Revisiting Implicit and Explicit Averaging for Noisy Optimization
IEEE Transactions on Evolutionary Computation(2023)
摘要
Explicit and implicit averaging are two well-known strategies for noisy optimization. Both strategies can counteract the disruptive effect of noise; however, a critical question remains: which one is more efficient? This question has been raised in many studies, with conflicting preferences and, in some cases, findings. Nevertheless, theoretical findings on the noisy sphere problem with additive Gaussian noise supports the superiority of implicit averaging, which may have had a strong impact on the preference of implicit averaging in more recent evolutionary methods for noisy optimization. This study speculates that the analytically supported superiority of implicit averaging relies on specific features of the noisy sphere problem with additive noise, which cannot be generalized to other problems. It enumerates these features and designs controlled numerical experiments to investigate this potential reliance. Each experiment gradually suppresses one specific feature, and the progress rate is numerically calculated for different values of the sample size given a fixed evaluation budget. Our empirical results indicate that for a wide range of noise strength and evaluation budget per iteration, the more these specific features are suppressed, the more the optimal averaging strategy deviates from implicit toward explicit averaging, which confirms our speculations. Consequently, the optimal sample size, which is regarded as the tradeoff between implicit and explicit averaging, depends on the problem characteristics and should be learned during optimization for maximum efficiency.
更多查看译文
关键词
noisy optimization,explicit averaging
AI 理解论文
溯源树
样例
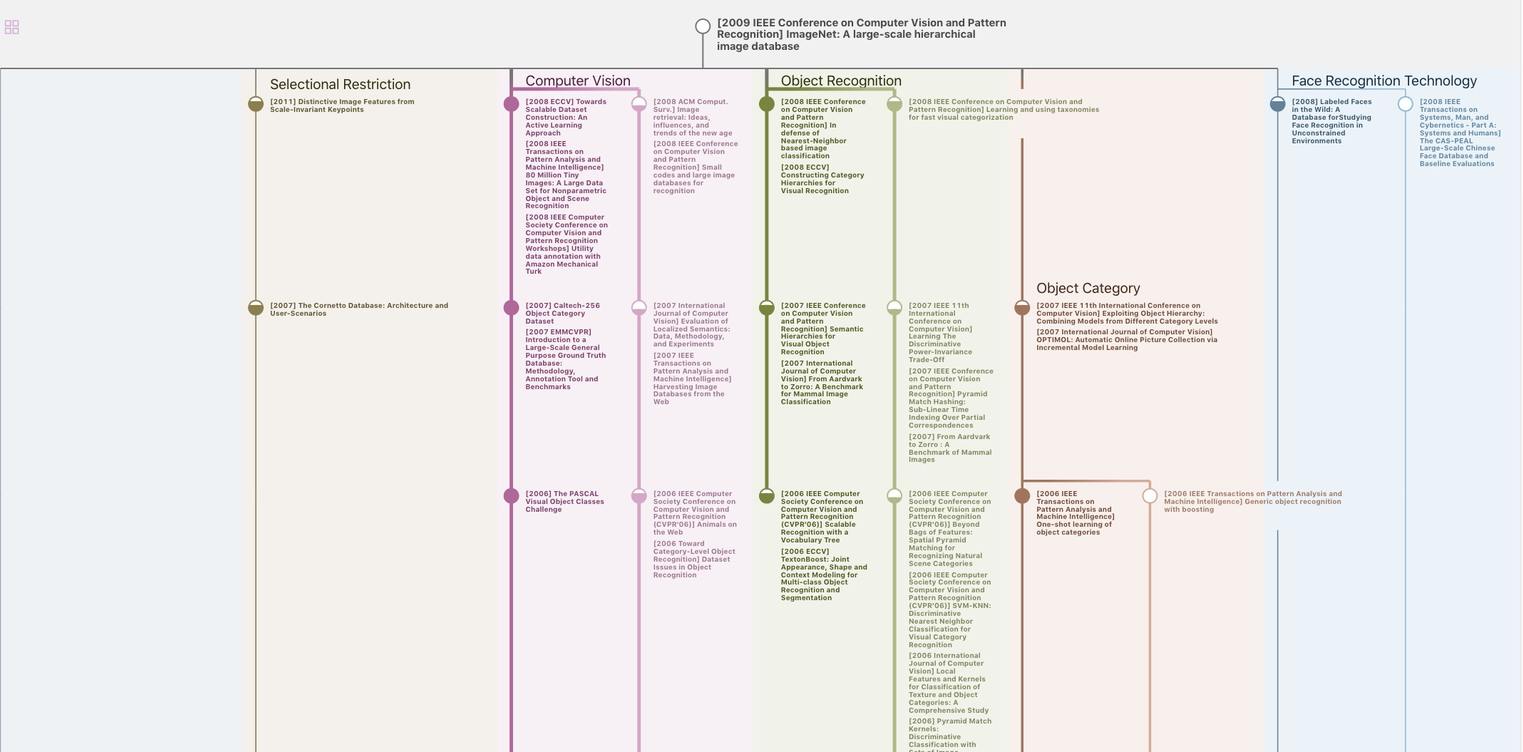
生成溯源树,研究论文发展脉络
Chat Paper
正在生成论文摘要