Dynamic adaptive threshold based learning for noisy annotations robust facial expression recognition
arxiv(2023)
摘要
The real-world facial expression recognition (FER) datasets suffer from noisy annotations due to crowd-sourcing, ambiguity in expressions, the subjectivity of annotators, and inter-class similarity. However, the recent deep networks have a strong capacity to memorize the noisy annotations leading to corrupted feature embedding and poor generalization. Recent works handle the problem by selecting samples with clean labels based on loss values using a fixed threshold for all the classes which may not always be reliable. They also depend upon the noise rate in the data which may not always be available. In this work, we propose a novel FER framework (DNFER) in which samples with clean labels are selected based on a class-specific threshold, computed dynamically in each mini-batch. Specifically, DNFER uses supervised training on selected clean samples and unsupervised consistent training on all the samples. This threshold is independent of noise rate and does not need any clean data, unlike other methods. In addition, to effectively learn from noisy annotated samples, the posterior distributions between weakly-augmented image and strongly-augmented image are aligned using an unsupervised consistency loss. We demonstrate the robustness of DNFER on both synthetic as well as on real noisy annotated FER datasets. In addition, DNFER obtains state-of-the-art performance on popular benchmark datasets, with 90.41% on RAFDB, 57.77 % on SFEW, 89.32% on FERPlus, and 65.22% on AffectNet-7. Our source codes are made publicly available at https://github.com/1980x/DNFER .
更多查看译文
关键词
Facial expression recognition,Noisy annotations,Dynamic training,Consistency,Strong augmentation,Weak-augmentation
AI 理解论文
溯源树
样例
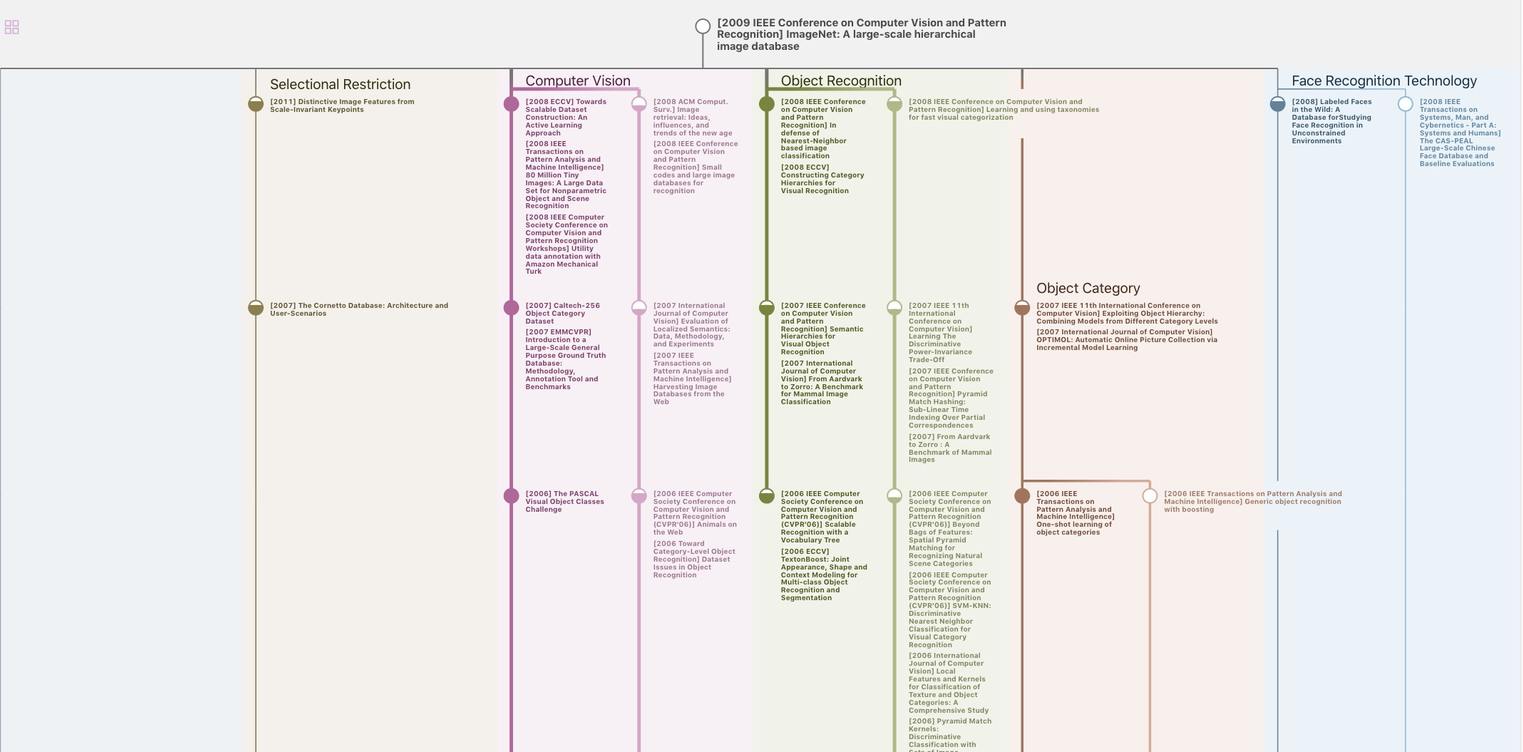
生成溯源树,研究论文发展脉络
Chat Paper
正在生成论文摘要