MaskNet++: Inlier/outlier identification for two point clouds
Computers & Graphics(2022)
摘要
Since point clouds are collected from different views and may be affected by sensors, reflective surfaces or other artifacts, they are often incomplete and may contain a significant amount of outliers. Thus, inlier/outlier identification is necessarily required as the first stage for most geometry processing workflows, for example, point cloud registration, 3D reconstruction, and SLAM. Deep neural networks can automatically and flexibly learn hierarchical features, bringing opportunities and challenges to the algorithms of inlier/outlier identification. MaskNet opened up a new path to estimate inliers, but it is limited to identify inliers/outliers of one point cloud, and it requires a point cloud without outliers as a template. Inspired by that, we propose an improved version, named MaskNet++, to identify inliers/outliers for two point clouds describing the same object or scene without a clean template. The spatial self-attention and channel cross-attention mechanisms are utilized to capture features of each point cloud and exchange the relevant information between two point clouds, respectively. Experiments show that the MaskNet++ can play well in inlier/outlier identification and point cloud denoising. Additionally, impressive progress is made when registration networks are retrofitted with the MaskNet++. Codes are available at https://github.com/zhouruqin/MaskNet2.
更多查看译文
关键词
Inlier/outlier identification,Spatial self-attention,Channel cross-attention,Point cloud registration
AI 理解论文
溯源树
样例
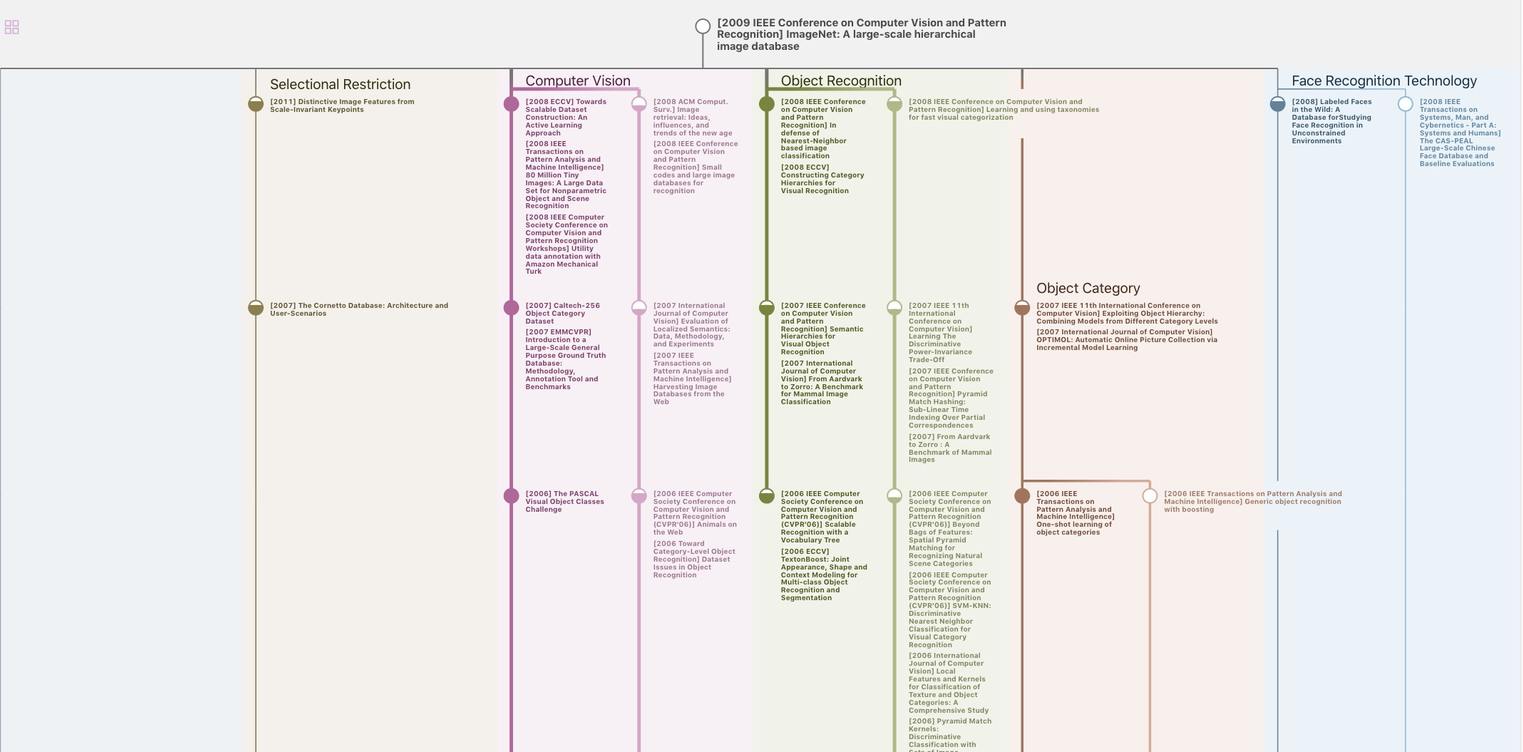
生成溯源树,研究论文发展脉络
Chat Paper
正在生成论文摘要