Neural PCA for Flow-Based Representation Learning
European Conference on Artificial Intelligence (ECAI)(2022)
摘要
Recent theoretical studies illustrated that kernel ridgeless regression can guarantee good generalization ability without an explicit regularization. In this paper, we investigate the statistical properties of ridgeless regression with random features and stochastic gradient descent. We explore the effect of factors in the stochastic gradient and random features, respectively. Specifically, random features error exhibits the double-descent curve. Motivated by the theoretical findings, we propose a tunable kernel algorithm that optimizes the spectral density of kernel during training. Our work bridges the interpolation theory and practical algorithm.
更多查看译文
关键词
neural pca,representation,learning,flow-based
AI 理解论文
溯源树
样例
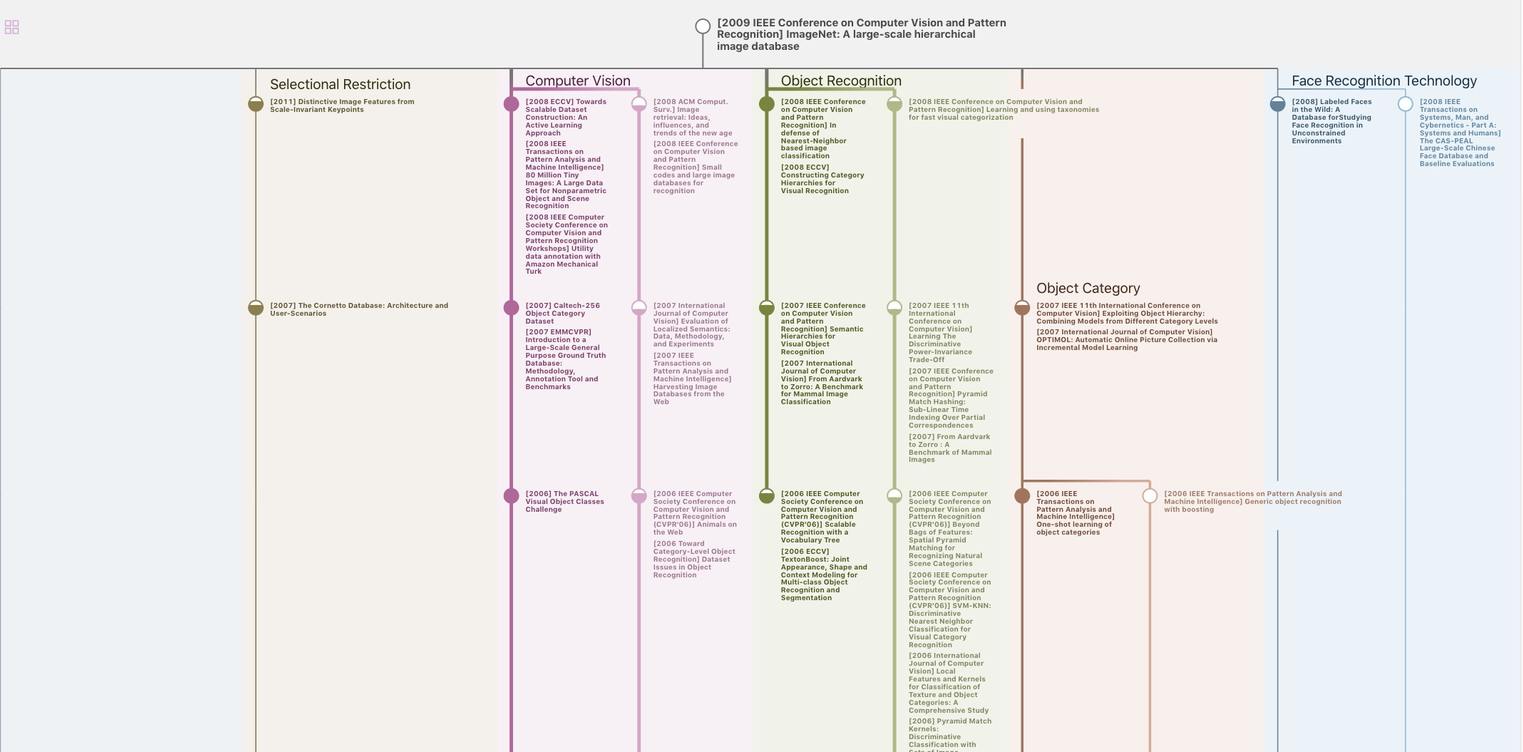
生成溯源树,研究论文发展脉络
Chat Paper
正在生成论文摘要