RIBAC: Towards Robust and Imperceptible Backdoor Attack against Compact DNN.
European Conference on Computer Vision(2022)
摘要
Recently backdoor attack has become an emerging threat to the security of deep neural network (DNN) models. To date, most of the existing studies focus on backdoor attack against the uncompressed model; while the vulnerability of compressed DNNs, which are widely used in the practical applications, is little exploited yet. In this paper, we propose to study and develop Robust and Imperceptible Backdoor Attack against Compact DNN models (RIBAC). By performing systematic analysis and exploration on the important design knobs, we propose a framework that can learn the proper trigger patterns, model parameters and pruning masks in an efficient way. Thereby achieving high trigger stealthiness, high attack success rate and high model efficiency simultaneously. Extensive evaluations across different datasets, including the test against the state-of-the-art defense mechanisms, demonstrate the high robustness, stealthiness and model efficiency of RIBAC. Code is available at https://github.com/huyvnphan/ECCV2022-RIBAC.
更多查看译文
关键词
Backdoor attack,Deep neural networks,Model security
AI 理解论文
溯源树
样例
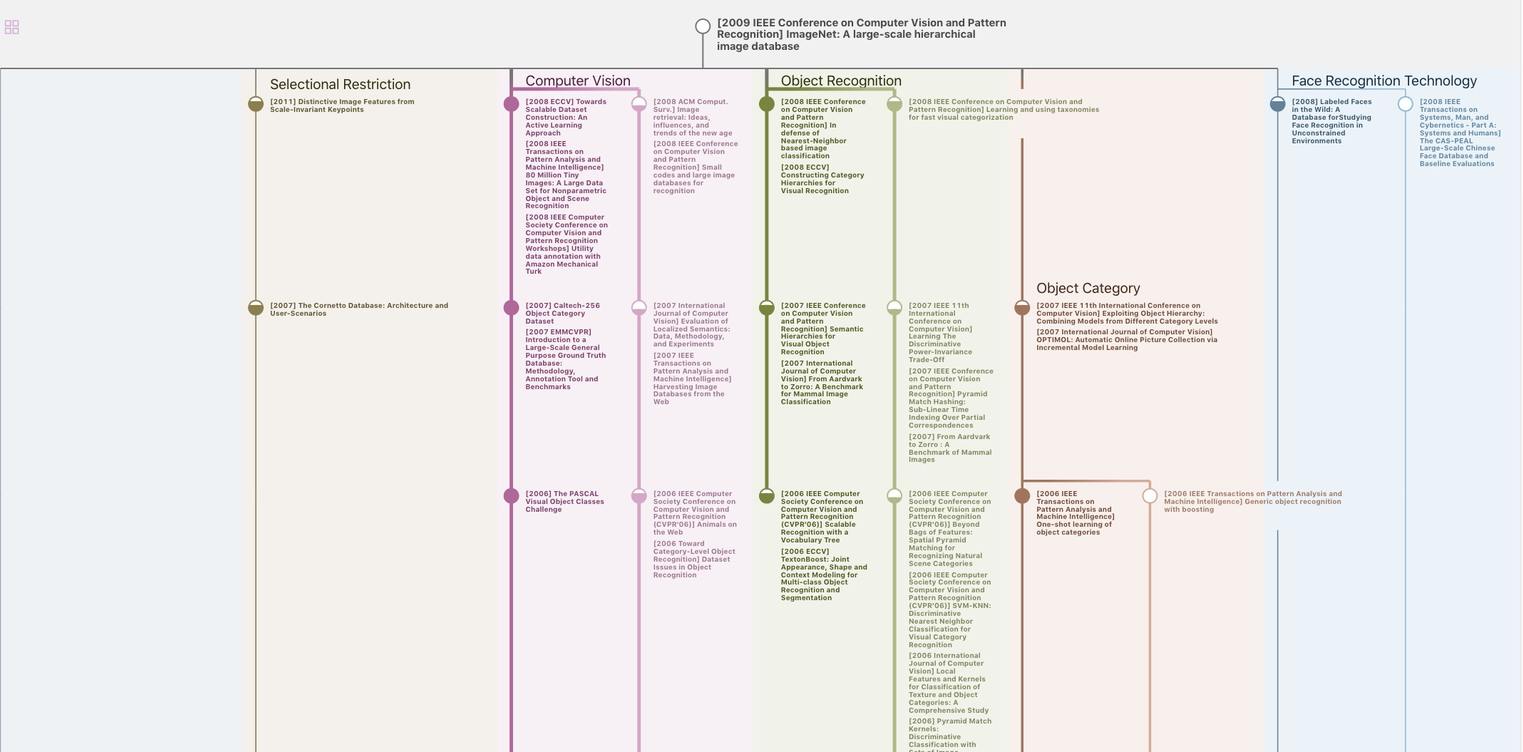
生成溯源树,研究论文发展脉络
Chat Paper
正在生成论文摘要