Multilingual Taxonomic Web Page Classification for Contextual Targeting at Yahoo
KDD '22: Proceedings of the 28th ACM SIGKDD Conference on Knowledge Discovery and Data Mining(2022)
摘要
As we move toward a cookie-less world, the ability to track users' online activities for behavior targeting will be drastically reduced, making contextual targeting an appealing alternative for advertising platforms. Category-based contextual targeting displays ads on web pages that are relevant to advertiser-targeted categories, according to a pre-defined taxonomy. Accurate web page classification is key to the success of this approach. In this paper, we use multilingual Transformer-based transfer learning models to classify web pages in five high-impact languages. We adopt multiple data sampling techniques to increase coverage for rare categories, and modify the loss using class-based re-weighting to smooth the influence of frequent versus rare categories. Offline evaluation shows that these are crucial for improving our classifiers. We leverage knowledge distillation to train accurate models that are lightweight in terms of (i) model size, and (ii) the input text used. Classifying web pages using only text from the URL addresses a unique challenge for contextual targeting in that bid requests come to ad systems as URLs without content, while crawling is time consuming and costly. We launched the proposed models for contextual targeting in the Yahoo DSP, significantly increasing its revenue.
更多查看译文
关键词
contextual targeting,taxonomic,classification,web
AI 理解论文
溯源树
样例
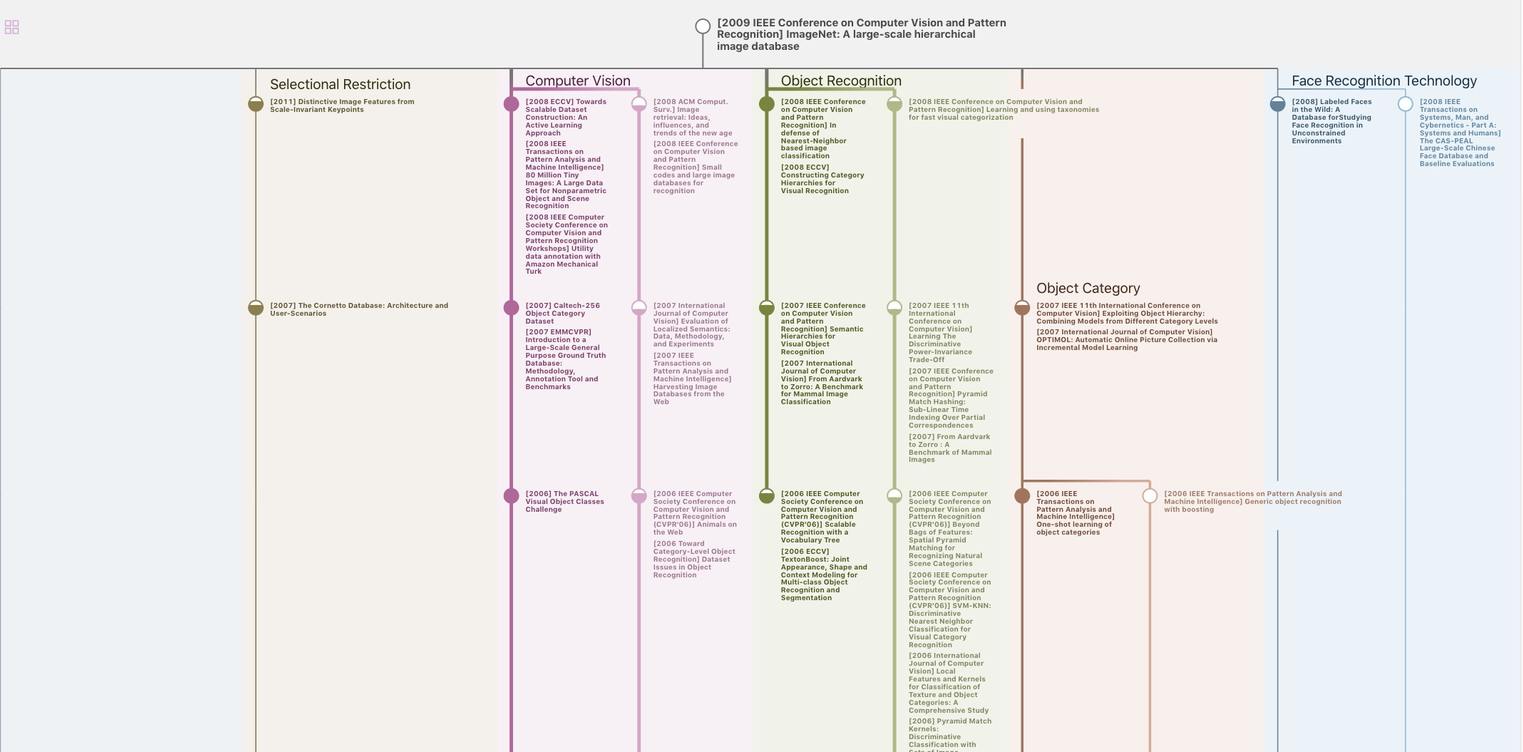
生成溯源树,研究论文发展脉络
Chat Paper
正在生成论文摘要