases where a rapid materialization is required. In this work, we propose an approach to overcome this limitation, based on the novel concept of mapping partitions. Mapping partitions are defined as groups of mapping rules that generate disjoint subsets of the knowledge graph. Each of these groups can be processed separately, reducing the total amount of memory and execution time required by the materialization process. We have included this optimization in our materialization engine Morph-KGC, and we have evaluated it over three different benchmarks. Our experimental results show that, compared with state-of-the-art techniques, the use of mapping partitions in Morph-KGC presents the following advantages: (i) it decreases significantly the time required for materialization, (ii) it reduces the maximum peak of memory used, and (iii) it scales to data sizes that other engines are not capable of processing currently.
更多查看译文
关键词
scalable knowledge graph materialization,mapping,morph-kgc
AI 理解论文
溯源树
样例
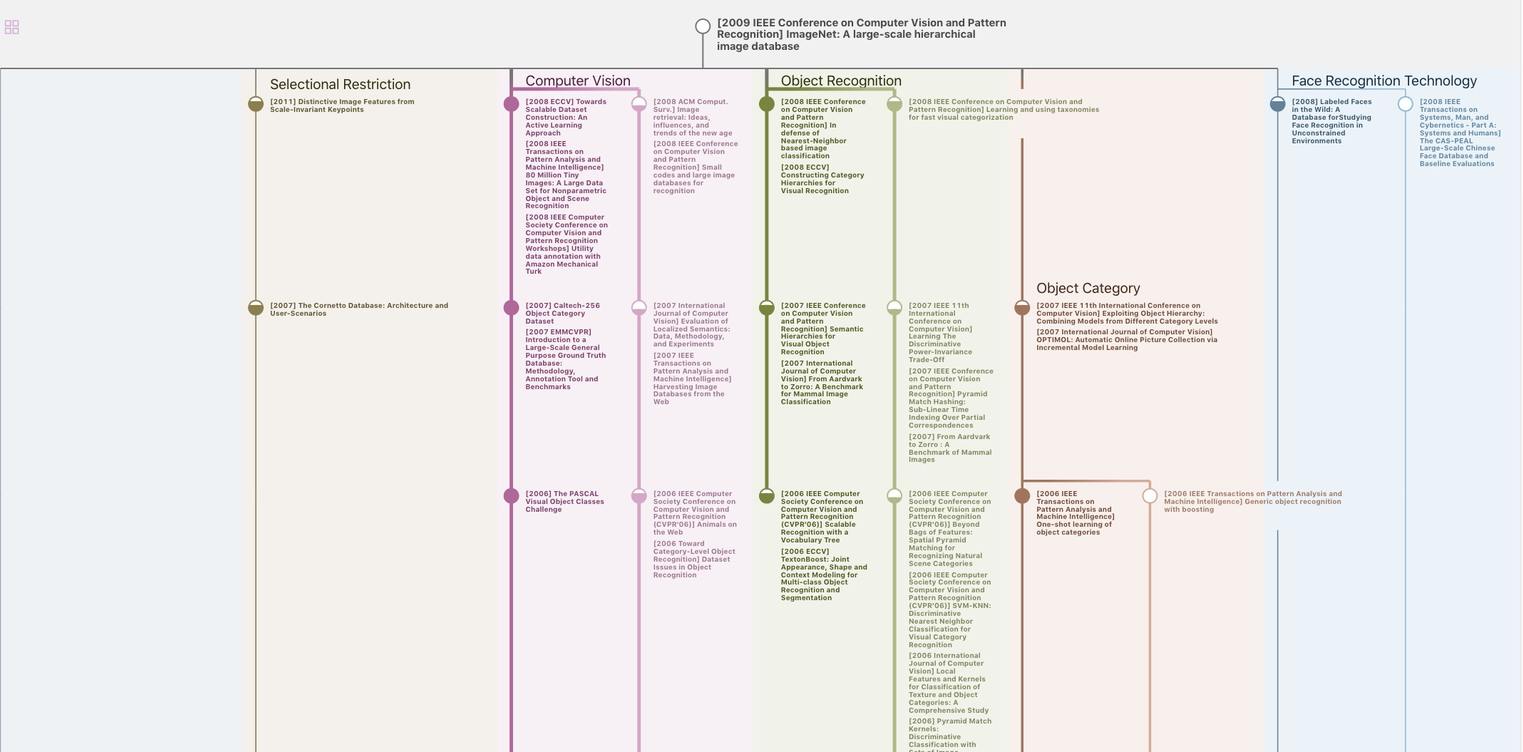
生成溯源树,研究论文发展脉络
Chat Paper
正在生成论文摘要