Kinematic Transfer Learning of Sampling Distributions for Manipulator Motion Planning.
IEEE International Conference on Robotics and Automation(2022)
摘要
Recent research has shown that guiding sampling-based planners with sampling distributions, learned from previous experiences via density estimation, can significantly decrease computation times for motion planning. We propose an algorithm that can estimate the density from the experiences of a robot with different kinematic structure, on the same task. The method allows to generalize collected data from one source manipulator to similarly designed target manipulators, significantly reducing the computation time for new queries for the target manipulator. We evaluate the algorithm in two experiments, including a constrained manipulation task with five different collaborative robots, and show that transferring information can significantly decrease planning time.
更多查看译文
关键词
kinematic structure,constrained manipulation task,collaborative robots,information transfer,kinematic transfer learning,sampling distributions,manipulator motion planning,sampling-based planners,density estimation
AI 理解论文
溯源树
样例
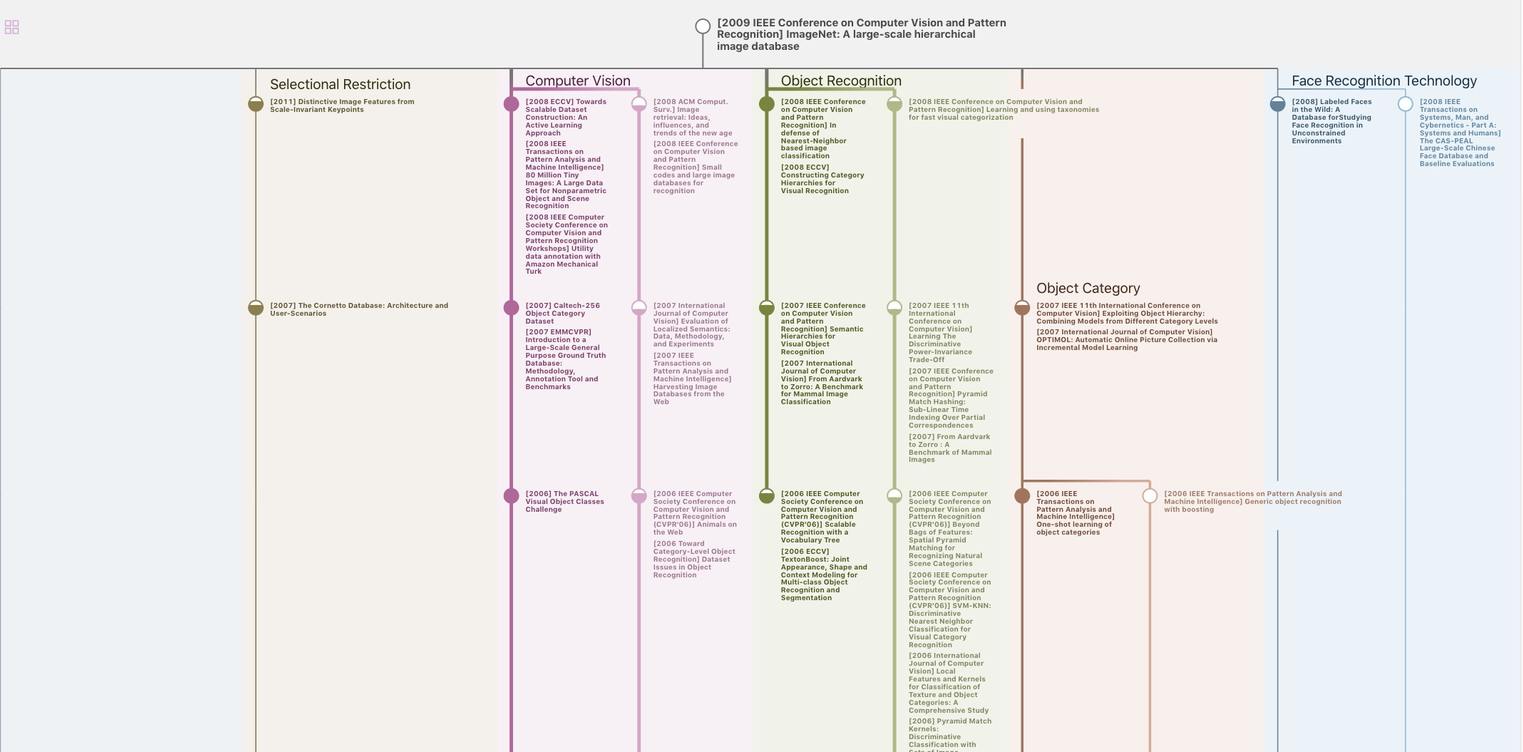
生成溯源树,研究论文发展脉络
Chat Paper
正在生成论文摘要