Neighborhood Focused Critic Policy Gradients for Multi-agent Reinforcement Learning
2022 International Conference on Computer Engineering and Artificial Intelligence (ICCEAI)(2022)
摘要
Centralized Training and Decentralized Exucetion (CTDE) is typical training pattern in multi-agent reinforcement learning. In actor-critic method, the actor updates based on the centralized critic which takes global state as criteria for individual contribution. While in cooperative multi-agent tasks, estimating individual contribution in the range of all agents leads to the overlook of local coordination, and thus intensify the mislead in credit assignment. This paper propose Neighbourhood Focused Critic (NFC) Policy Gradients for multi-agent reinforcement learning, parameterizing critic by neighbourhood focused graph neural network in Actor-critic method. NFC allows the centralized critic focus on local coordination in each agent's neighbourhood, and decentralized actors optimizes policies according to the critic estimation, thus alleviating the credit assignment problem. We test our NFC in StarCraft Multi-Agent Challenge (SMAC) environment, the results show that it improves performance and convergence speed significantly comparing to methods with globally focused critic.
更多查看译文
关键词
multi-agent reinforcement learning,graph neural network,cooperative games
AI 理解论文
溯源树
样例
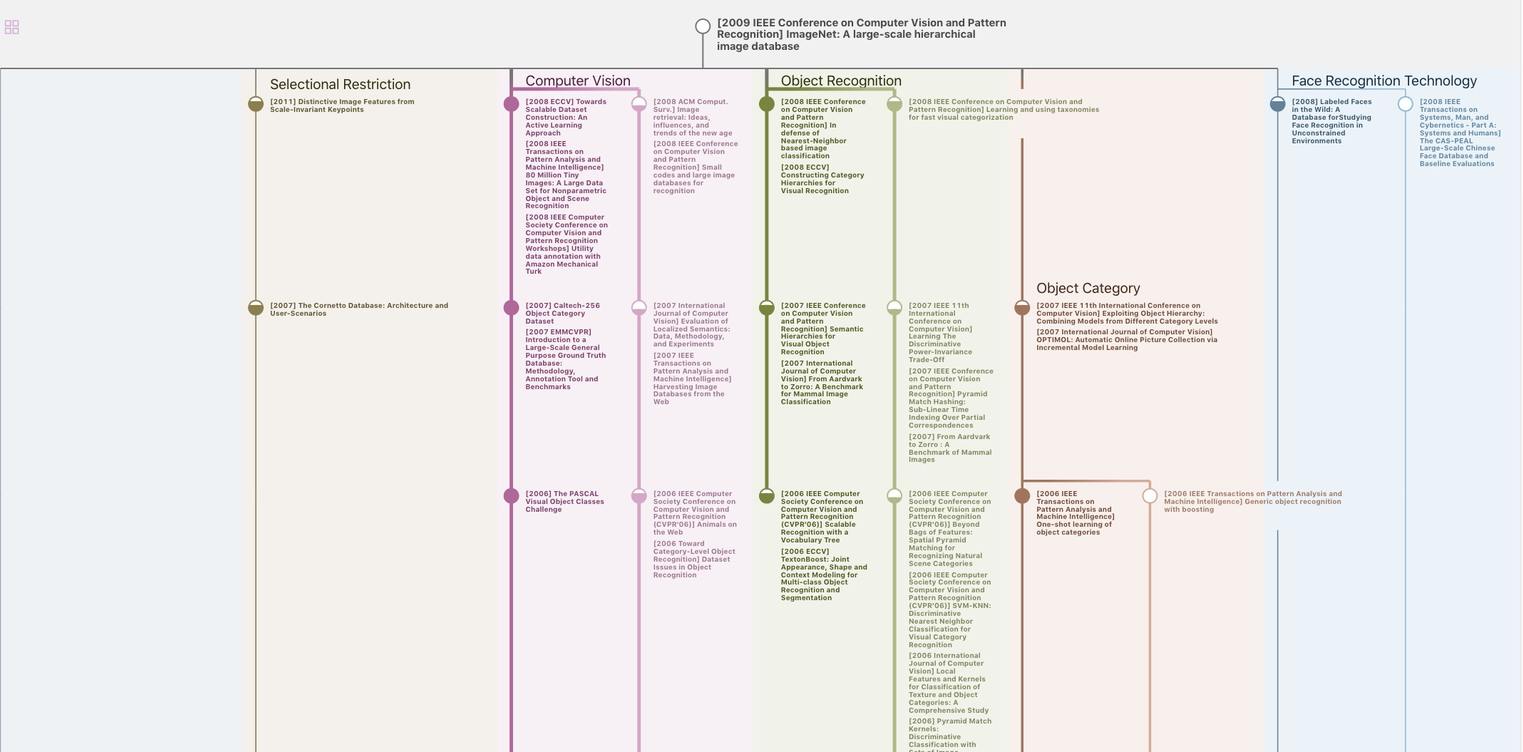
生成溯源树,研究论文发展脉络
Chat Paper
正在生成论文摘要