A transformer-based low-resolution face recognition method via on-and-offline knowledge distillation
Neurocomputing(2022)
摘要
It has been widely noticed the performance of algorithms for high-resolution face recognition (HRFR) degrades significantly for low-resolution face recognition (LRFR). In this paper, we discover the main source of this performance degradation comes from the human-defined inductive bias of CNN, which constrains the model to absorb effective information and leads to overfitting. To overcome the shortcoming, for the first time, we adopt a transformer-based DNN algorithm named DeiT to accomplish LRFR tasks. On the other hand, we further borrow the form of knowledge distillation. Traditional knowledge distillation network for LRFR sets the student model to be simpler than or the same as the teacher model while using the teacher model off-the-shelf, leading to the model capacity gap. Instead, we fuse an online network into the original parameter-fixed teacher model to learn how to transfer knowledge. The final “knowledge” is the sum fusion of the outputs of both the teacher model and the student model.
更多查看译文
关键词
Face recognition,Low resolution,Knowledge Distillation,Transformer
AI 理解论文
溯源树
样例
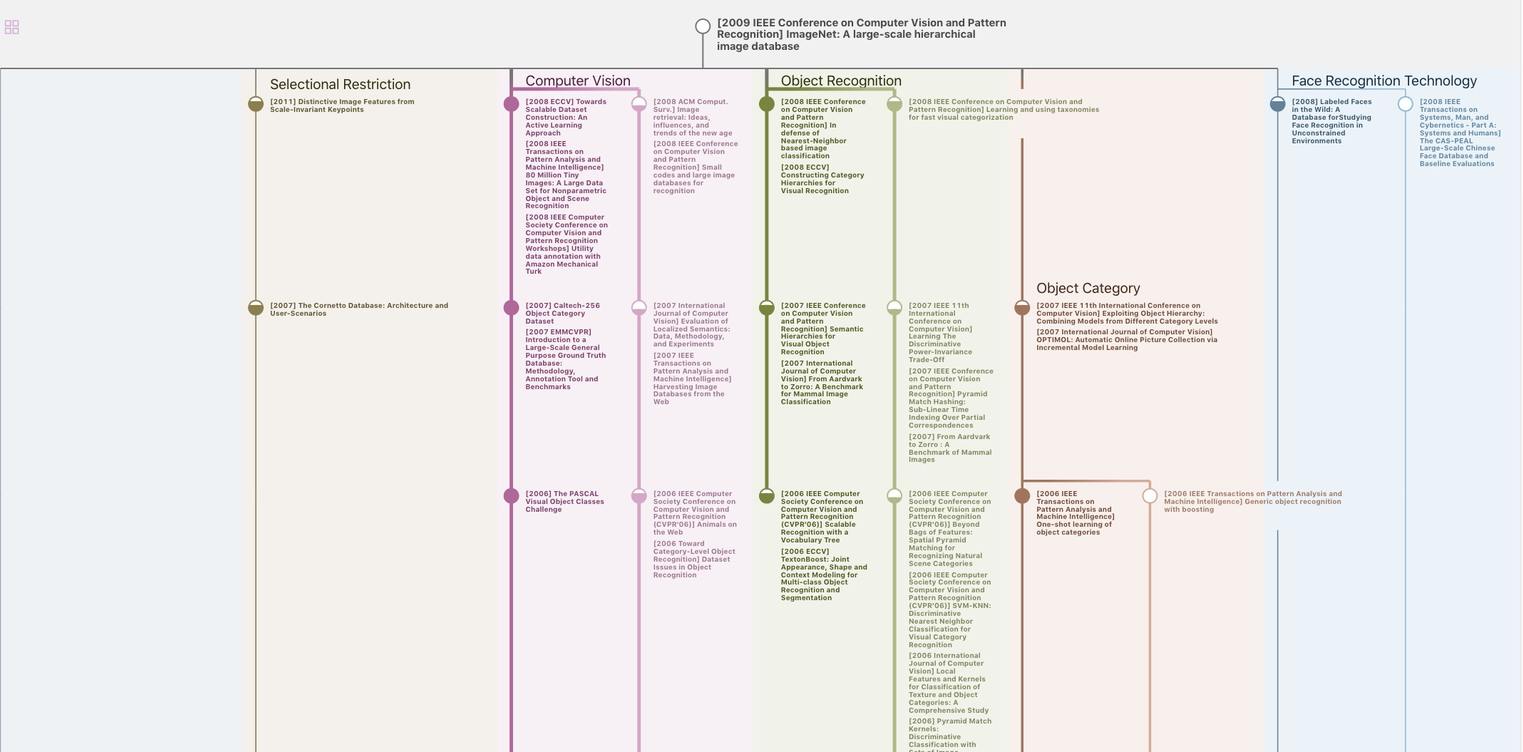
生成溯源树,研究论文发展脉络
Chat Paper
正在生成论文摘要