Autism Spectrum Disorder Classification Based on Interpersonal Neural Synchrony: Can Classification be Improved by Dyadic Neural Biomarkers Using Unsupervised Graph Representation Learning?
International Conference on Medical Image Computing and Computer-Assisted Intervention (MICCAI)(2022)
摘要
Research in machine learning for autism spectrum disorder (ASD) classification bears the promise to improve clinical diagnoses. However, recent studies in clinical imaging have shown the limited generalization of biomarkers across and beyond benchmark datasets. Despite increasing model complexity and sample size in neuroimaging, the classification performance of ASD remains far away from clinical application. This raises the question of how we can overcome these barriers to develop early biomarkers for ASD. One approach might be to rethink how we operationalize the theoretical basis of this disease in machine learning models. Here we introduced unsupervised graph representations that explicitly map the neural mechanisms of a core aspect of ASD, deficits in dyadic social interaction, as assessed by dual brain recordings, termed hyperscanning, and evaluated their predictive performance. The proposed method differs from existing approaches in that it is more suitable to capture social interaction deficits on a neural level and is applicable to young children and infants. First results from functional near-infrared spectroscopy data indicate potential predictive capacities of a task-agnostic, interpretable graph representation. This first effort to leverage interaction-related deficits on neural level to classify ASD may stimulate new approaches and methods to enhance existing models to achieve developmental ASD biomarkers in the future.
更多查看译文
关键词
autism spectrum disorder classification,dyadic neural biomarkers,unsupervised graph representation learning,interpersonal neural synchrony
AI 理解论文
溯源树
样例
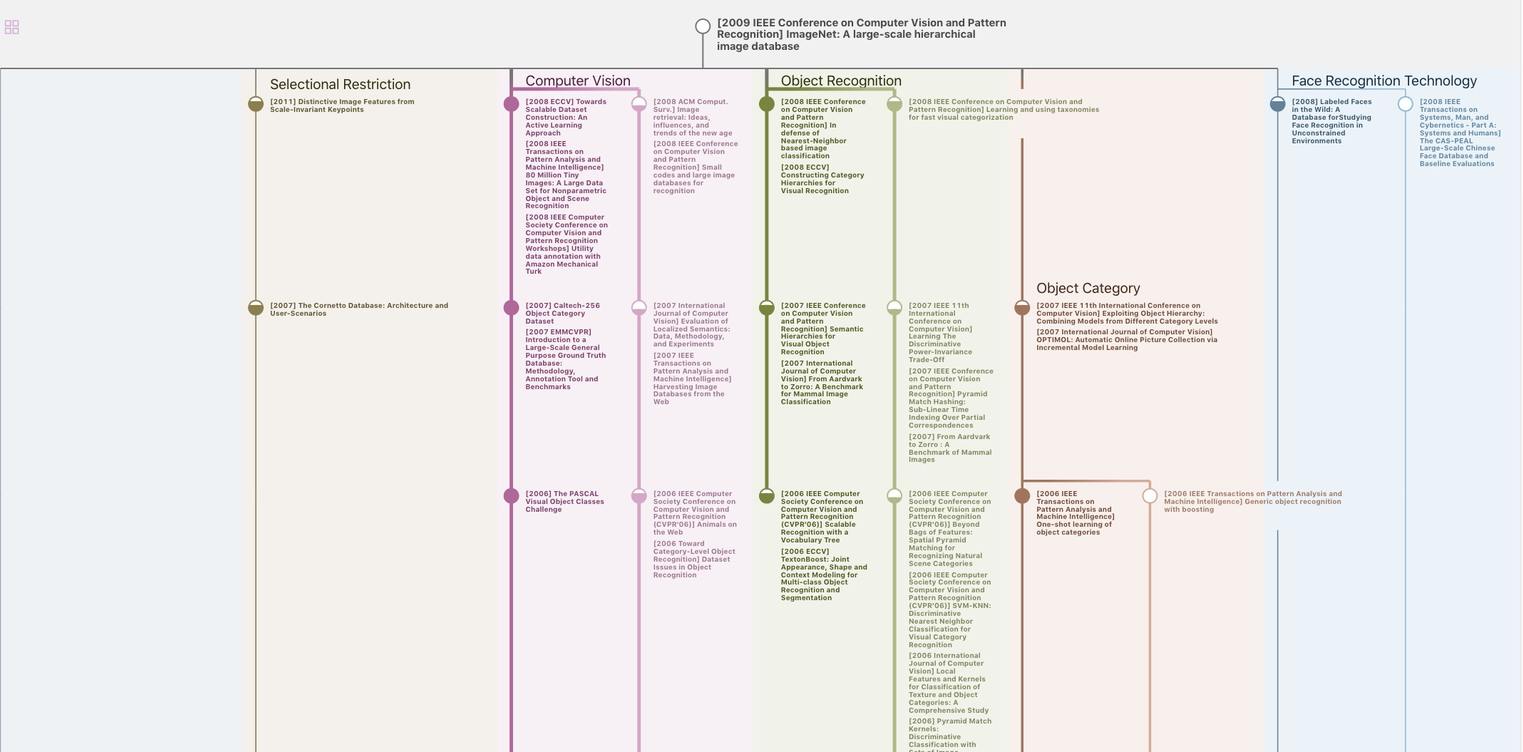
生成溯源树,研究论文发展脉络
Chat Paper
正在生成论文摘要