Face hallucination using multisource references and cross-scale dual residual fusion mechanism
INTERNATIONAL JOURNAL OF INTELLIGENT SYSTEMS(2022)
摘要
There is an increasing interest in enhancing the quality of low-resolution (LR) facial images for various social life applications. Existing methods often use domain-specific prior knowledge, which is effective in improving the face super-resolution model's performance. However, it is challenging to obtain rich and accurate prior information from LR inputs in real-world scenarios, which can limit the robustness and generalization ability of the developed face super-resolution model. In this paper, a multisource reference-based face super-resolution Network, namely MSRNet, is proposed. Without considering the prior knowledge of faces, the network can reconstruct a LR face image with a magnitude factor of 8 under the guidance of multiple reference face images of different identities. By constructing an "appearance-alike" reference data set Face_Ref, the designed MSRNet aims to fully exploit the local and spatially similar high frequency information between the distinct references and the current face. More specifically, to effectively combine the information from multiple references, a cross-scale and cross-space feature fusion mechanism is introduced for external and internal references, and then the enhanced local semantics are finally incorporated into the high-resolution face reconstruction. The robustness of face image super-resolution is increased compared to current correlation approaches, since it not only eliminates the need for face prior knowledge but also avoids performing alignment operations on reference faces with multiple expressions and different poses. Experimental results show that the proposed model is able to produce results for face super-resolution that are satisfying and dependable and outperforms the state-of-the-art methods in terms of visual perceptual quality and quantity evaluation.
更多查看译文
关键词
face super-resolution, face hallucination, multiscale feature fusion, reference-based reconstruction
AI 理解论文
溯源树
样例
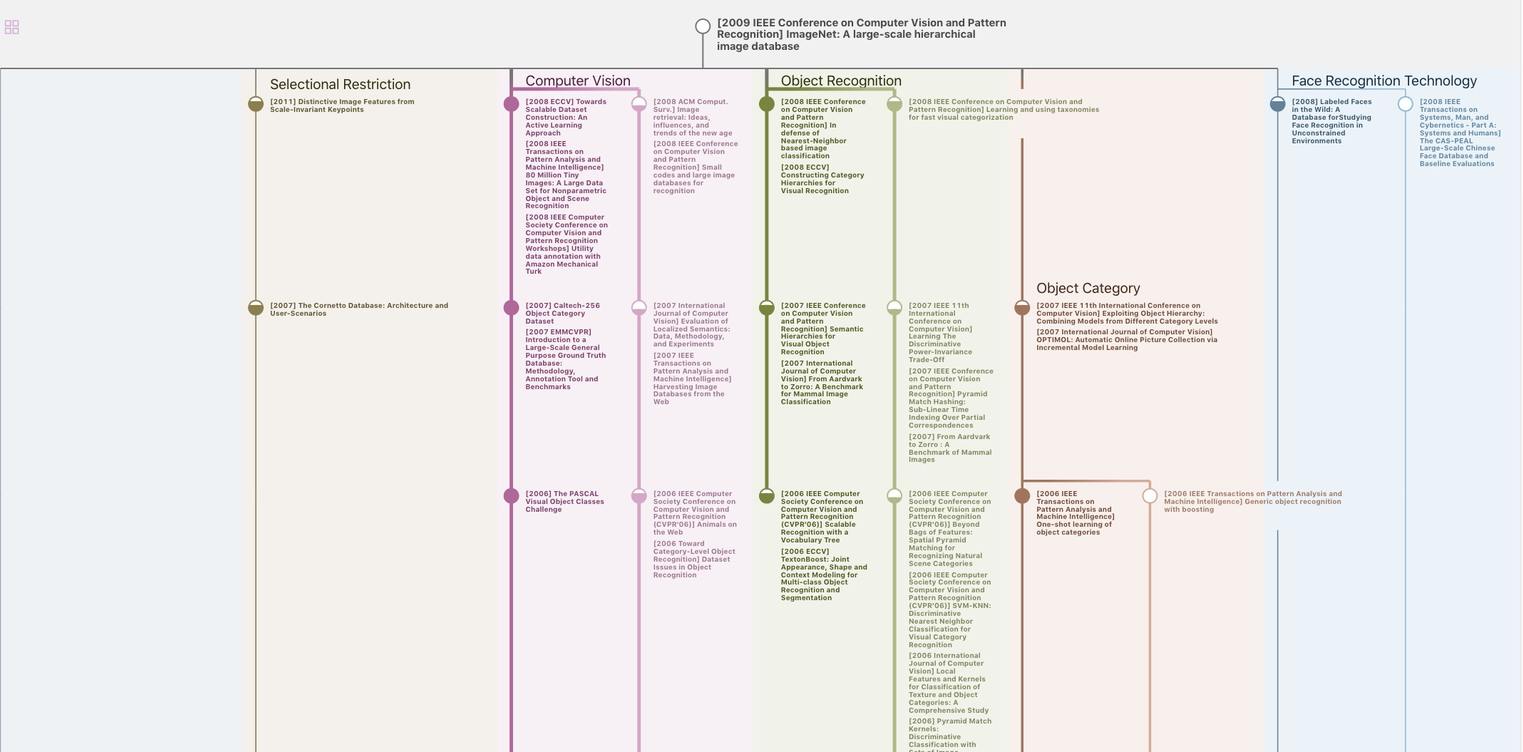
生成溯源树,研究论文发展脉络
Chat Paper
正在生成论文摘要