Hybrid data selection with preservation rough sets
Soft Computing(2022)
摘要
The nearest neighbor classifier is one of the simplest yet accurate decision-making algorithms. However, it suffers in the presence of noisy or redundant data. This article addresses the instance selection problem to improve lazy learners in hybrid and incomplete datasets. It introduces Preservation Rough Set (PRS) model, which can deal with hybrid (numeric and categorical) and incomplete decision systems. The properties of PRS are demonstrated by theorems, and its capabilities are shown by means of an original instance selection algorithm to determine which instances are relevant and which are not to improve decision-making. The numerical experiments conducted allow asseverating that the proposed algorithm is competitive and lead to highly accurate decision-making for nearest neighbor, voting algorithm, and Naïve Associative Classifier. In addition, the experiments show the ability of the proposal for dealing with noisy datasets.
更多查看译文
关键词
Rough sets,Hybrid and incomplete data,Instance selection,Decision-making
AI 理解论文
溯源树
样例
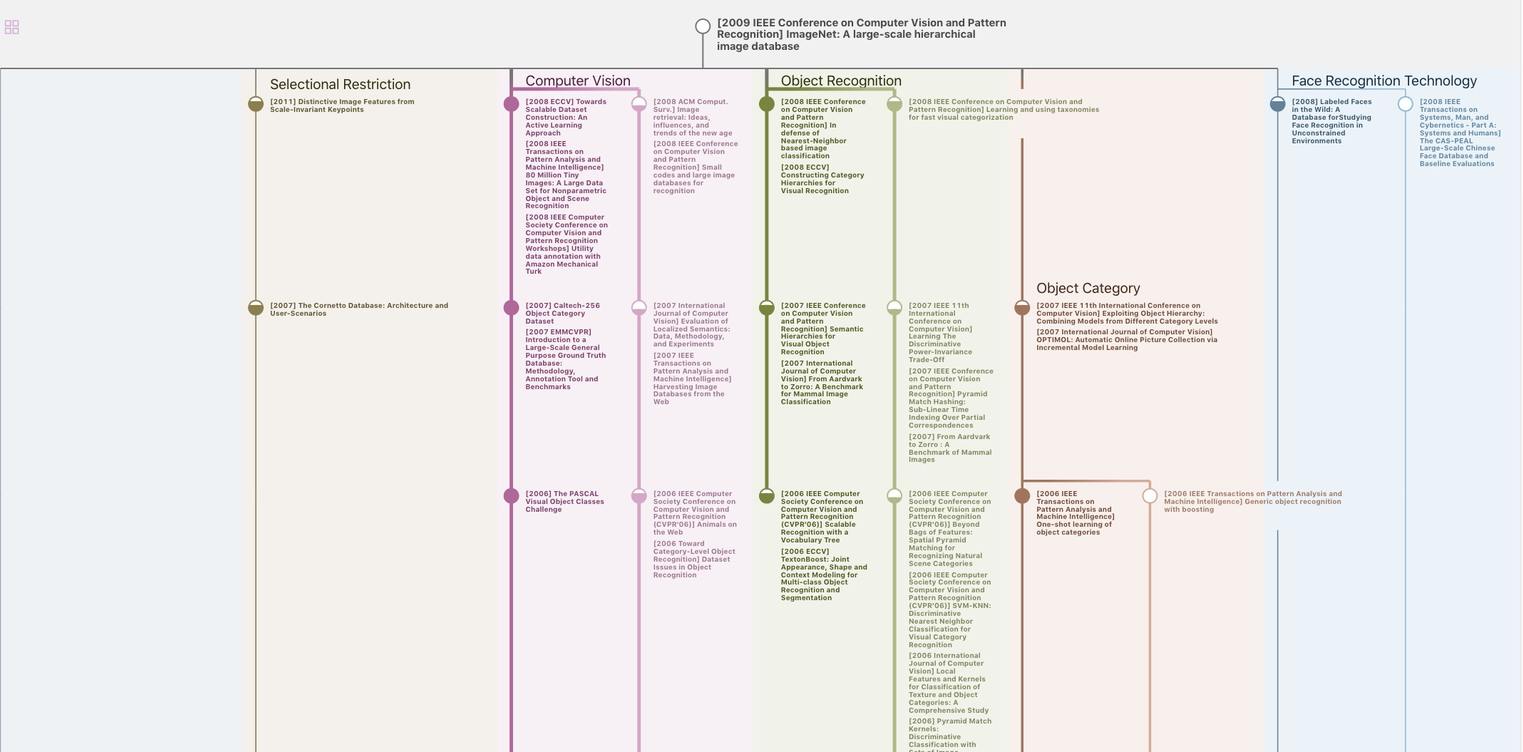
生成溯源树,研究论文发展脉络
Chat Paper
正在生成论文摘要