Multi Armed Bandit vs. A/B Tests in E-commence - Confidence Interval and Hypothesis Test Power Perspectives
KDD '22: Proceedings of the 28th ACM SIGKDD Conference on Knowledge Discovery and Data Mining(2022)
摘要
An emerging dilemma that faces practitioners in large scale online experimentation for e-commence is whether to use Multi-Armed Bandit (MAB) algorithms for testing or traditional A/B testing (A/B). This paper provides a comprehensive comparison between the two, from the perspectives of confidence intervals, hypothesis test powers, and their relationships with traffic split and sample size both theoretically and numerically. We first make comparisons between MAB with A/B tests in terms of conditions under which disjoint confidence intervals occur, and analyze their connection with the traffic split. Then we explore the relationship between the two in terms of sample sizes needed to achieve the required hypothesis test power, and analyze under what conditions MAB could have a higher test power than A/B given the same sample size. Based on the theoretical analysis, we propose two new MAB algorithms that combine the strengths of traditional MAB and A/B together, with higher (or equal) test power and higher (or equal) expected rewards than A/B testing under certain common conditions in e-commerce. Last, we evaluate and compare the performance among the classical MAB algorithms, our newly proposed MAB algorithms, and A/B testing in terms of their accuracy of identifying ground truth winner with practical significance, power rewards trade-off, sample sizes etc. in both simulated datasets and industrial historical datasets. We hope the work can not only facilitate a better understanding of pros and cons of MAB and A/B testing, but also help build the connections between the two and provide possible approaches that can leverage the best from both worlds.
更多查看译文
AI 理解论文
溯源树
样例
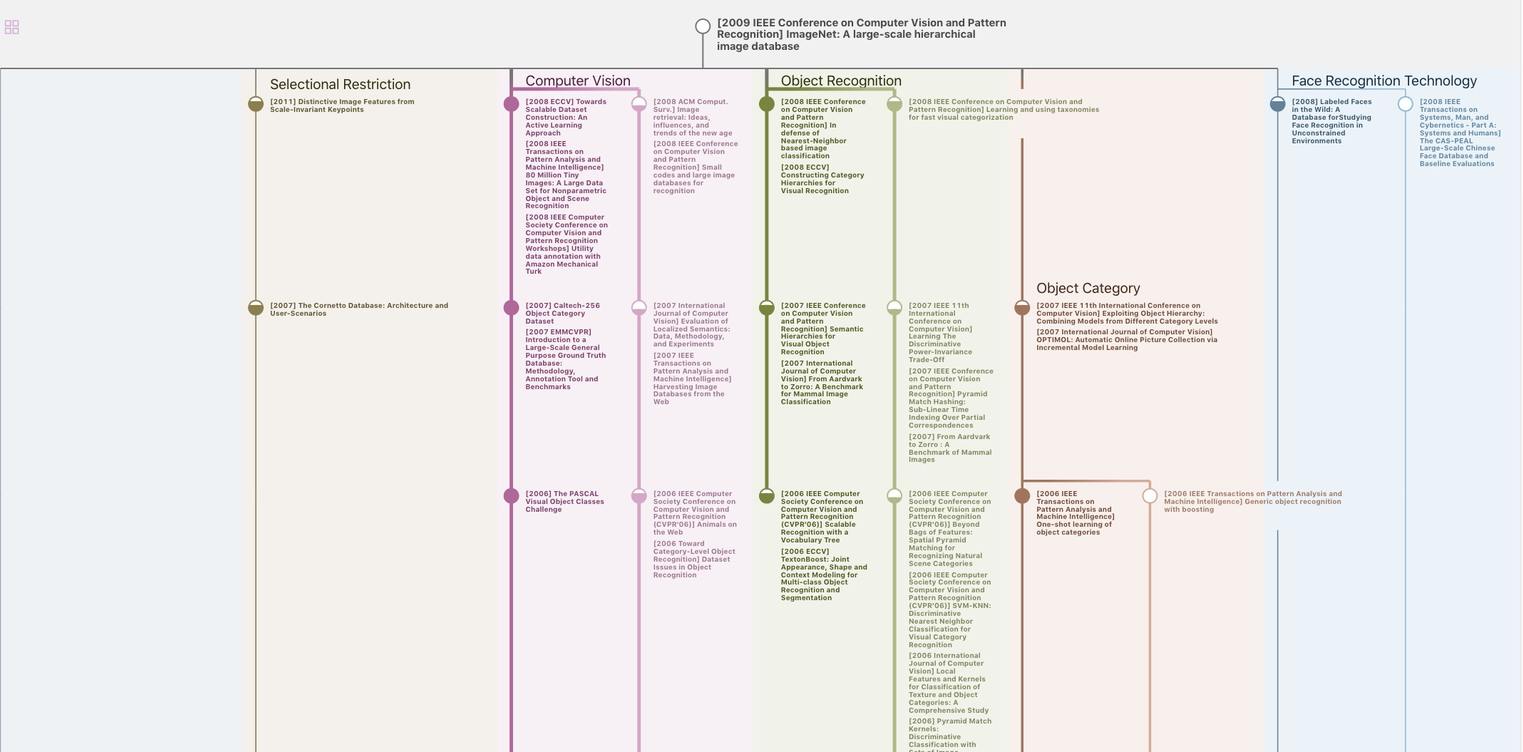
生成溯源树,研究论文发展脉络
Chat Paper
正在生成论文摘要