Matrix Profile XXIV: Scaling Time Series Anomaly Detection to Trillions of Datapoints and Ultra-fast Arriving Data Streams
KDD '22: Proceedings of the 28th ACM SIGKDD Conference on Knowledge Discovery and Data Mining(2022)
摘要
Time series anomaly detection remains one of the most active areas of research in data mining. In spite of the dozens of creative solutions proposed for this problem, recent empirical evidence suggests that time series discords, a relatively simple twenty-year old distance-based technique, remains among the state-of-art techniques. While there are many algorithms for computing the time series discords, they all have limitations. First, they are limited to the batch case, whereas the online case is more actionable. Second, these algorithms exhibit poor scalability beyond tens of thousands of datapoints. In this work we introduce DAMP, a novel algorithm that addresses both these issues. DAMP computes exact left-discords on fast arriving streams, at up to 300,000 Hz using a commodity desktop. This allows us to find time series discords in datasets with trillions of datapoints for the first time. We will demonstrate the utility of our algorithm with the most ambitious set of time series anomaly detection experiments ever conducted.
更多查看译文
AI 理解论文
溯源树
样例
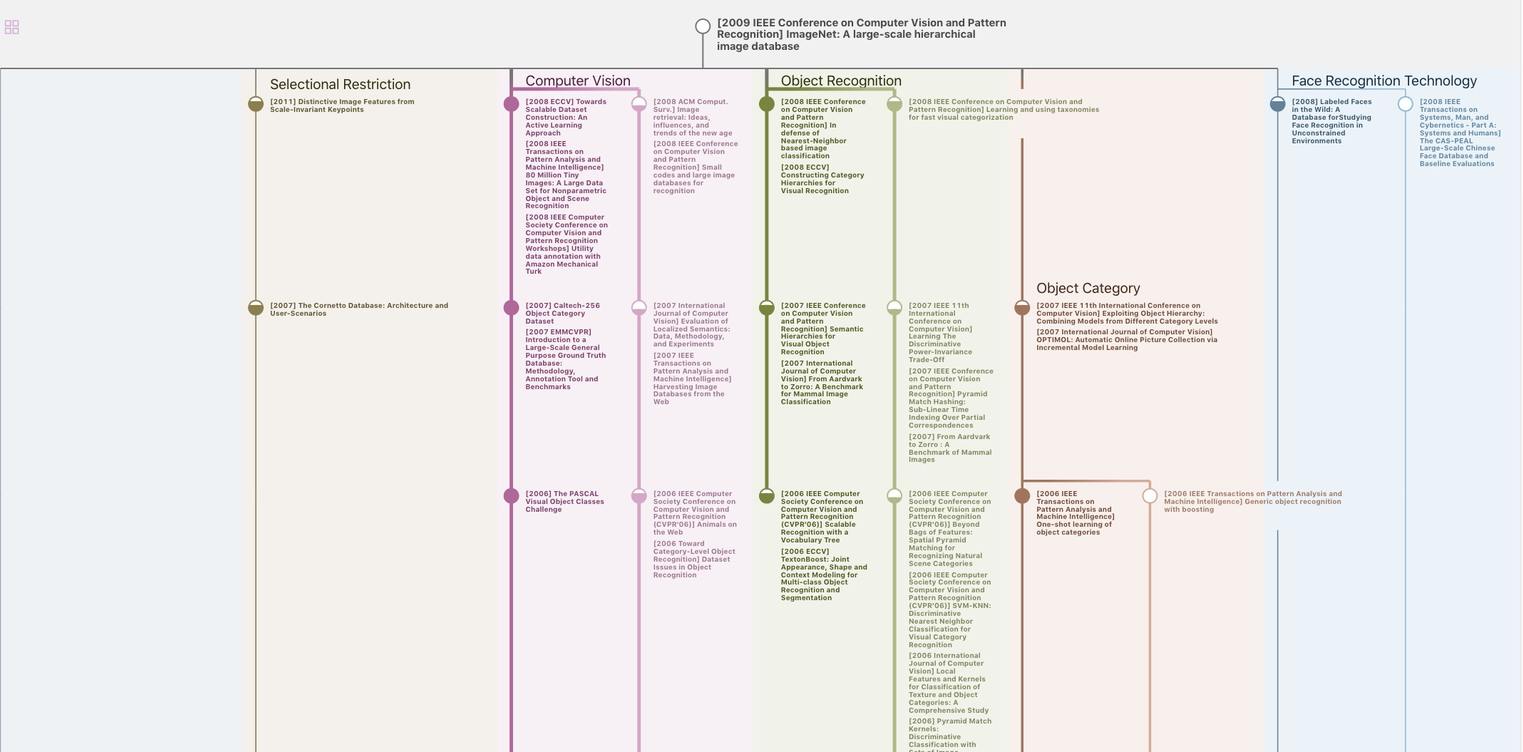
生成溯源树,研究论文发展脉络
Chat Paper
正在生成论文摘要