Survey on Evolutionary Deep Learning: Principles, Algorithms, Applications, and Open Issues
ACM COMPUTING SURVEYS(2024)
摘要
Over recent years, there has been a rapid development of deep learning (DL) in both industry and academia fields. However, finding the optimal hyperparameters of a DL model often needs high computational cost and human expertise. To mitigate the above issue, evolutionary computation (EC) as a powerful heuristic search approach has shown significant merits in the automated design of DL models, so-called evolutionary deep learning (EDL). This article aims to analyze EDL from the perspective of automated machine learning (AutoML). Specifically, we first illuminate EDL from DL and EC and regard EDL as an optimization problem. According to theDL pipeline, we systematically introduce EDL methods ranging from data preparation, model generation, to model deployment with a new taxonomy (i.e., what and how to evolve/optimize), and focus on the discussions of solution representation and search paradigm in handling the optimization problem by EC. Finally, key applications, open issues, and potentially promising lines of future research are suggested. This survey has reviewed recent developments of EDL and offers insightful guidelines for the development of EDL.
更多查看译文
关键词
Deep learning,evolutionary computation,data preparation,model generation,model deployment
AI 理解论文
溯源树
样例
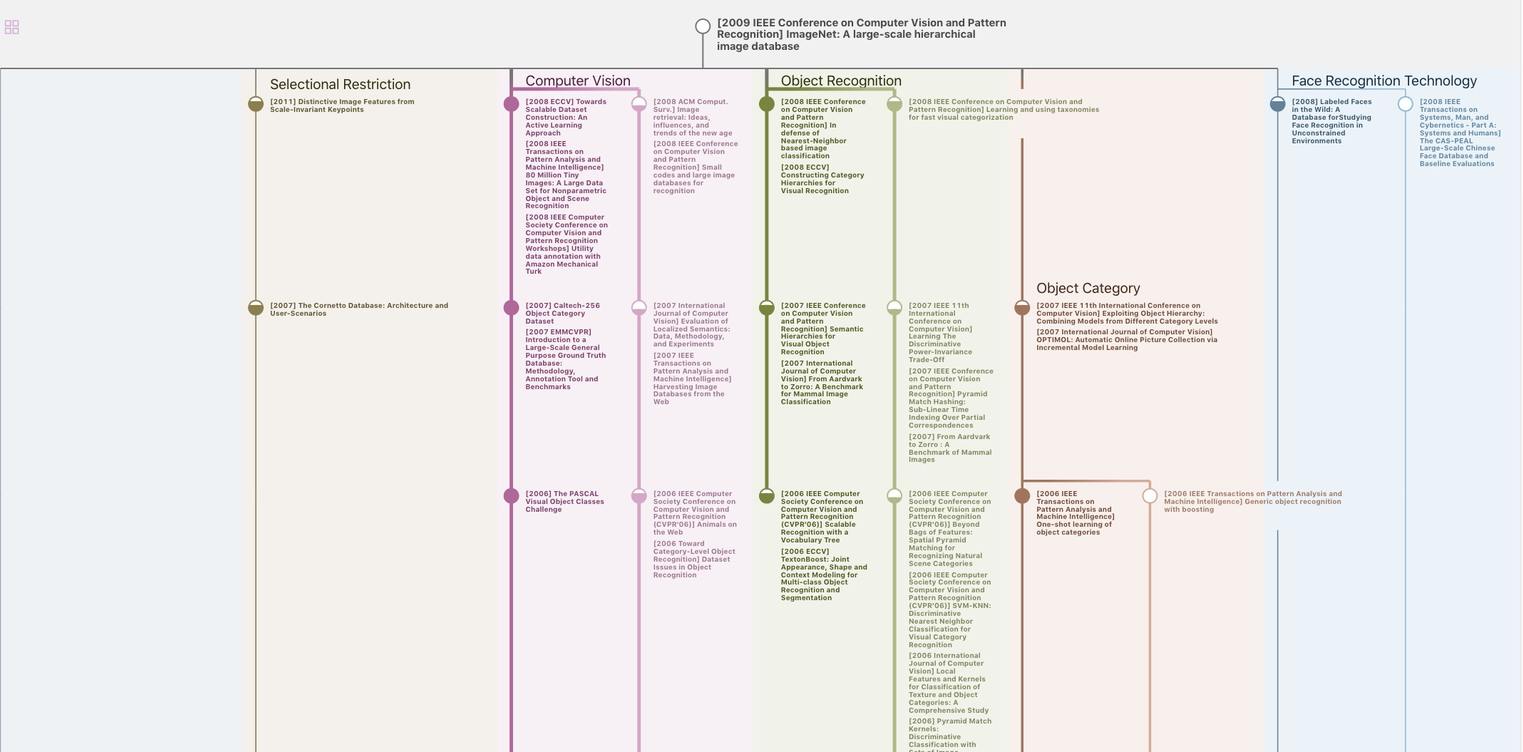
生成溯源树,研究论文发展脉络
Chat Paper
正在生成论文摘要