AdaAX: Explaining Recurrent Neural Networks by Learning Automata with Adaptive States
KDD '22: Proceedings of the 28th ACM SIGKDD Conference on Knowledge Discovery and Data Mining(2022)
摘要
Recurrent neural networks (RNN) are widely used for handling sequence data. However, their black-box nature makes it difficult for users to interpret the decision-making process. We propose a new method to construct deterministic finite automata to explain RNN. In an automaton, states are abstracted from hidden states produced by the RNN, and the transitions represent input symbols. Thus, users can follow the paths of transitions, called patterns, to understand how a prediction is produced. Existing methods for extracting automata partition the hidden state space at the beginning of the extraction, which often leads to solutions that are either inaccurate or too large in size to comprehend. Unlike previous methods, our approach allows the automata states to be formed adaptively during the extraction. Instead of defining patterns on pre-determined clusters, our proposed model, AdaAX, identifies small sets of hidden states determined by patterns with finer granularity in data. Then these small sets are gradually merged to form states, allowing users to trade fidelity for lower complexity. Experiments show that our automata can achieve higher fidelity while being significantly smaller in size than baseline methods on synthetic and complex real datasets.
更多查看译文
AI 理解论文
溯源树
样例
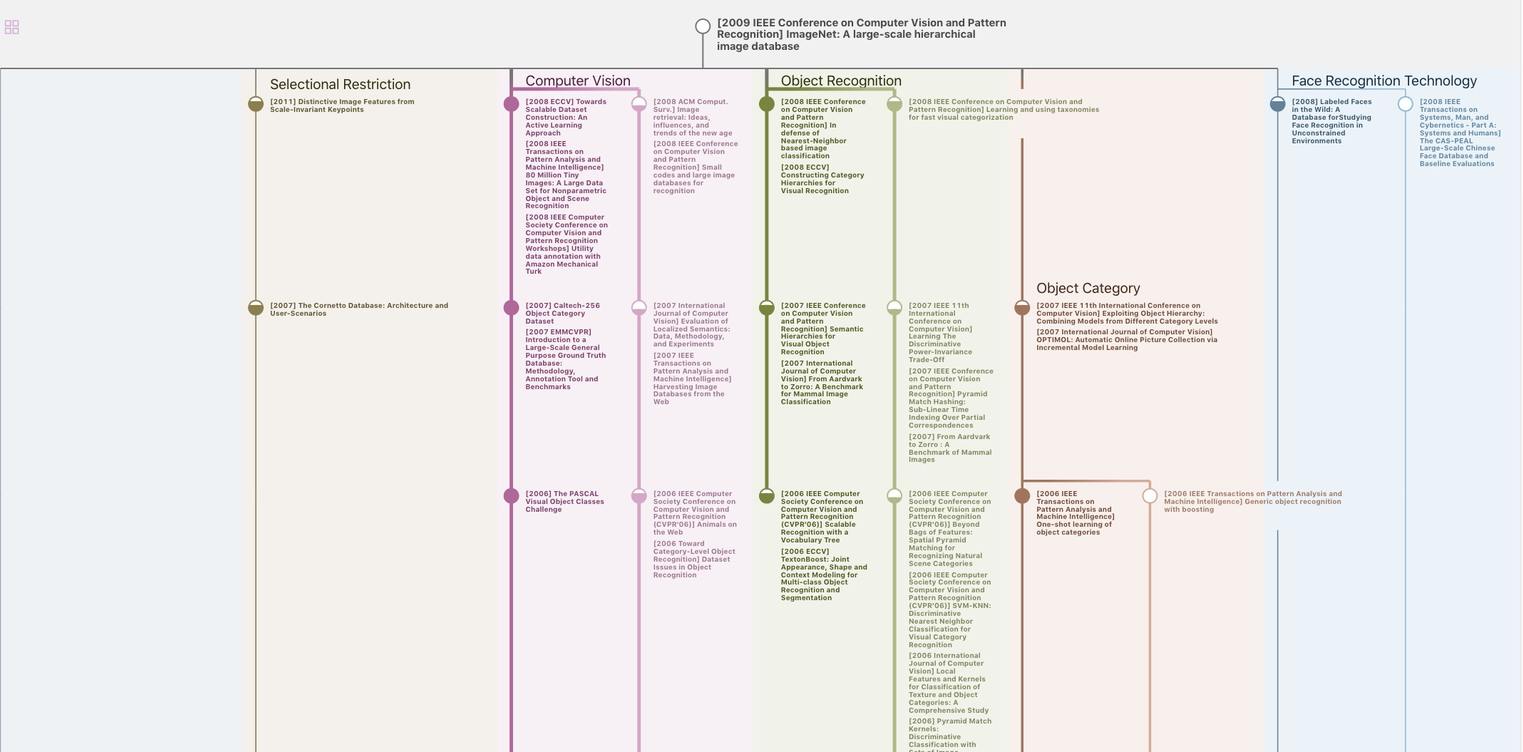
生成溯源树,研究论文发展脉络
Chat Paper
正在生成论文摘要