Predictive models for small-for-gestational-age births in women exposed to pesticides before pregnancy based on multiple machine learning algorithms
FRONTIERS IN PUBLIC HEALTH(2022)
摘要
BackgroundThe association between prenatal pesticide exposures and a higher incidence of small-for-gestational-age (SGA) births has been reported. No prediction model has been developed for SGA neonates in pregnant women exposed to pesticides prior to pregnancy. MethodsA retrospective cohort study was conducted using information from the National Free Preconception Health Examination Project between 2010 and 2012. A development set (n = 606) and a validation set (n = 151) of the dataset were split at random. Traditional logistic regression (LR) method and six machine learning classifiers were used to develop prediction models for SGA neonates. The Shapley Additive Explanation (SHAP) model was applied to determine the most influential variables that contributed to the outcome of the prediction. Results757 neonates in total were analyzed. SGA occurred in 12.9% (n = 98) of cases overall. With an area under the receiver-operating-characteristic curve (AUC) of 0.855 [95% confidence interval (CI): 0.752-0.959], the model based on category boosting (CatBoost) algorithm obtained the best performance in the validation set. With the exception of the LR model (AUC: 0.691, 95% CI: 0.554-0.828), all models had good AUCs. Using recursive feature elimination (RFE) approach to perform the feature selection, we included 15 variables in the final model based on CatBoost classifier, achieving the AUC of 0.811 (95% CI: 0.675-0.947). ConclusionsMachine learning algorithms can develop satisfactory tools for SGA prediction in mothers exposed to pesticides prior to pregnancy, which might become a tool to predict SGA neonates in the high-risk population.
更多查看译文
关键词
small for gestational age, exposure to pesticides, machine learning, prediction, environmental pollution
AI 理解论文
溯源树
样例
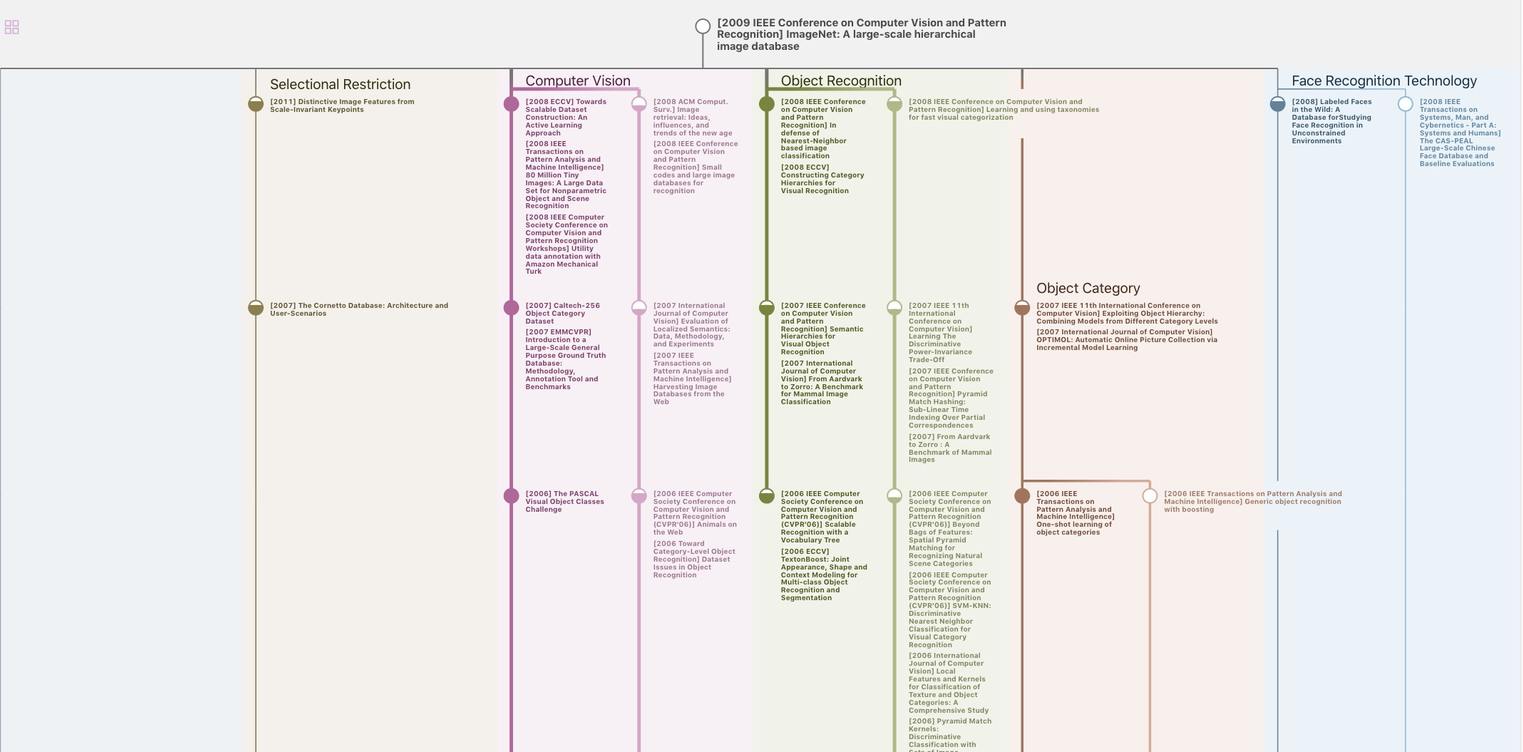
生成溯源树,研究论文发展脉络
Chat Paper
正在生成论文摘要