Online anomaly detection for aeroengine gas path based on piecewise linear representation and support vector data description
IEEE Sensors Journal(2022)
摘要
The online anomaly detection of an aeroengine gas path system is very important to ensure the safety of aircraft. The traditional online anomaly detection method based on a sliding window has many shortcomings, which leads to the lack of accuracy of anomaly detection. In addition, the extreme lack of abnormal samples is also a difficult problem for engine anomaly detection. Therefore, this article proposes an anomaly detection method based on piecewise linear representation (PLR) and support vector data description (SVDD). First, the monitoring data of the aeroengine gas path are segmented and linearly represented so that the data in each segment have basically consistent changing trends, which can make up for the defects of the traditional sliding window. And an improved online segmentation method is proposed that increases the efficiency of the segmentation algorithm. Then, segment features are extracted, and input samples for the anomaly detection model are constructed. The classification model employs SVDD that requires only normal samples. The SVDD model is trained on the offline normal samples and anomaly detection is performed on the online samples. Experimental results show the superiority of this method in aeroengine gas path anomaly detection.
更多查看译文
关键词
Aeroengine,anomaly detection,piecewise linear representation (PLR),support vector data description (SVDD)
AI 理解论文
溯源树
样例
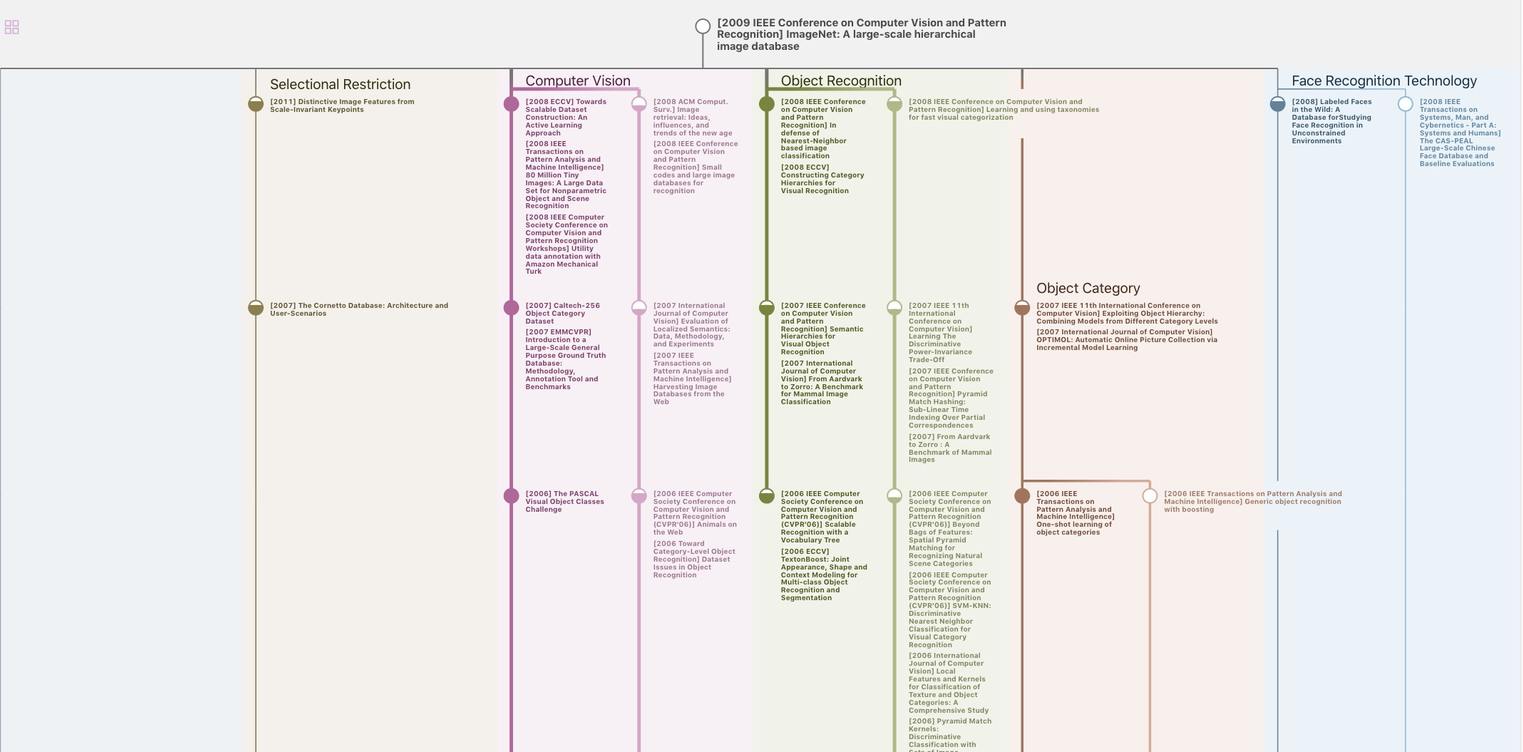
生成溯源树,研究论文发展脉络
Chat Paper
正在生成论文摘要