Dynamic User-Scheduling and Power Allocation for SWIPT Aided Federated Learning: A Deep Learning Approach
IEEE TRANSACTIONS ON MOBILE COMPUTING(2023)
摘要
Federated learning (FL) has been considered as a promising paradigm for enabling distributed machine learning (ML) in wireless networks. To address the limited energy capacity of wireless devices, we propose a simultaneous wireless information and power transfer (SWIPT) aided FL, in which one FL server (FLS) co-located at a cellular base station (BS) uses SWIPT to simultaneously broadcast the global model to wireless user-devices (UDs) and provide wireless power transfer to them. The UDs then use the harvested energy to train their local models and further transmit the local models to the FLS for aggregation. To improve the spectrum efficiency, we consider that the UDs form a non-orthogonal multiple access (NOMA) group for simultaneously sending their local models over the same spectrum channel. Taking the UDs' time-varying available energy and channel conditions into account, we propose a dynamic optimization of the UDs-scheduling, the BS's transmit-power allocation, and the UDs' power-splitting factors for SWIPT, with the objective of minimizing the long-term energy consumption while ensuring the FL convergence. The optimization problem, however, is challenging to solve since it is a finite-horizon dynamic programming problem but with an unknown stopping time, and moreover, the action space covers both discrete and continuous variables. To address these difficulties, we first execute a series of equivalent transformations to reduce the number of decision variables and then formulate the problem as a stochastic shortest path problem, based on which we propose an actor-critic deep reinforcement learning algorithm with the proximal policy optimization to efficiently learn the policy that dynamically adjusts the UDs-scheduling for FL as well as the BS's transmit-power for SWIPT. Numerical results validate the effectiveness and performance of our proposed algorithm. The results demonstrate that our proposed algorithm can effectively reduce the long-term energy consumption in comparison with two baseline algorithms.
更多查看译文
关键词
Optimization,Resource management,NOMA,Training,Heuristic algorithms,Energy consumption,Simultaneous wireless information and power transfer,Federated learning,SWIPT,dynamic user-scheduling,stochastic shortest path,deep reinforcement learning,and proximal policy optimization
AI 理解论文
溯源树
样例
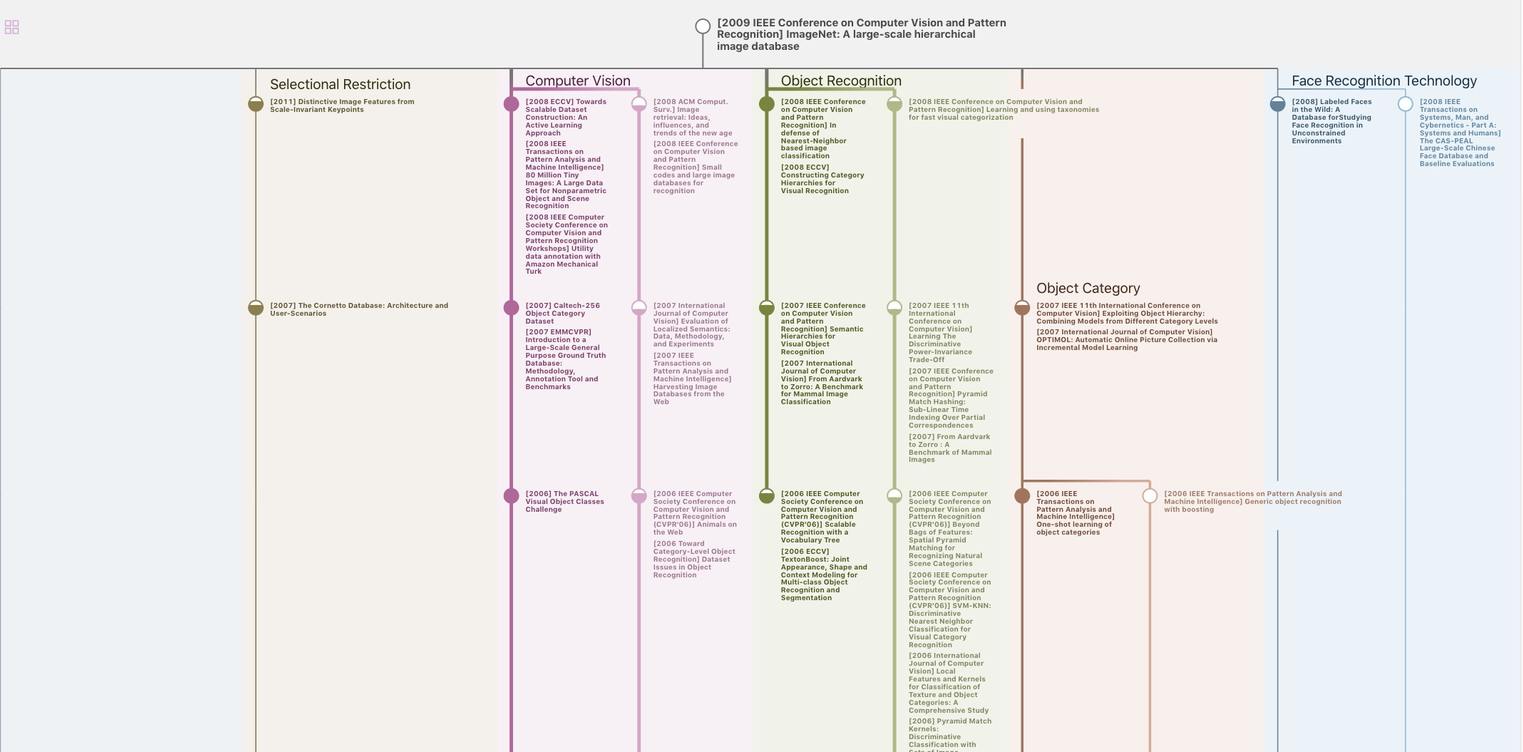
生成溯源树,研究论文发展脉络
Chat Paper
正在生成论文摘要