Prognostic Significance of Tumor-Infiltrating Lymphocytes Using Deep Learning on Pathology Images in Colorectal Cancers
arxiv(2022)
摘要
Purpose Tumor-infiltrating lymphocytes (TILs) have significant prognostic values in cancers. However, very few automated, deep-learning-based TIL scoring algorithms have been developed for colorectal cancers (CRC). Methods We developed an automated, multiscale LinkNet workflow for quantifying cellular-level TILs for CRC tumors using H&E-stained images. The predictive performance of the automatic TIL scores (TIL) for disease progression and overall survival was evaluate using two international datasets, including 554 CRC patients from The Cancer Genome Atlas (TCGA) and 1130 CRC patients from Molecular and Cellular Oncology (MCO). Results The LinkNet model provided an outstanding precision (0.9508), recall (0.9185), and overall F1 score (0.9347). Clear dose-response relationships were observed between TILs and risk of disease progression or death decreased in both TCGA and MCO cohorts. Both univariate and multivariate Cox regression analyses for the TCGA data demonstrated that patients with high TILs had significant (approx. 75%) reduction of risk for disease progression. In both MCO and TCGA studies, the TIL-high group was significantly associated with improved overall survival in univariate analysis (30% and 54% reduction in risk, respectively). However, potential confounding was observed in the MCO dataset. The favorable effects of high TILs were consistently observed in different subgroups according to know risk factors. Conclusion A deep-learning workflow for automatic TIL quantification based on LinkNet was successfully developed.
更多查看译文
关键词
colorectal cancers,deep
AI 理解论文
溯源树
样例
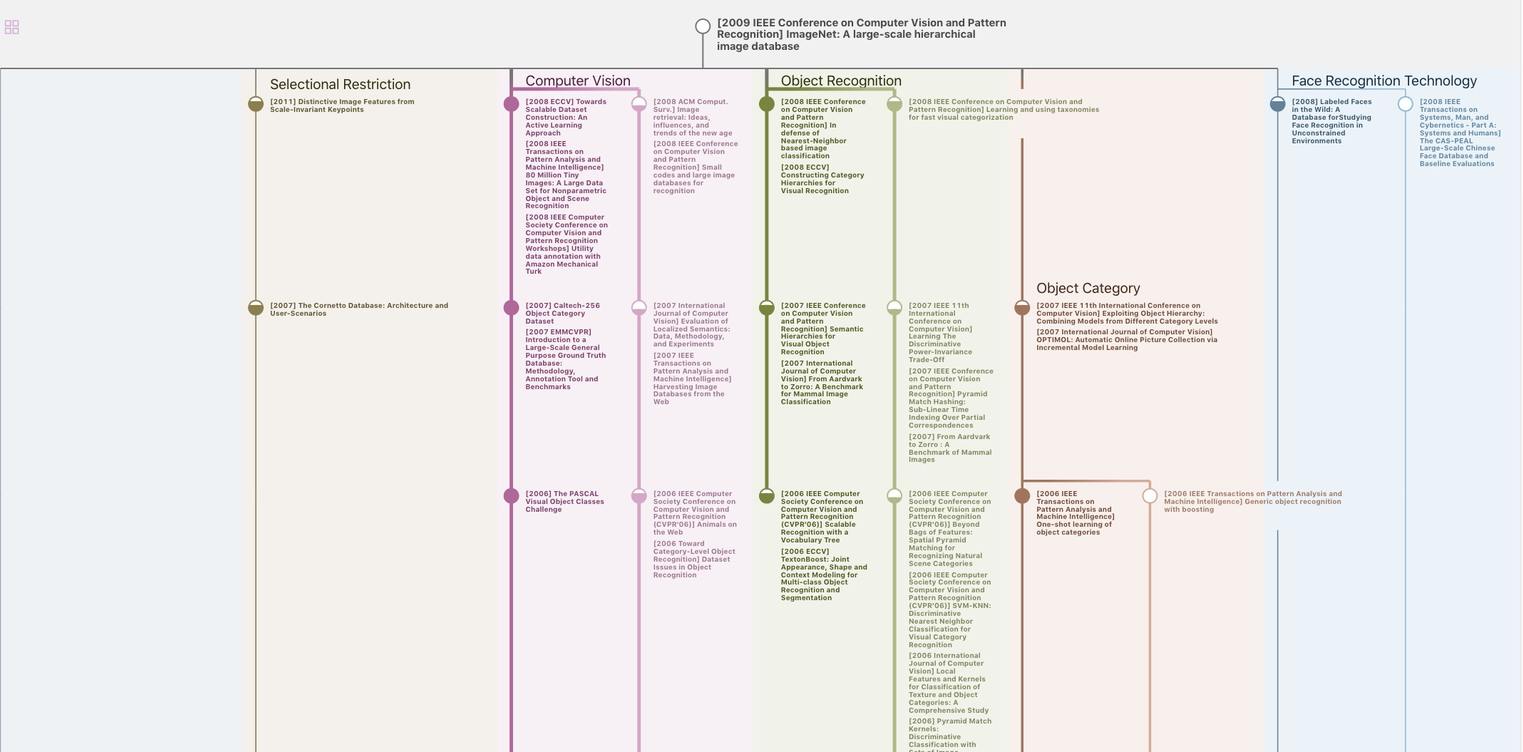
生成溯源树,研究论文发展脉络
Chat Paper
正在生成论文摘要