TEP-GNN: Accurate Execution Time Prediction of Functional Tests Using Graph Neural Networks.
PROFES(2022)
摘要
Predicting the performance of production code prior to actually executing or benchmarking it is known to be highly challenging. In this paper, we propose a predictive model, dubbed TEP-GNN, which demonstrates that high-accuracy performance prediction is possible for the special case of predicting unit test execution times. TEP-GNN uses FA-ASTs, or flow-augmented ASTs, as a graph-based code representation approach, and predicts test execution times using a powerful graph neural network (GNN) deep learning model. We evaluate TEP-GNN using four real-life Java open source programs, based on 922 test files mined from the projects' public repositories. We find that our approach achieves a high Pearson correlation of 0.789, considerable outperforming a baseline deep learning model. However, we also find that more work is needed for trained models to generalize to unseen projects. Our work demonstrates that FA-ASTs and GNNs are a feasible approach for predicting absolute performance values, and serves as an important intermediary step towards being able to predict the performance of arbitrary code prior to execution.
更多查看译文
关键词
Performance,Software testing,Machine learning
AI 理解论文
溯源树
样例
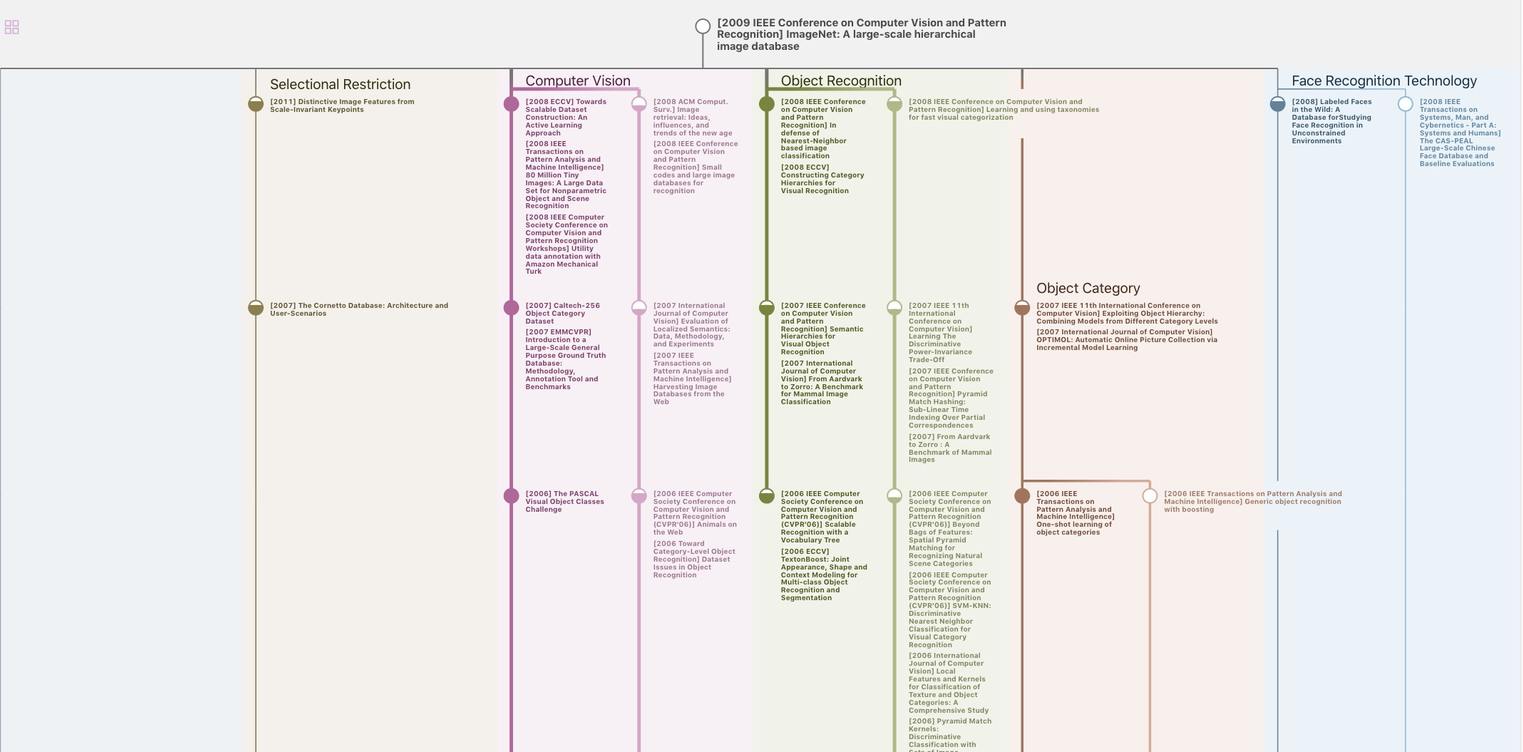
生成溯源树,研究论文发展脉络
Chat Paper
正在生成论文摘要