Efficient Factorisation-based Gaussian Process Approaches for Online Tracking
2022 25th International Conference on Information Fusion (FUSION)(2022)
摘要
Target tracking often relies on complex models with non-stationary parameters. Gaussian process (GP) is a model-free method that can achieve accurate performance. However, the inverse of the covariance matrix poses scalability challenges. Since the covariance matrix is typically dense, direct inversion and determinant evaluation methods suffer from cubic complexity to data size. This bottleneck limits the GP for long-term tracking or high-speed tracking. We present an efficient factorisation-based GP approach without any additional hyperparameters. The proposed approach reduces the computational complexity of the Cholesky decomposition by hierarchically factorising the covariance matrix into off-diagonal low-rank parts. Meanwhile, the resulting low-rank approximated Cholesky factor can also reduce the computation complexity of the inverse and the determinant operations. Numerical results based on offline and online tracking problems demonstrate the effectiveness of the proposed approach.
更多查看译文
关键词
Gaussian process,sensor networks,uncertainty quantification,factorisation,covariance matrix,hierarchical off-diagonal matrix,low-rank approximation,Cholesky factorisation,online tracking
AI 理解论文
溯源树
样例
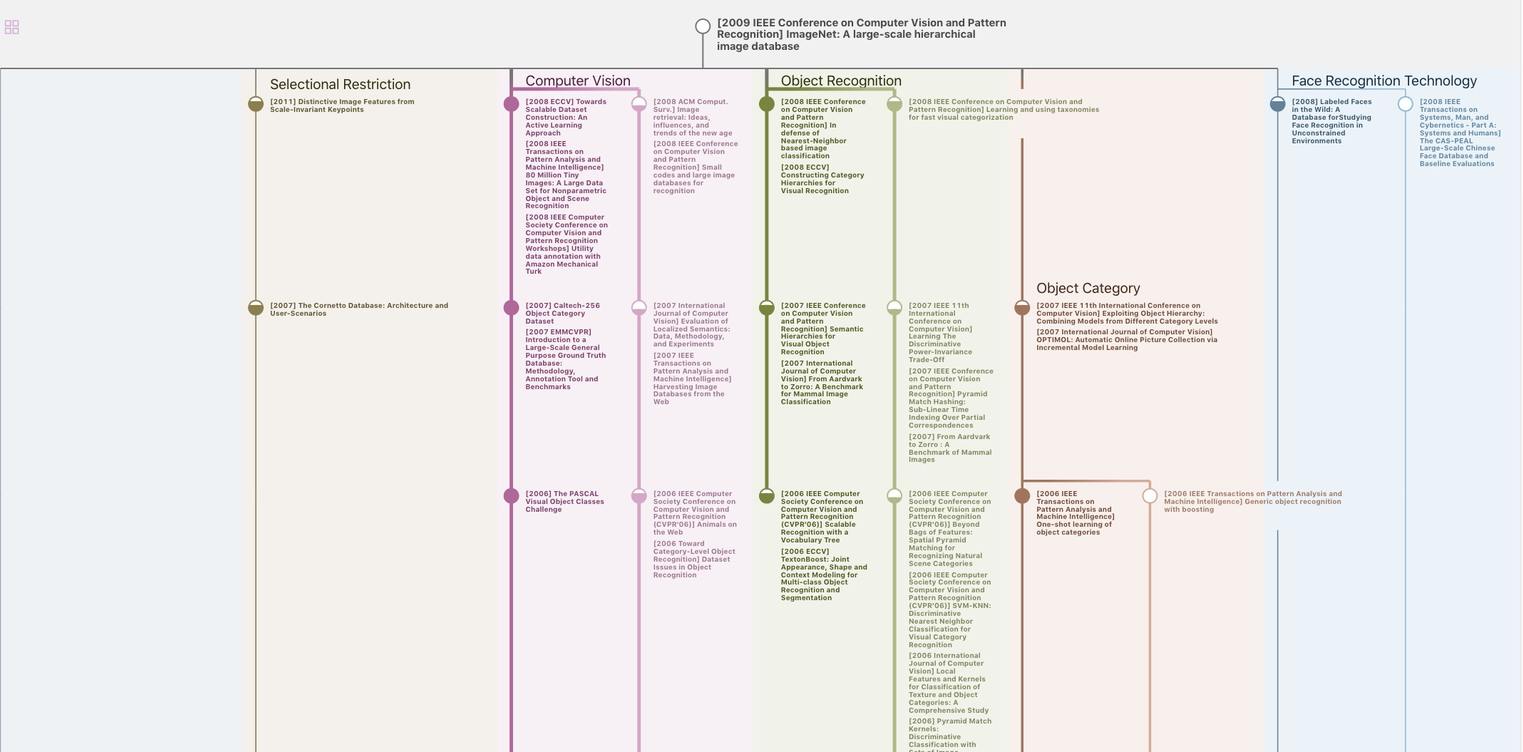
生成溯源树,研究论文发展脉络
Chat Paper
正在生成论文摘要