PDD-Based Decoder for LDPC Codes with Model-Driven Neural Networks
IEEE Communications Letters(2022)
摘要
In this work, we develop a double-loop iterative decoding algorithm for low density parity check (LDPC) codes based on the penalty dual decomposition (PDD) framework. We utilize the linear programming (LP) relaxation and the penalty method to handle the discrete constraints and the over-relaxation method is employed to improve convergence. Then, we unfold the proposed PDD decoding algorithm into a model-driven neural network, namely the learnable PDD decoding network (LPDN). We turn the tunable coefficients and parameters in the proposed PDD decoder into layer-dependent trainable parameters which can be optimized by gradient descent-based methods during network training. Simulation results demonstrate that the proposed LPDN with well-trained parameters is able to provide superior error-correction performance with much lower computational complexity as compared to the PDD decoder.
更多查看译文
关键词
Decoding,Iterative decoding,Signal processing algorithms,Neural networks,Computational complexity,Convex functions,Convergence,LDPC codes,penalty dual decomposition,deep learning,deep unfolding,model-driven
AI 理解论文
溯源树
样例
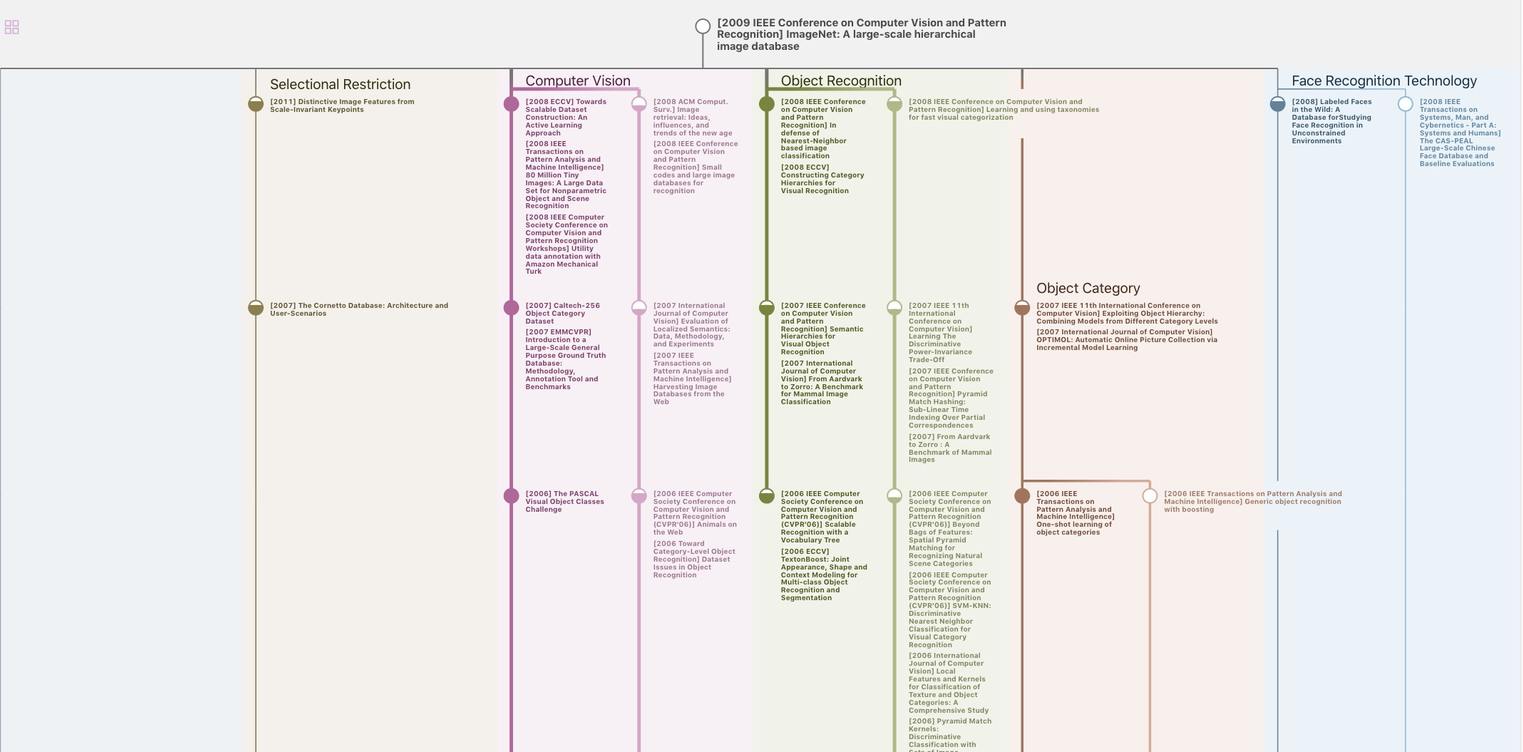
生成溯源树,研究论文发展脉络
Chat Paper
正在生成论文摘要