Derivation of prognostic contextual histopathological features from whole-slide images of tumours via graph deep learning
Nature biomedical engineering(2022)
摘要
Methods of computational pathology applied to the analysis of whole-slide images (WSIs) do not typically consider histopathological features from the tumour microenvironment. Here, we show that a graph deep neural network that considers such contextual features in gigapixel-sized WSIs in a semi-supervised manner can provide interpretable prognostic biomarkers. We designed a neural-network model that leverages attention techniques to learn features of the heterogeneous tumour microenvironment from memory-efficient representations of aggregates of highly correlated image patches. We trained the model with WSIs of kidney, breast, lung and uterine cancers and validated it by predicting the prognosis of 3,950 patients with these four different types of cancer. We also show that the model provides interpretable contextual features of clear cell renal cell carcinoma that allowed for the risk-based retrospective stratification of 1,333 patients. Deep graph neural networks that derive contextual histopathological features from WSIs may aid diagnostic and prognostic tasks.
更多查看译文
关键词
Image processing,Machine learning,Prognostic markers,Renal cell carcinoma,Biomedicine,general,Biomedical Engineering/Biotechnology
AI 理解论文
溯源树
样例
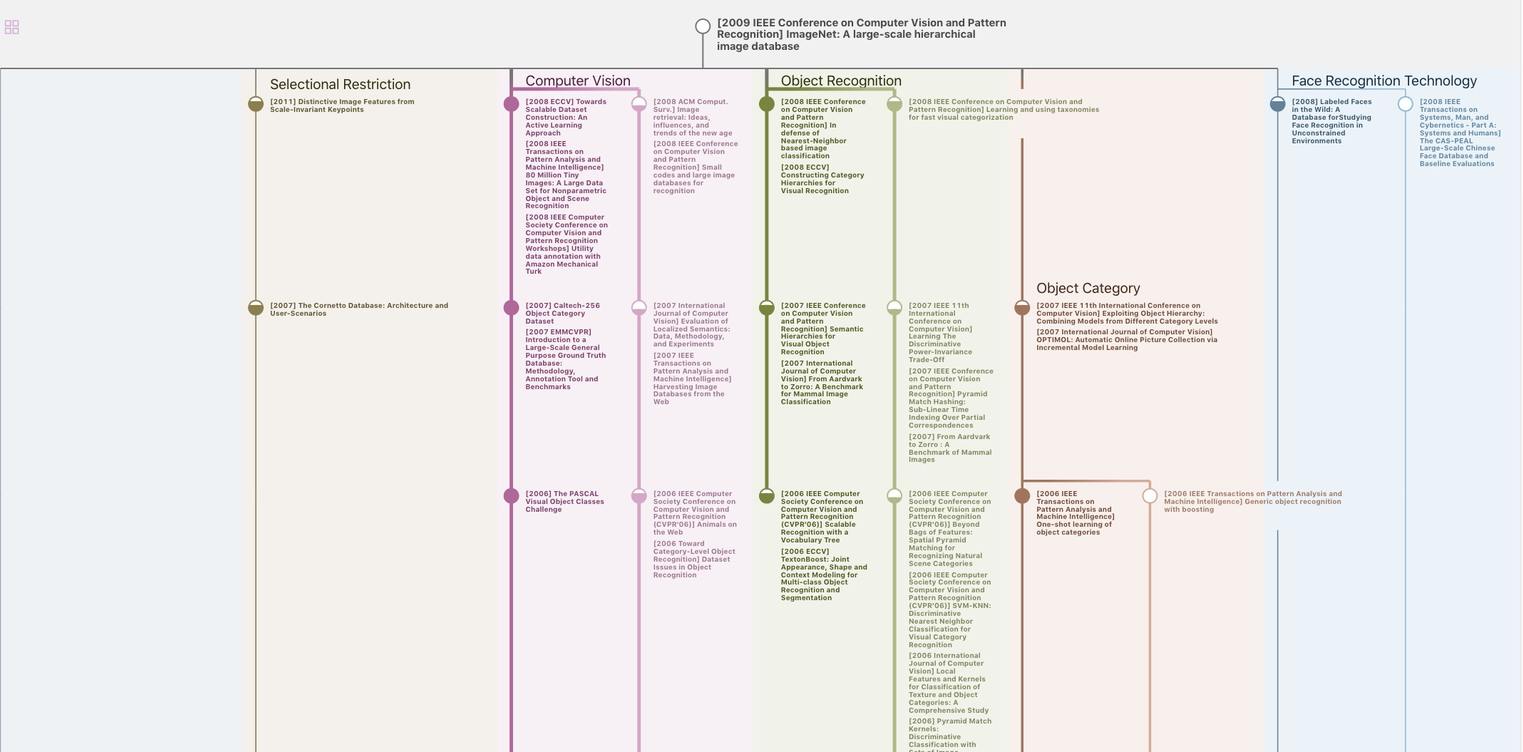
生成溯源树,研究论文发展脉络
Chat Paper
正在生成论文摘要