SPOT: Knowledge-Enhanced Language Representations for Information Extraction
arxiv(2022)
摘要
Knowledge-enhanced pre-trained models for language representation have been shown to be more effective in knowledge base construction tasks (i.e.,~relation extraction) than language models such as BERT. These knowledge-enhanced language models incorporate knowledge into pre-training to generate representations of entities or relationships. However, existing methods typically represent each entity with a separate embedding. As a result, these methods struggle to represent out-of-vocabulary entities and a large amount of parameters, on top of their underlying token models (i.e.,~the transformer), must be used and the number of entities that can be handled is limited in practice due to memory constraints. Moreover, existing models still struggle to represent entities and relationships simultaneously. To address these problems, we propose a new pre-trained model that learns representations of both entities and relationships from token spans and span pairs in the text respectively. By encoding spans efficiently with span modules, our model can represent both entities and their relationships but requires fewer parameters than existing models. We pre-trained our model with the knowledge graph extracted from Wikipedia and test it on a broad range of supervised and unsupervised information extraction tasks. Results show that our model learns better representations for both entities and relationships than baselines, while in supervised settings, fine-tuning our model outperforms RoBERTa consistently and achieves competitive results on information extraction tasks.
更多查看译文
关键词
extraction,information
AI 理解论文
溯源树
样例
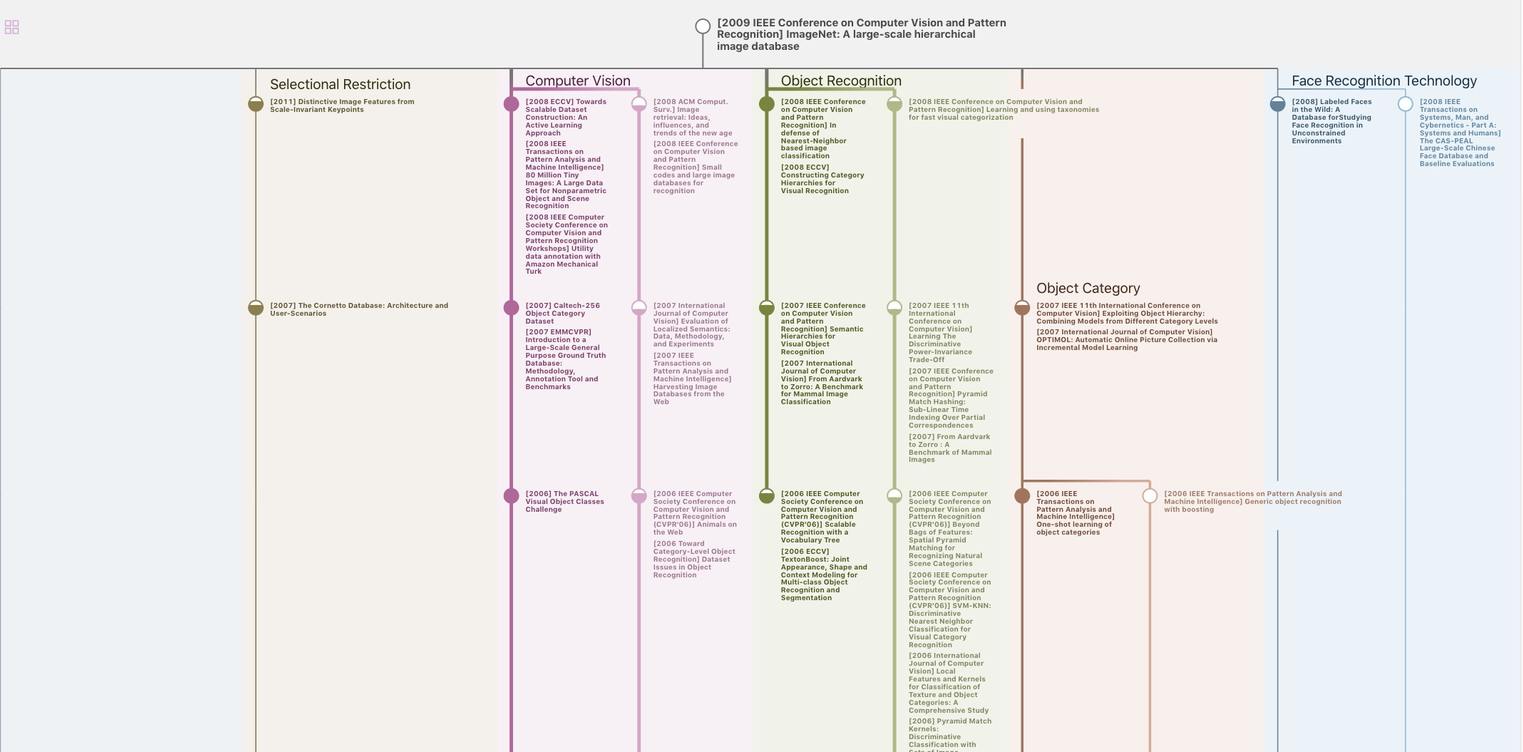
生成溯源树,研究论文发展脉络
Chat Paper
正在生成论文摘要