Communication Efficient Federated Multi-task Learning over Wireless Networks
IEEE Internet of Things Journal(2022)
摘要
This article investigates the scheduling framework of the federated multitask learning (FMTL) problem with a hard-cooperation structure over wireless networks, in which the scheduling becomes more challenging due to the different convergence behaviors of different tasks. Based on the special model structure, we propose a dynamic user and task scheduling scheme with a block-wise incremental gradient aggregation algorithm, in which the neural network model is decomposed into a common feature-extraction module and
$M$
task-specific modules. Different block gradients with respect to different modules can be scheduled separately. We further propose a Lyapunov-drift-based scheduling scheme that minimizes the overall communication latency by utilizing both the instantaneous data importance and the channel state information. We prove that the proposed scheme can converge almost surely to a KKT solution of the training problem such that the data-distortion issue is resolved. Simulation results illustrate that the proposed scheme significantly reduces the communication latency compared to the state-of-the-art baseline schemes.
更多查看译文
关键词
Federated multitask learning (FMTL),Lyapunov analysis,user scheduling,wireless federated learning (FL)
AI 理解论文
溯源树
样例
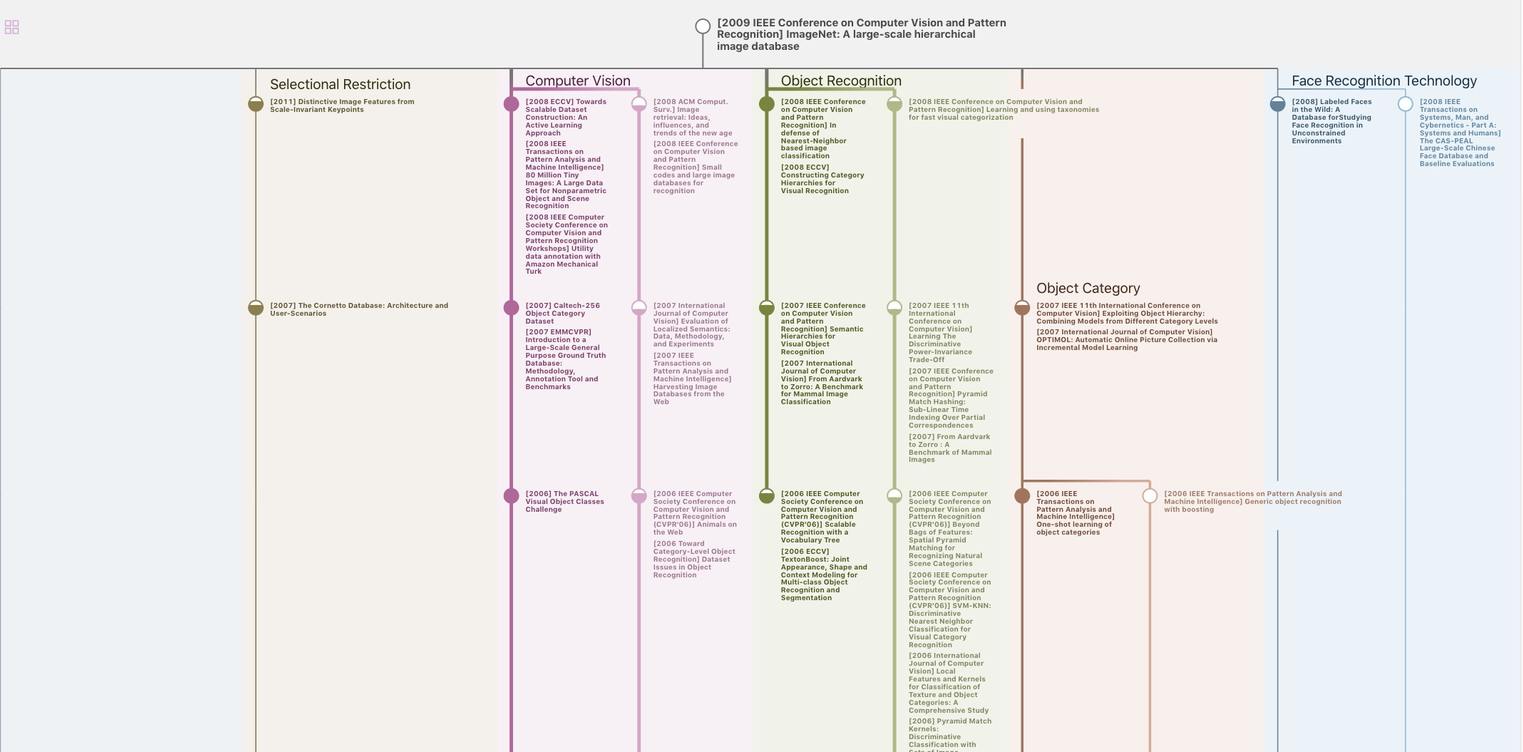
生成溯源树,研究论文发展脉络
Chat Paper
正在生成论文摘要