Federated Select: A Primitive for Communication- and Memory-Efficient Federated Learning
arxiv(2022)
摘要
Federated learning (FL) is a framework for machine learning across heterogeneous client devices in a privacy-preserving fashion. To date, most FL algorithms learn a "global" server model across multiple rounds. At each round, the same server model is broadcast to all participating clients, updated locally, and then aggregated across clients. In this work, we propose a more general procedure in which clients "select" what values are sent to them. Notably, this allows clients to operate on smaller, data-dependent slices. In order to make this practical, we outline a primitive, federated select, which enables client-specific selection in realistic FL systems. We discuss how to use federated select for model training and show that it can lead to drastic reductions in communication and client memory usage, potentially enabling the training of models too large to fit on-device. We also discuss the implications of federated select on privacy and trust, which in turn affect possible system constraints and design. Finally, we discuss open questions concerning model architectures, privacy-preserving technologies, and practical FL systems.
更多查看译文
关键词
learning,select,memory-efficient
AI 理解论文
溯源树
样例
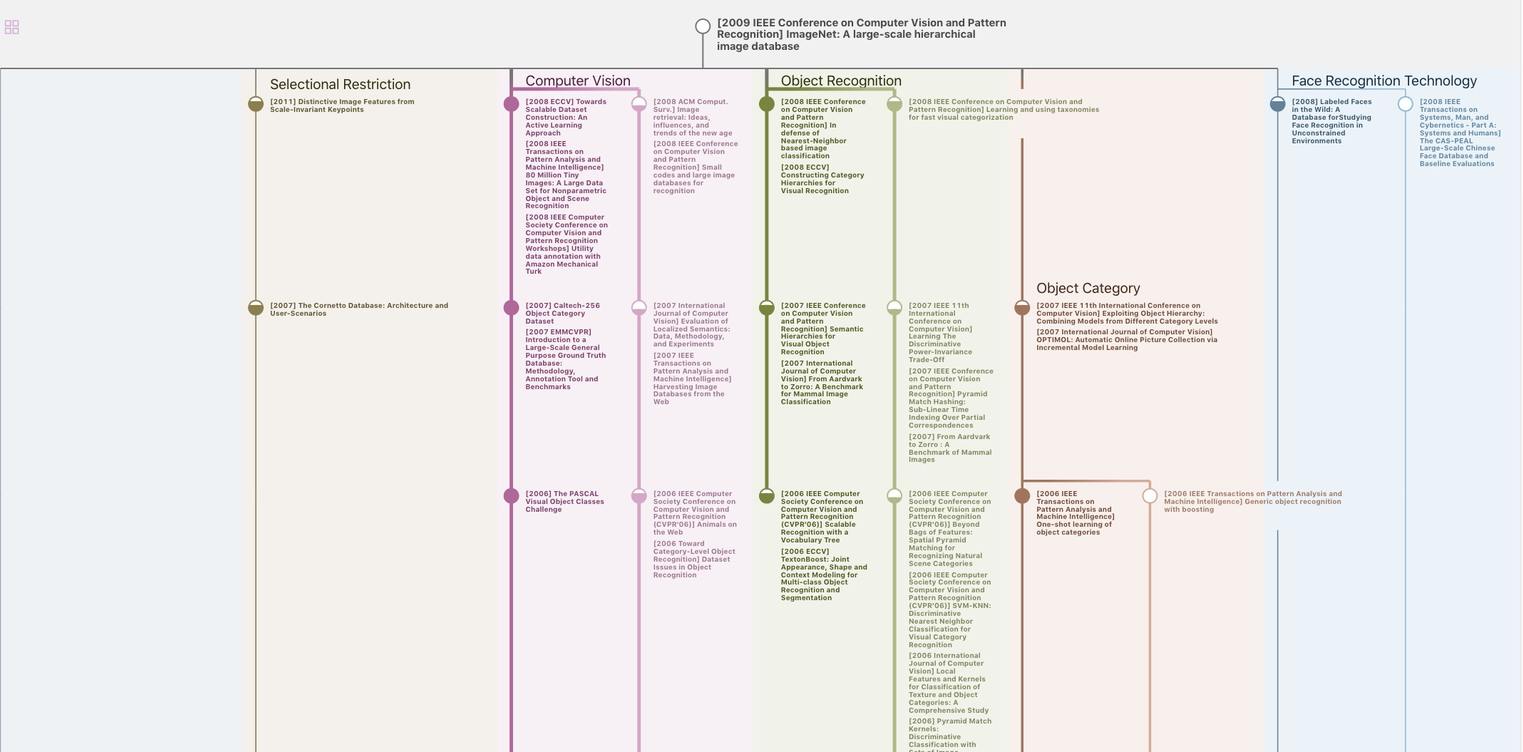
生成溯源树,研究论文发展脉络
Chat Paper
正在生成论文摘要