Influence of Bias and Variance in Selection of Machine Learning Classifiers for Biomedical Applications
Algorithms for Intelligent Systems Smart Data Intelligence(2022)
摘要
Machine learning classifiers play vital role in biomedical signals analysis and disease diagnosis. The selection of proper machine learning model for disease detection is based on the data characteristics. Bias and variance are the important errors which affects the machine learning model performance. Bias and variance are often taken into consideration for error analysis of any model. Unbiasedness is often considered as a positive property of a classifier selection condition but here we present a low variance is at least as significant, as a non-negligible variance introduces the possible solution for over-fitting problem in classifier selection and model training. In machine learning (ML), the performance degradation caused by over-fitting the ML classifiers selection criterion is a common problem but attained a minimum attention in machine learning literature. This paper is aimed to address the problems faced due to over-fitting in machine learning. The effects of over-fitting are often of comparable degree to differences in performance between various learning algorithms and hence cannot be avoided in experimental evaluation. Common performance measuring matrices are dependent on selection of bias/variance and hence results in over-fitting which are unreliable in practice. We discuss various methods to evade the over-fitting in the selection of classifiers and also discuss subsequent bias/variance selection in performance parameter evaluation. While this study focuses on statistical parameter-based ML classifiers selection, the findings are quite general and can be applied for any model selection in practice involving ML classifiers selection in biomedical signal and data applications. The novelty of the suggested method highlights on effect of bias and variance in choosing the ML classifiers, especially for biomedical signals and data classification. There is very limited work carried out to the best of our knowledge toward the ML classifier selection based on bias and variance, and hence, our suggested method ensures better performance in abnormality detection using biomedical signal and data.
更多查看译文
关键词
machine learning classifiers,machine learning,biomedical applications,selection
AI 理解论文
溯源树
样例
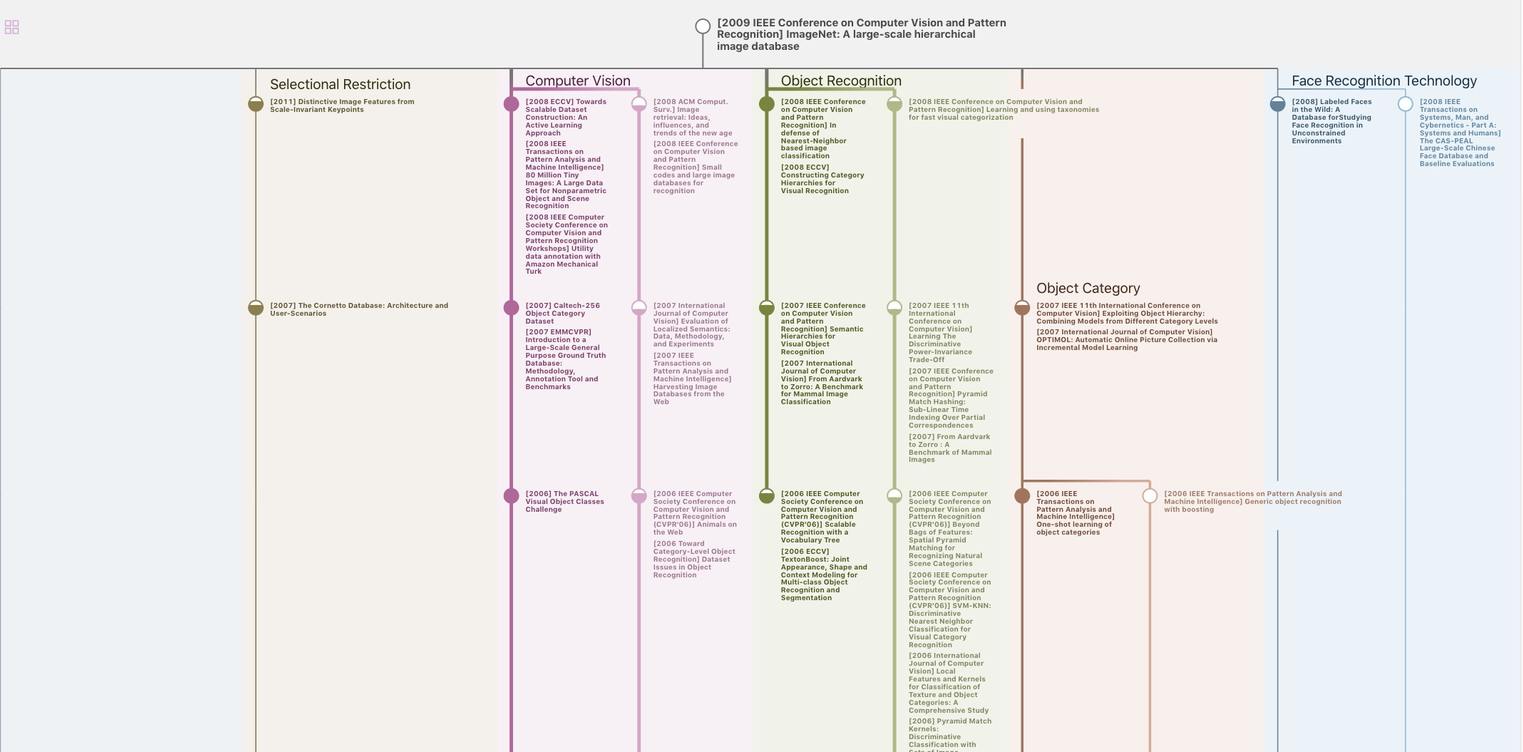
生成溯源树,研究论文发展脉络
Chat Paper
正在生成论文摘要