CLNN-loop: a deep learning model to predict CTCF-mediated chromatin loops in the different cell lines and CTCF-binding sites (CBS) pair types
BIOINFORMATICS(2022)
摘要
Motivation: Three-dimensional (3D) genome organization is of vital importance in gene regulation and disease mechanisms. Previous studies have shown that CTCF-mediated chromatin loops are crucial to studying the 3D structure of cells. Although various experimental techniques have been developed to detect chromatin loops, they have been found to be time-consuming and costly. Nowadays, various sequence-based computational methods can capture significant features of 3D genome organization and help predict chromatin loops. However, these methods have low performance and poor generalization ability in predicting chromatin loops. Results: Here, we propose a novel deep learning model, called CLNN-loop, to predict chromatin loops in different cell lines and CTCF-binding sites (CBS) pair types by fusing multiple sequence-based features. The analysis of a series of examinations based on the datasets in the previous study shows that CLNN-loop has satisfactory performance and is superior to the existing methods in terms of predicting chromatin loops. In addition, we apply the SHAP framework to interpret the predictions of different models, and find that CTCF motif and sequence conservation are important signs of chromatin loops in different cell lines and CBS pair types.
更多查看译文
关键词
chromatin loops,different cell lines,clnn-loop,ctcf-mediated,ctcf-binding
AI 理解论文
溯源树
样例
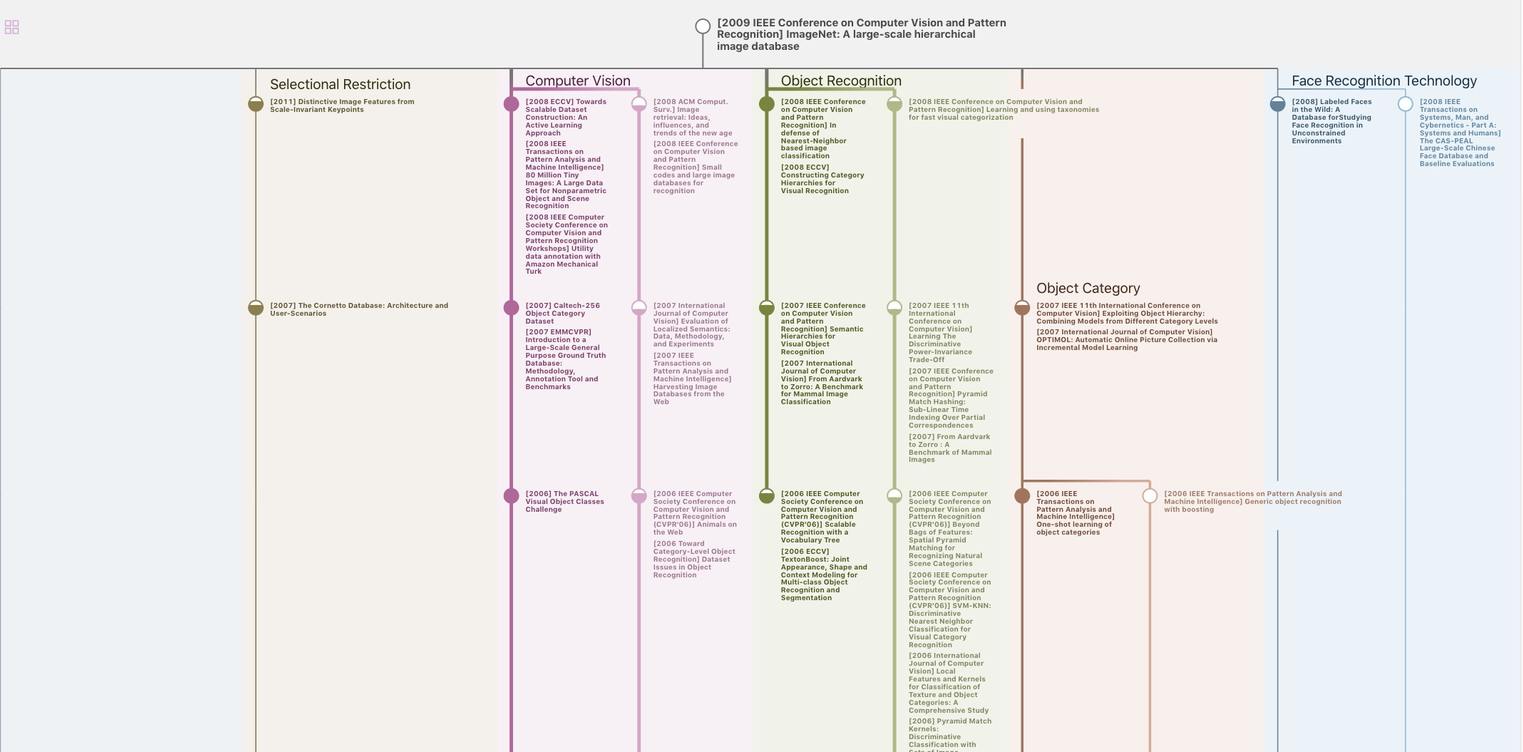
生成溯源树,研究论文发展脉络
Chat Paper
正在生成论文摘要