BARReL: Bottleneck Attention for Adversarial Robustness in Vision-Based Reinforcement Learning
arxiv(2022)
摘要
Robustness to adversarial perturbations has been explored in many areas of computer vision. This robustness is particularly relevant in vision-based reinforcement learning, as the actions of autonomous agents might be safety-critic or impactful in the real world. We investigate the susceptibility of vision-based reinforcement learning agents to gradient-based adversarial attacks and evaluate a potential defense. We observe that Bottleneck Attention Modules (BAM) included in CNN architectures can act as potential tools to increase robustness against adversarial attacks. We show how learned attention maps can be used to recover activations of a convolutional layer by restricting the spatial activations to salient regions. Across a number of RL environments, BAM-enhanced architectures show increased robustness during inference. Finally, we discuss potential future research directions.
更多查看译文
关键词
adversarial robustness,bottleneck attention,vision-based
AI 理解论文
溯源树
样例
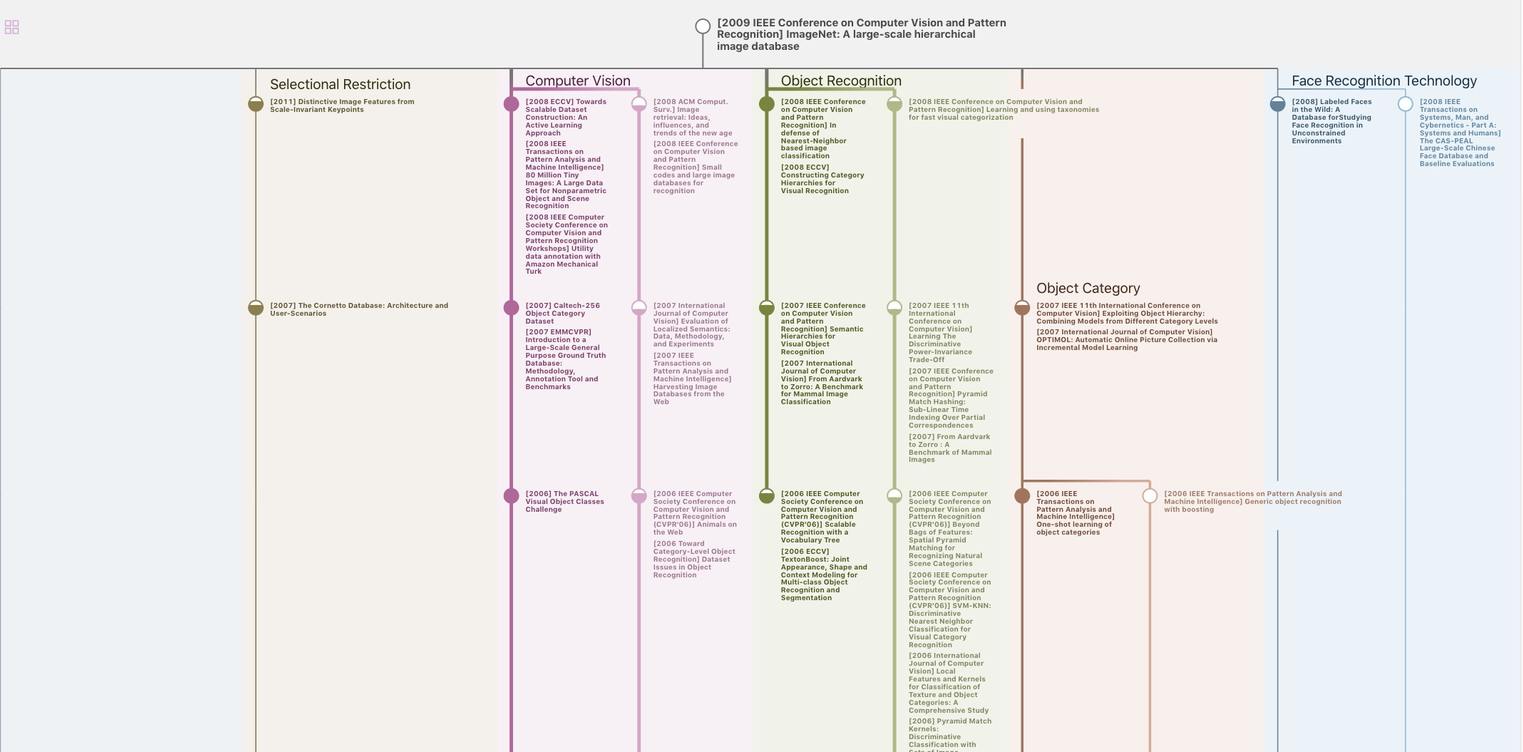
生成溯源树,研究论文发展脉络
Chat Paper
正在生成论文摘要