MvDeCor: Multi-view Dense Correspondence Learning for Fine-Grained 3D Segmentation.
European Conference on Computer Vision(2022)
摘要
We propose to utilize self-supervised techniques in the 2D domain for fine-grained 3D shape segmentation tasks. This is inspired by the observation that view-based surface representations are more effective at modeling high-resolution surface details and texture than their 3D counterparts based on point clouds or voxel occupancy. Specifically, given a 3D shape, we render it from multiple views, and set up a dense correspondence learning task within the contrastive learning framework. As a result, the learned 2D representations are view-invariant and geometrically consistent, leading to better generalization when trained on a limited number of labeled shapes than alternatives based on self-supervision in 2D or 3D alone. Experiments on textured (RenderPeople) and untextured (PartNet) 3D datasets show that our method outperforms state-of-the-art alternatives in fine-grained part segmentation. The improvements over baselines are greater when only a sparse set of views is available for training or when shapes are textured, indicating that MvDeCor benefits from both 2D processing and 3D geometric reasoning. Project page: https://nv-tlabs.github.io/MvDeCor/.
更多查看译文
关键词
3d
AI 理解论文
溯源树
样例
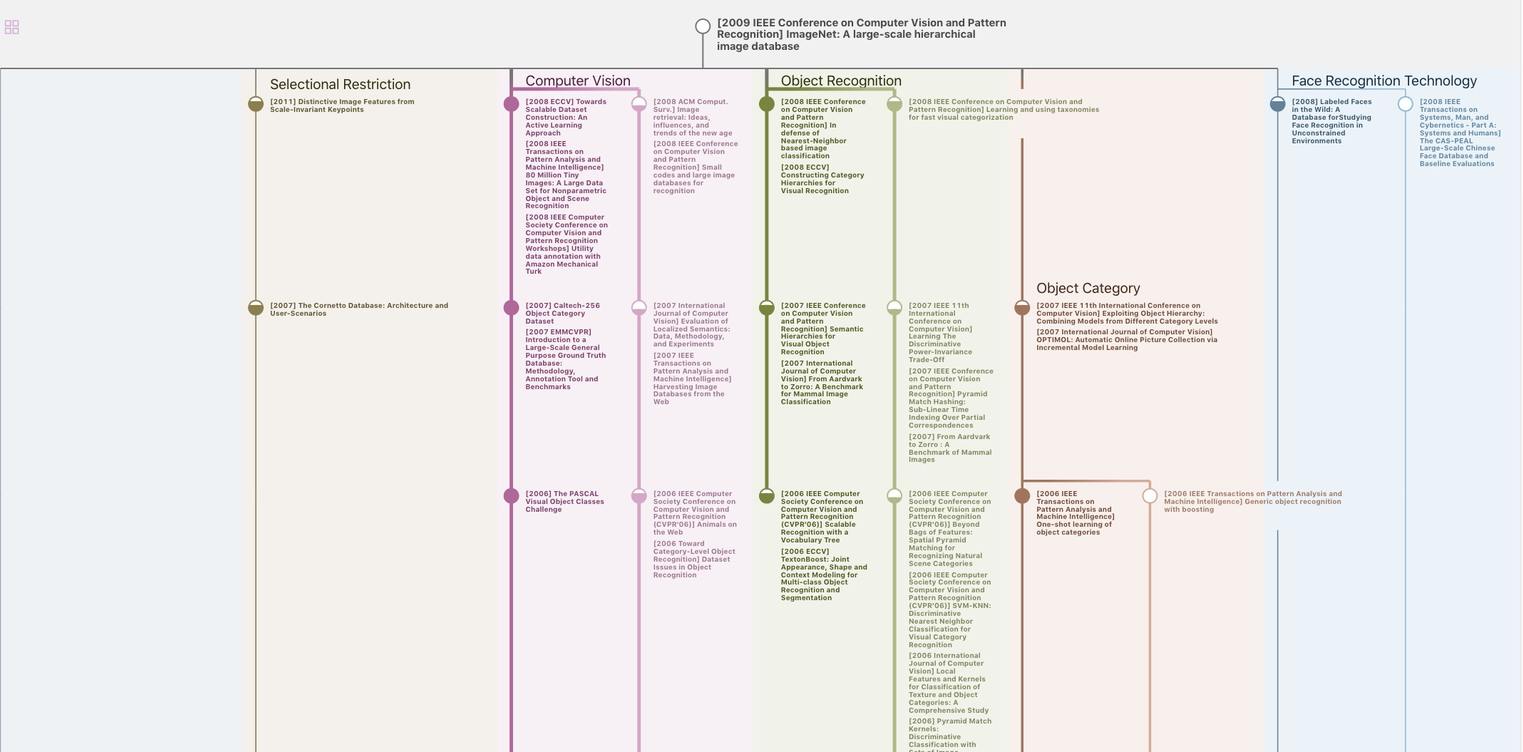
生成溯源树,研究论文发展脉络
Chat Paper
正在生成论文摘要