Spatiotemporal variational neural network for reconstruction of highly accelerated cardiac cine MRI
European Heart Journal - Cardiovascular Imaging(2022)
摘要
Abstract Funding Acknowledgements Type of funding sources: Private company. Main funding source(s): Siemens Healthcare GmbH; NIH Grant. Background Acquisition of cardiac cine MRI usually requires the subject to repeatedly hold their breath for several seconds. Deep learning-based reconstruction of highly undersampled data could allow higher accelerations and has been proposed for several applications. The variational network [1] (VN) was one of the first network architectures for MRI reconstruction, replicating iterative reconstruction with a regularization term learnt from training data. Purpose To expand the concept of VNs to spatiotemporal data from cardiac cine acquisitions. Reconstructions are presented and compared using 3D, 2D spatial-only, and 2D+t spatiotemporal convolutions. Methods A VN with intermediate quantities in k-space [2] was trained to reconstruct cine sequences from retrospectively undersampled data. The network architecture is illustrated in Fig. 1. It consisted of 10 cascades, each resembling a gradient descent step of an iterative reconstruction. Each refinement step contained a Residual U-Net (cf. Fig. 1d). Pre-estimated coil sensitivity maps were used in all transformations between k-space and image space. The convolution operations were implemented as either 2D spatial-only, 3D, or separable 2D+t spatiotemporal convolutions. A 2D+t convolution consisted of a 2D spatial convolution followed by an activation function and a 1D temporal convolution (cf. Fig. 1e). The OCMR dataset [3] was used for training and evaluation. It contains 183 fully sampled cine slices from 74 subjects in different imaging planes with 15 to 38 coils and 15 to 31 temporal frames at 1.5T and 3T. The training set contained 142 slices from 33 individuals, which includes all multi-slice acquisitions from the dataset. The validation set consisted of 22 single slices and the network performance was evaluated on the remaining, previously unseen 19 single-slice acquisitions. The k-space data was retrospectively undersampled using a variable density mask [4]. Sensitivity-based coil combination of the fully sampled k-space was used as ground truth. Coil sensitivity maps were estimated with ESPIRiT. Six networks were trained using the different convolution types mentioned above, each for acceleration factors R=8 and R=12. Results Exemplary reconstructions and quantitative results are given in Fig. 2. At R=8, reconstruction with 2D+t and 3D convolutions reached high structural similarity (SSIM) scores of around 0.95, while the 2D convolutions only reached 0.74. Artifacts are clearly visible in reconstructions learnt with 2D kernels. At R=12, reconstruction quality degraded most considerably with 2D convolution kernels. Conclusion Reconstruction of highly accelerated cardiac cine data using VNs yielded very good preliminary results. Convolutions that exploit temporal correlations are clearly beneficial over spatial-only convolutions. 2D+t spatiotemporal convolutions may be preferable over 3D convolutions due to reduced model capacity with similar reconstruction capability. Network design with 2D+t convolutionExample reconstruction and time profiles
更多查看译文
关键词
spatiotemporal variational neural network,mri,neural network
AI 理解论文
溯源树
样例
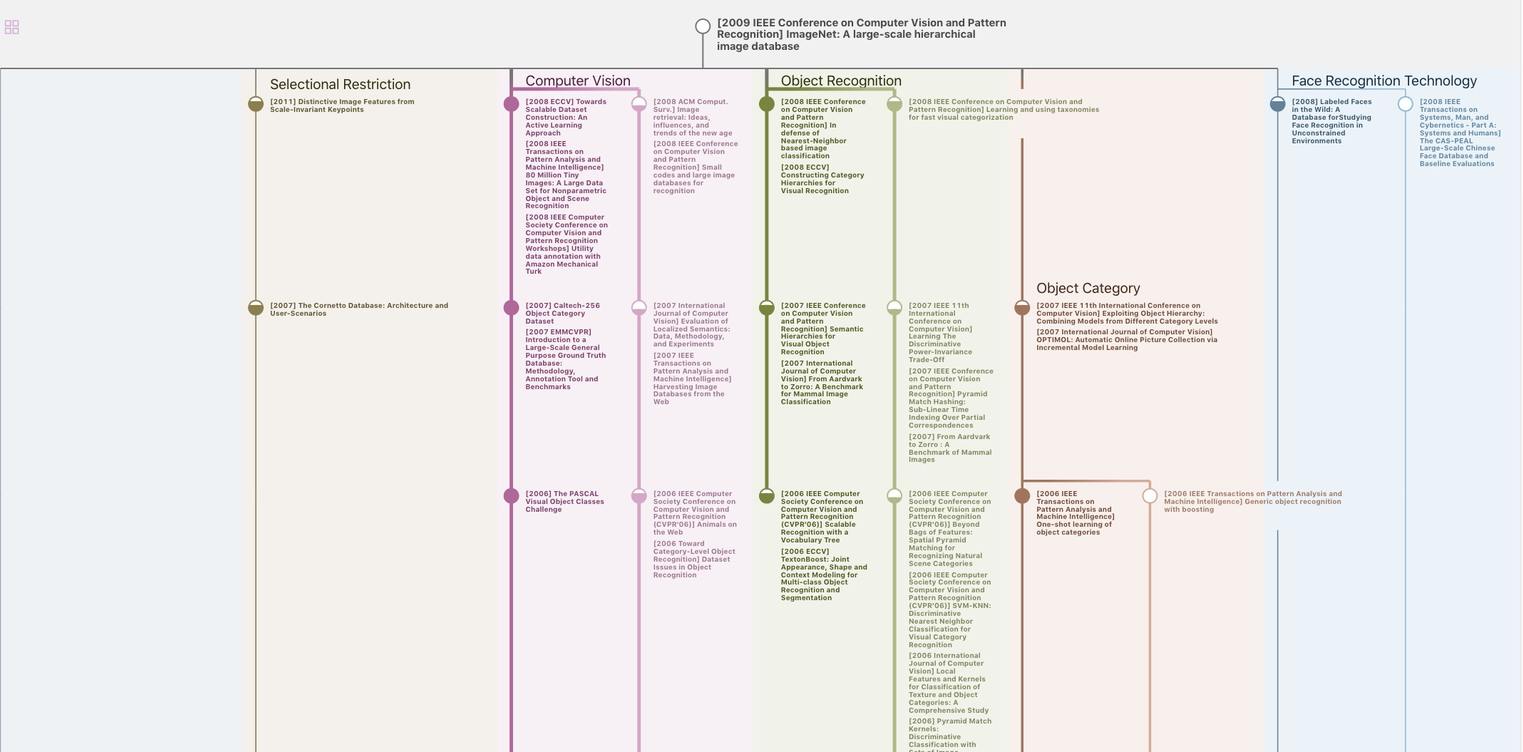
生成溯源树,研究论文发展脉络
Chat Paper
正在生成论文摘要