Causal Discovery on Non-Euclidean Data
KDD '22: Proceedings of the 28th ACM SIGKDD Conference on Knowledge Discovery and Data Mining(2022)
摘要
Researchers recently started developing deep learning models capable of handling non-Euclidean data. However, because of existing framework limitations on model representations and learning algorithms, few have explored causal discovery on non-Euclidean data. This paper is the first attempt to do so. We start by proposing the Non-Euclidean Causal Model (NECM) which describes the causal generative relationship of non-Euclidean data and creates a new tensor data type along with a mapping process for the non-Euclidean causal mechanism. Second, within the NECM, we propose the non-Euclidean Hybrid Learning (NEHL) method, a causal discovery algorithm relying on the concept of the ball covariance recently introduced in the statistics field. Third, we generate two types of non-Euclidean datasets: Functional Data and Symmetric Positive Definite manifold data in conformity with the NECM. Finally, experimental results on the generated data and real-world data demonstrate the effectiveness of the proposed NEHL method.
更多查看译文
关键词
discovery,data,non-euclidean
AI 理解论文
溯源树
样例
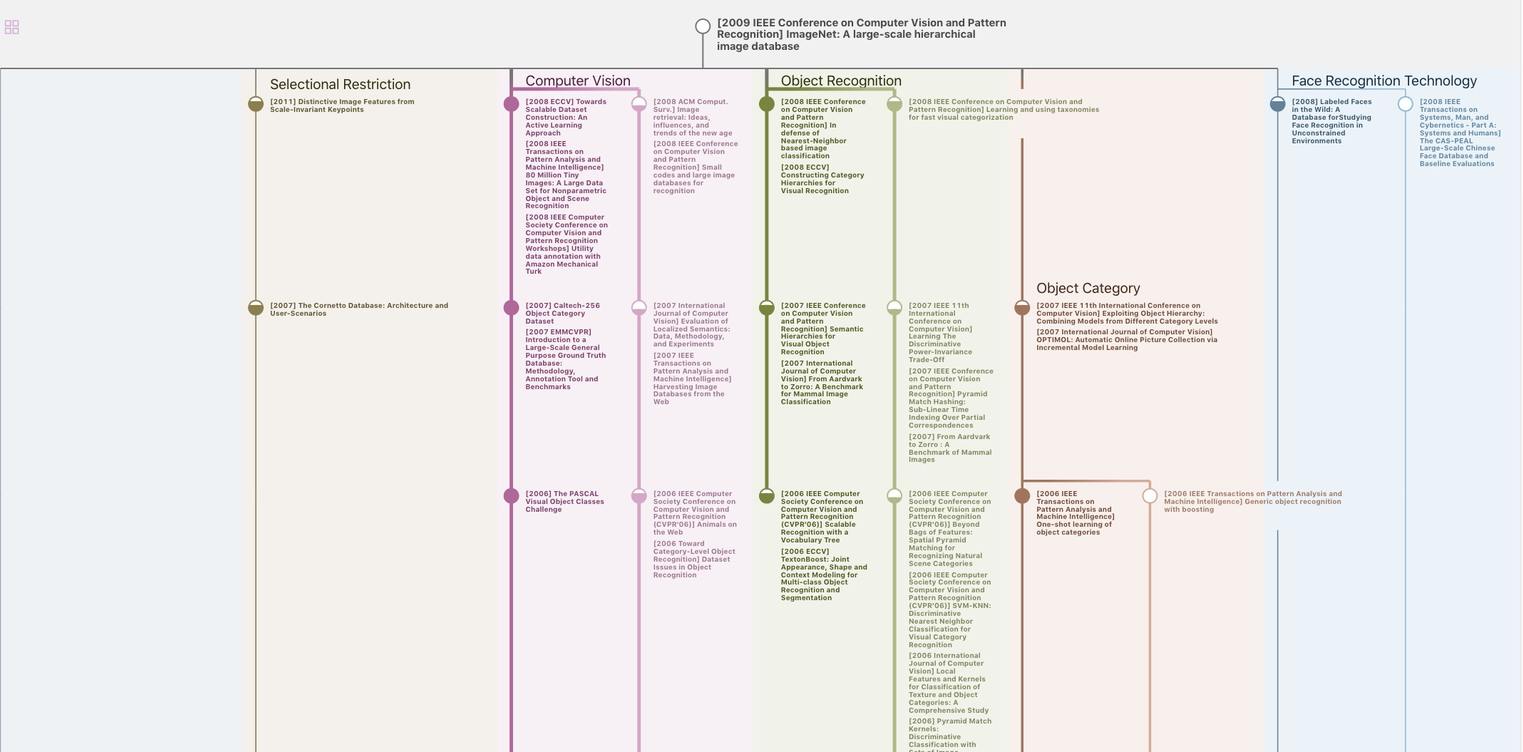
生成溯源树,研究论文发展脉络
Chat Paper
正在生成论文摘要