Boosting lesion annotation via aggregating explicit relations in external medical knowledge graph
Artificial Intelligence in Medicine(2022)
摘要
Predicting a comprehensive set of relevant labels on chest X-ray images faces great challenges towards bridging visual and textual modalities. Despite the success of Graph Convolutional Networks (GCN) on modeling label dependencies using co-occurrence matrix generated from dataset, they still suffer from inherent label imbalance in dataset and ignore the explicit relations among labels presented in external medical knowledge graph (KG). We argue that jointly exploiting both the label co-occurrence matrix in dataset and the label relations in external knowledge graph facilitates multi-label lesion annotation. To model relevant lesion labels more comprehensively, we propose a KG-augmented model via Aggregating Explicit Relations for multi-label lesion annotation, called AER-GCN. The KG-augmented model employs GCN to learn the explicit label relations in external medical KG, and aggregates the explicit relations into statistical graph built from label co-occurrence information. Specially, we present three approaches on modeling the explicit label correlations in external knowledge, and two approaches on incorporating the explicit relations into co-occurrence relations for lesion annotation. We exploit SNOMED CT as the source of external knowledge and evaluate the performance of AER-GCN on the ChestX-ray and IU X-ray datasets. Extensive experiments demonstrate that our model outperforms other state-of-the-art models.
更多查看译文
关键词
Knowledge graph,Lesion annotation,Multi-label Image Classification
AI 理解论文
溯源树
样例
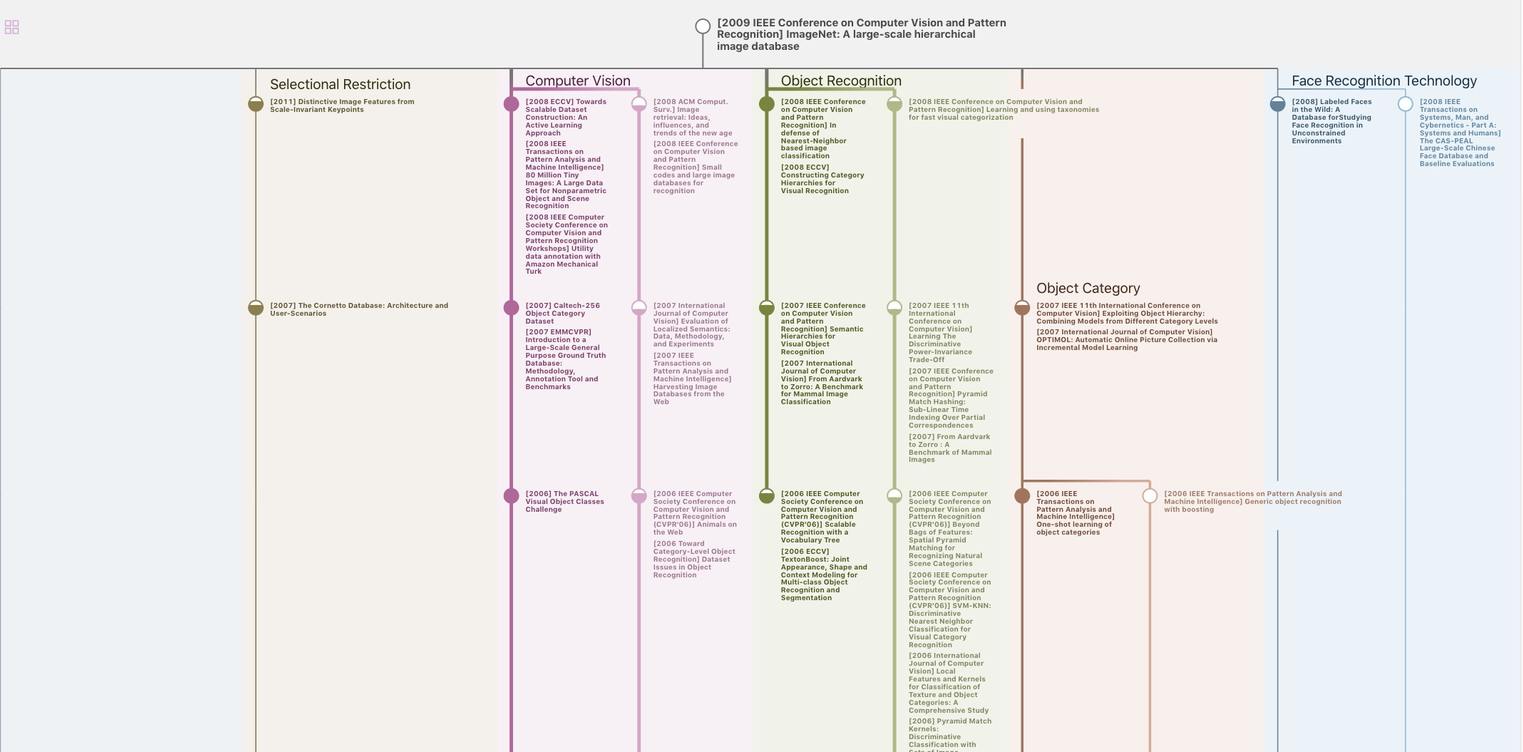
生成溯源树,研究论文发展脉络
Chat Paper
正在生成论文摘要