Using deep learning to distinguish malignant from benign parotid tumors on plain computed tomography images
FRONTIERS IN ONCOLOGY(2022)
摘要
ObjectivesEvaluating the diagnostic efficiency of deep-learning models to distinguish malignant from benign parotid tumors on plain computed tomography (CT) images. Materials and methodsThe CT images of 283 patients with parotid tumors were enrolled and analyzed retrospectively. Of them, 150 were benign and 133 were malignant according to pathology results. A total of 917 regions of interest of parotid tumors were cropped (456 benign and 461 malignant). Three deep-learning networks (ResNet50, VGG16_bn, and DenseNet169) were used for diagnosis (approximately 3:1 for training and testing). The diagnostic efficiencies (accuracy, sensitivity, specificity, and area under the curve [AUC]) of three networks were calculated and compared based on the 917 images. To simulate the process of human diagnosis, a voting model was developed at the end of the networks and the 283 tumors were classified as benign or malignant. Meanwhile, 917 tumor images were classified by two radiologists (A and B) and original CT images were classified by radiologist B. The diagnostic efficiencies of the three deep-learning network models (after voting) and the two radiologists were calculated. ResultsFor the 917 CT images, ResNet50 presented high accuracy and sensitivity for diagnosing malignant parotid tumors; the accuracy, sensitivity, specificity, and AUC were 90.8%, 91.3%, 90.4%, and 0.96, respectively. For the 283 tumors, the accuracy, sensitivity, and specificity of ResNet50 (after voting) were 92.3%, 93.5% and 91.2%, respectively. ConclusionResNet50 presented high sensitivity in distinguishing malignant from benign parotid tumors on plain CT images; this made it a promising auxiliary diagnostic method to screen malignant parotid tumors.
更多查看译文
关键词
deep learning, convolutional neural network, residual neural network, parotid tumor, computed tomography
AI 理解论文
溯源树
样例
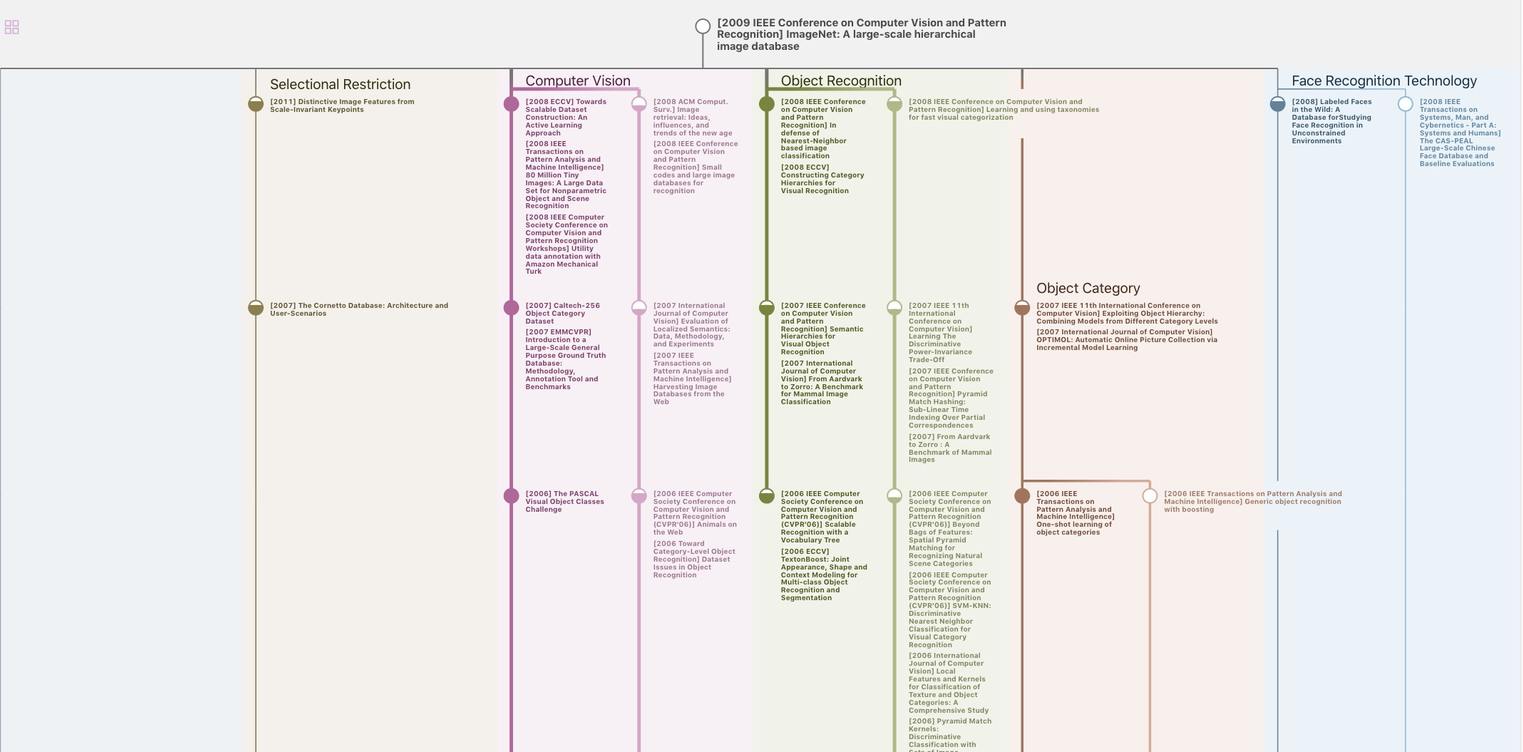
生成溯源树,研究论文发展脉络
Chat Paper
正在生成论文摘要