Adaptive Learning for Weakly Labeled Streams
KDD '22: Proceedings of the 28th ACM SIGKDD Conference on Knowledge Discovery and Data Mining(2022)
摘要
In plenty of real-world applications, data are collected in a streaming fashion, and their accurate labels are hard to obtain. For instance, in the environmental monitoring task, sensors are collecting the data all the time. Still, their labels are scarce because the labeling process requires human effort and can conceal annotation errors. This paper investigates the problem of learning with weakly labeled data streams, in which data are continuously collected, and only a limited subset of streaming data is labeled but potentially with noise. This setting is challenging and of great importance but rarely studied in the literature. When the data are constantly gathered with unknown noise on labels, it is quite challenging to design algorithms to obtain a well-generalized classifier. To address this difficulty, we propose a novel noise transition matrix estimation approach for data streams with scarce noisy labels by online anchor points identification. Based on that, we propose an adaptive learning algorithm for weakly labeled data streams via model reuse and effectively alleviate the negative influence of label noise with unlabeled data. Both theoretical analysis and extensive experiments justify and validate the effectiveness of the proposed approach.
更多查看译文
关键词
weakly labeled streams,learning
AI 理解论文
溯源树
样例
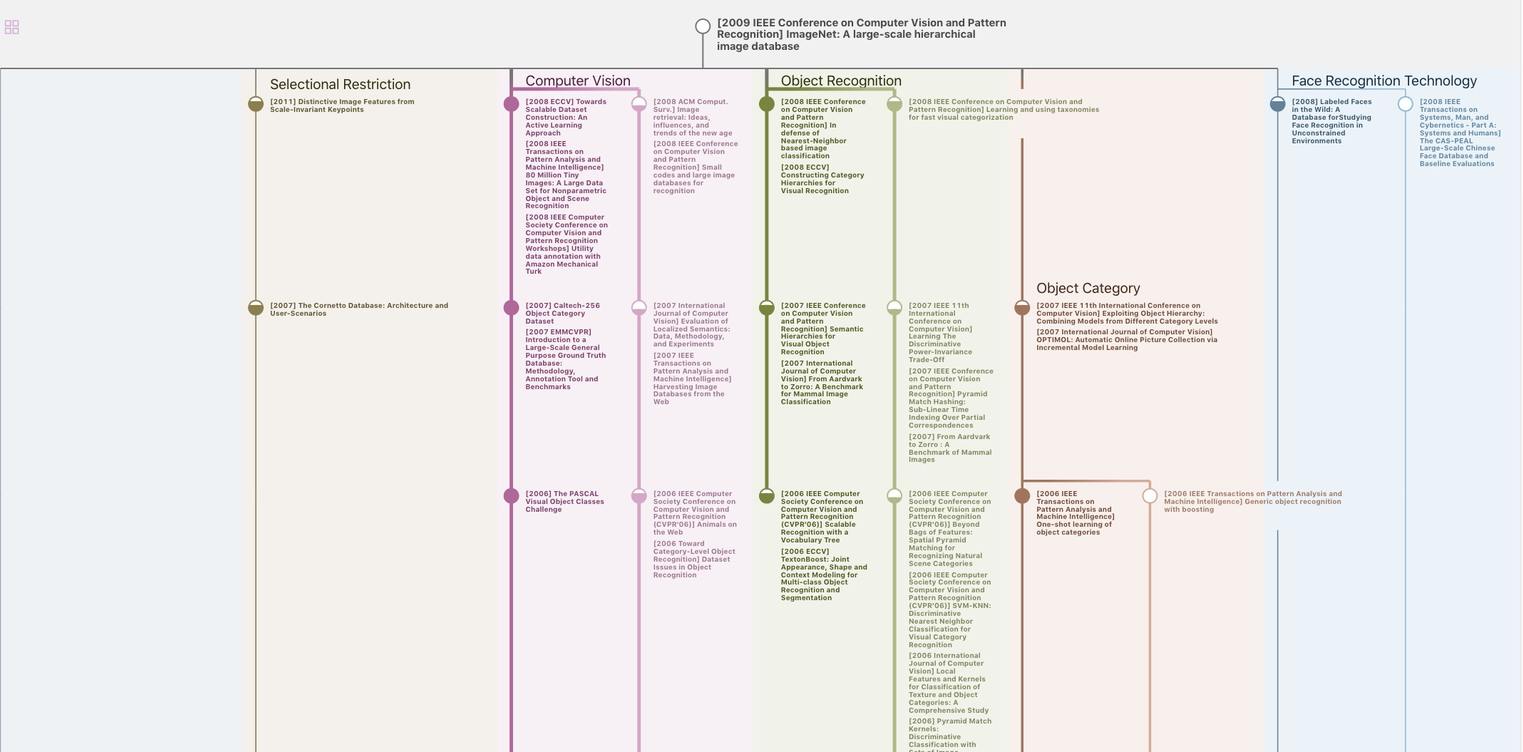
生成溯源树,研究论文发展脉络
Chat Paper
正在生成论文摘要