SensorSCAN: Self-Supervised Learning and Deep Clustering for Fault Diagnosis in Chemical Processes
arxiv(2022)
摘要
Modern industrial facilities generate large volumes of raw sensor data during production process. This data is used to monitor and control the processes and can be analyzed to detect and predict process abnormalities. Typically, the data has to be annotated by experts to be further used in predictive modeling. Most of today's research is focusing on either unsupervised anomaly detection algorithms or supervised methods, that require manually annotated data. The studies are often done using process simulator generated data for a narrow class of events and proposed algorithms are rarely verified on publicly available datasets. In this paper, we propose a novel method SensorSCAN for unsupervised fault detection and diagnosis designed for industrial chemical sensor data. We demonstrate our model performance on two publicly available datasets based on the Tennessee Eastman Process with various fault types. Results show that our method significantly outperforms existing approaches (+0.2-0.3 TPR for a fixed FPR) and detects most of the process faults without the use of expert annotation. In addition, we performed experiments to show that our method is suitable for real-world applications where the number of fault types is not known in advance.
更多查看译文
关键词
Self-supervised learning,Deep clustering,Fault detection,Fault diagnosis,Industrial processes,Chemical processes,Sensor data
AI 理解论文
溯源树
样例
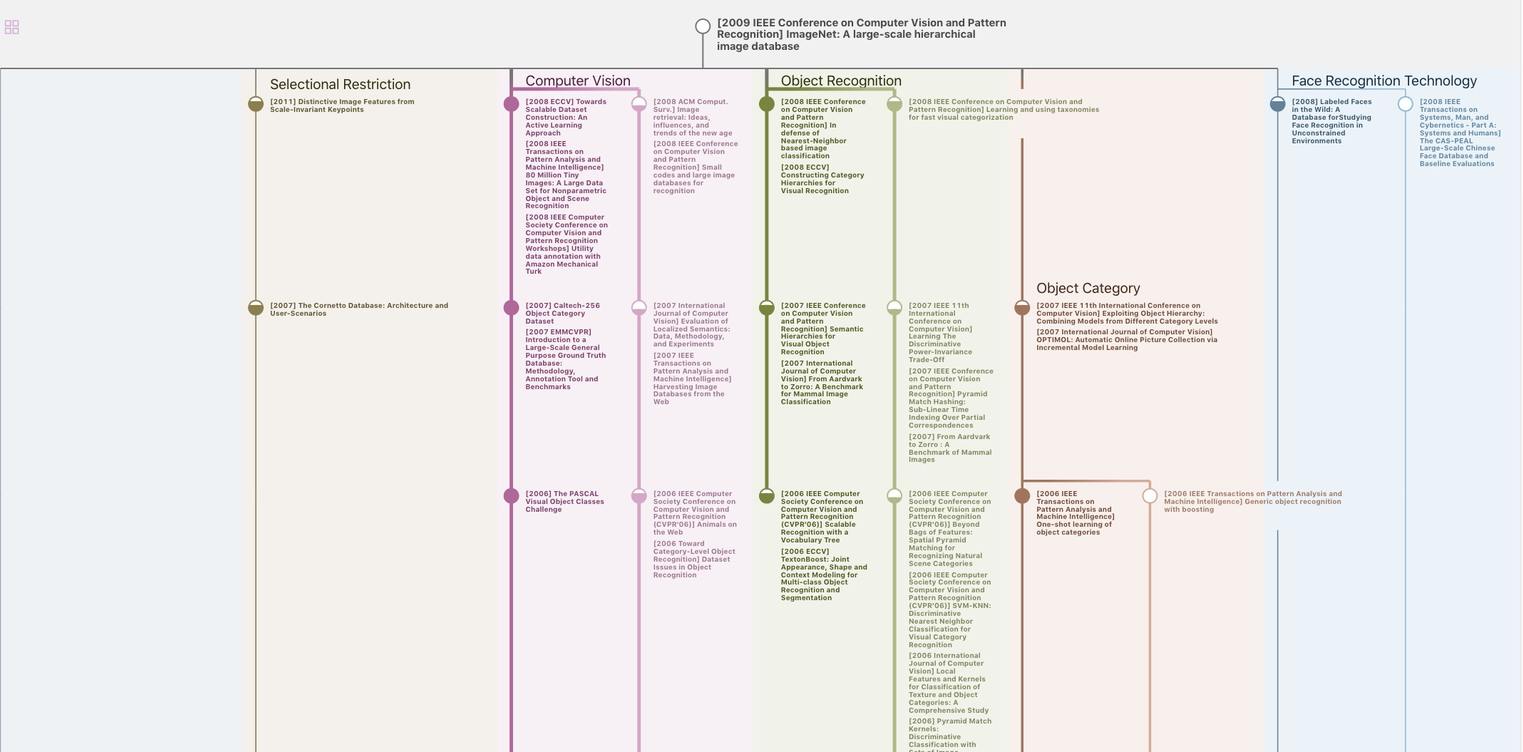
生成溯源树,研究论文发展脉络
Chat Paper
正在生成论文摘要