RG-GCN: A Random Graph Based on Graph Convolution Network for Point Cloud Semantic Segmentation
REMOTE SENSING(2022)
摘要
Point cloud semantic segmentation, a challenging task in 3D data processing, is popular in many realistic applications. Currently, deep learning methods are gradually being applied to point cloud semantic segmentation. However, as it is difficult to manually label point clouds in 3D scenes, it remains difficult to obtain sufficient training samples for the supervised deep learning network. Although an increasing number of excellent methods have been proposed in recent years, few of these have focused on the problem of semantic segmentation with insufficient samples. To address this problem, this paper proposes a random graph based on graph convolution network, referred to as RG-GCN. The proposed network consists of two key components: (1) a random graph module is proposed to perform data augmentation by changing the topology of the built graphs; and (2) a feature extraction module is proposed to obtain local significant features by aggregating point spatial information and multidimensional features. To validate the performance of the RG-GCN, the indoor dataset S3DIS and outdoor dataset Toronto3D are used to validate the proposed network via a series of experiments. The results show that the proposed network achieves excellent performance for point cloud semantic segmentation of the two different datasets.
更多查看译文
关键词
point cloud,semantic segmentation,graph convolution network,data augmentation
AI 理解论文
溯源树
样例
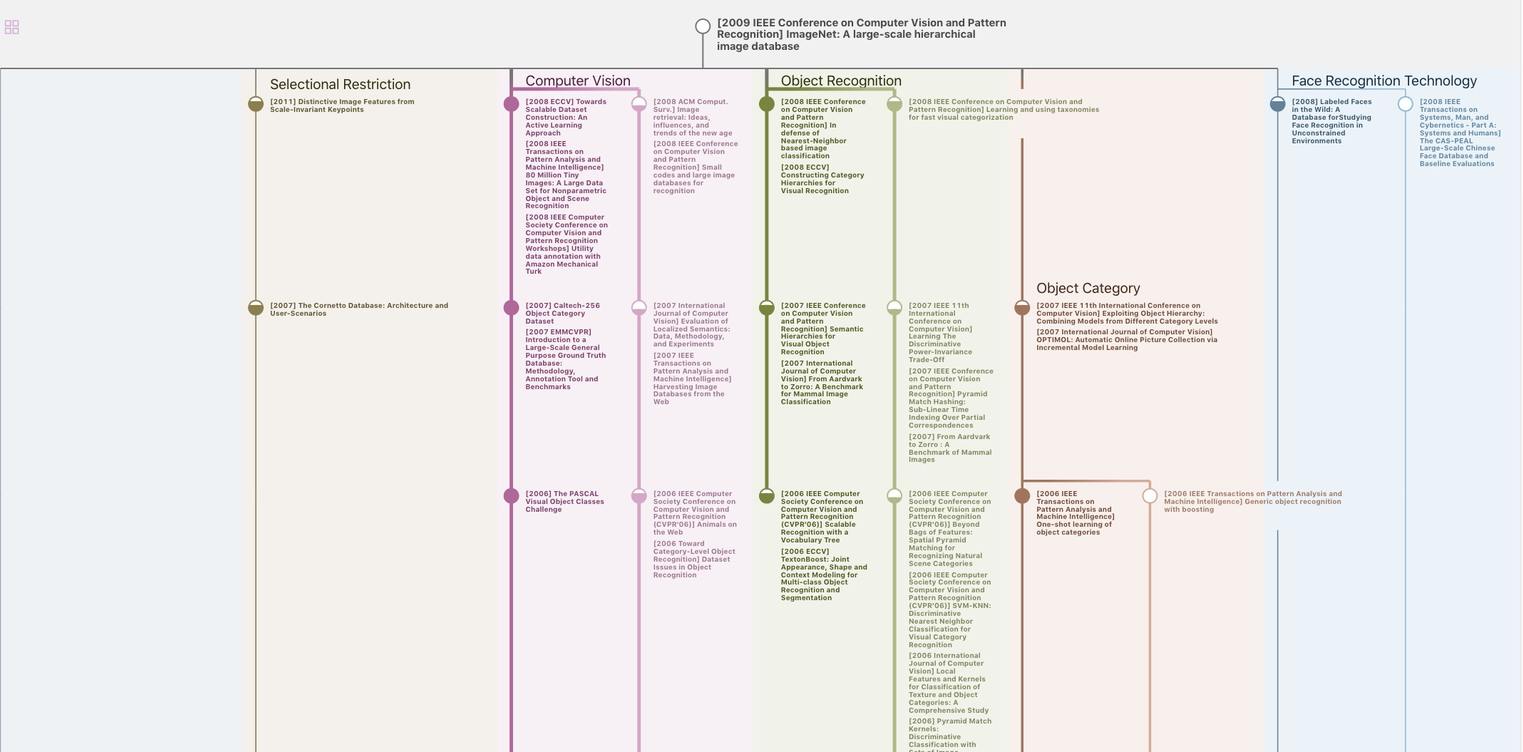
生成溯源树,研究论文发展脉络
Chat Paper
正在生成论文摘要