Augmenting Log-based Anomaly Detection Models to Reduce False Anomalies with Human Feedback
KDD '22: Proceedings of the 28th ACM SIGKDD Conference on Knowledge Discovery and Data Mining(2022)
摘要
With the increasing complexity of modern software systems, it is essential yet hard to detect anomalies and diagnose problems precisely. Existing log-based anomaly detection approaches rely on a few key assumptions on system logs and perform well in some experimental systems. However, real-world industrial systems are often with poor logging quality, in which system logs are noisy and often violate the assumptions of existing approaches. This makes these approaches inefficient. This paper first conducts a comprehensive study on the system logs of three large-scale industrial software systems. Through the study, we identify four typical anti-patterns that affect the detection results the most. Based on these patterns, we propose HiLog, an effective human-in-the-loop log-based anomaly detection approach that integrates human knowledge to augment anomaly detection models. With little human labeling effort, our approach can significantly improve the effectiveness of existing models. Experiment results on three large-scale industrial software systems show that our method improves over 50% precision rate on average.
更多查看译文
AI 理解论文
溯源树
样例
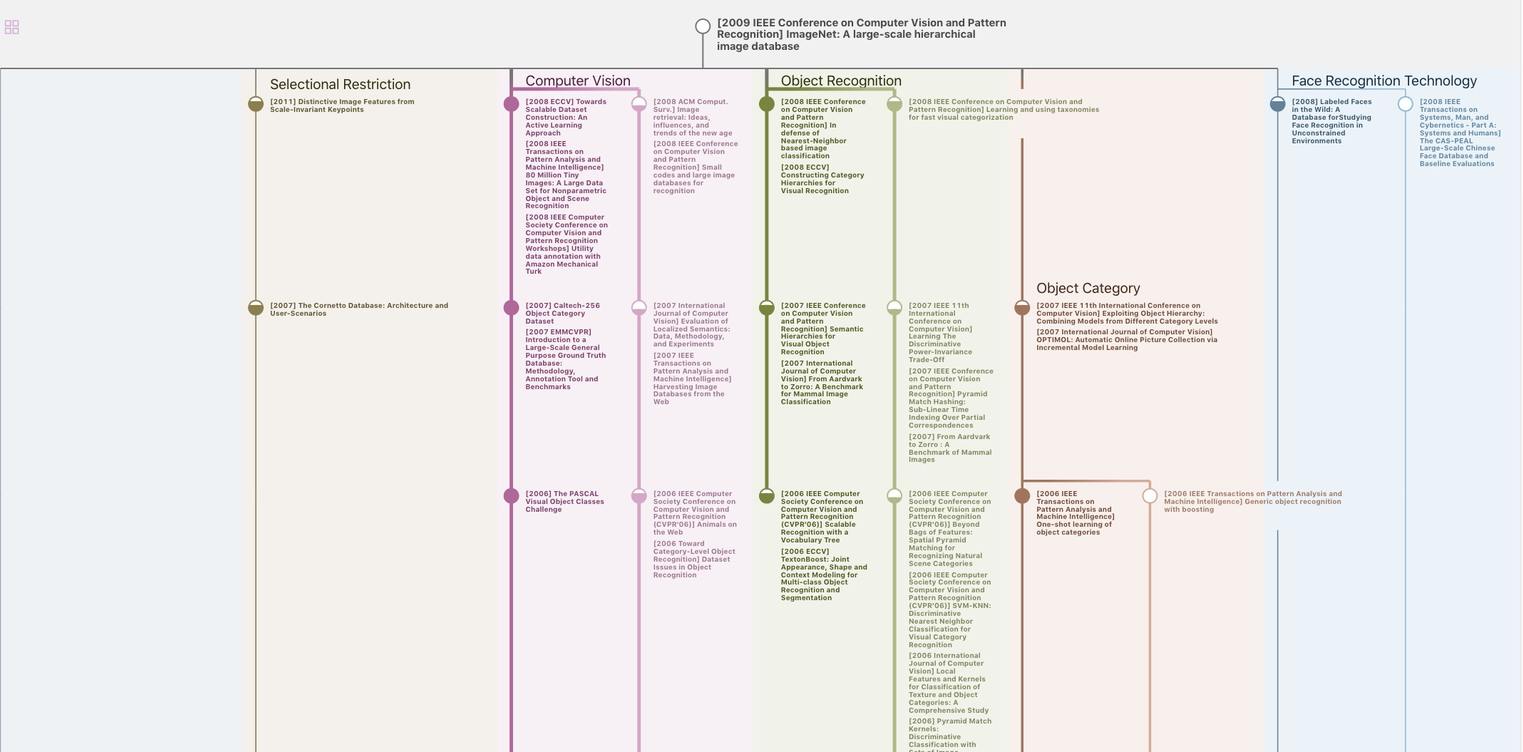
生成溯源树,研究论文发展脉络
Chat Paper
正在生成论文摘要