Event-Triggered Time-Varying Bayesian Optimization
arxiv(2023)
摘要
We consider the problem of sequentially optimizing a time-varying objective function using time-varying Bayesian optimization (TVBO). Here, the key challenge is the exploration-exploitation trade-off under time variations. Current approaches to TVBO require prior knowledge of a constant rate of change. However, the rate of change is usually neither known nor constant. We propose an event-triggered algorithm, ET-GP-UCB, that treats the optimization problem as static until it detects changes in the objective function online and then resets the dataset. This allows the algorithm to adapt to realized temporal changes without the need for prior knowledge. The event-trigger is based on probabilistic uniform error bounds used in Gaussian process regression. We provide regret bounds for ET-GP-UCB and show in numerical experiments that it is competitive with state-of-the-art algorithms even though it requires no knowledge about the temporal changes. Further, ET-GP-UCB outperforms these baselines if the rate of change is misspecified, and we demonstrate that it is readily applicable to various settings without tuning hyperparameters.
更多查看译文
关键词
bayesian optimization,event-triggered,time-varying
AI 理解论文
溯源树
样例
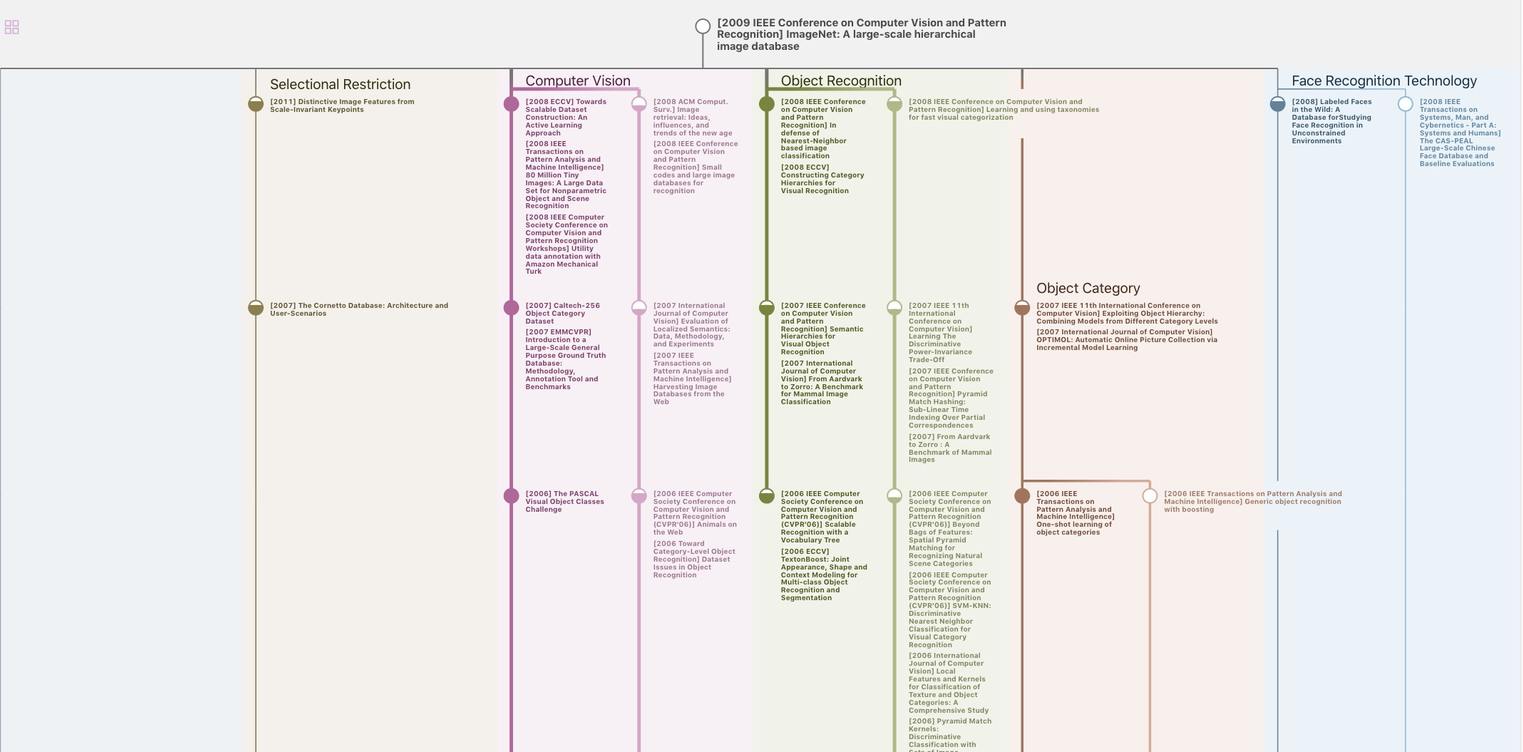
生成溯源树,研究论文发展脉络
Chat Paper
正在生成论文摘要