Detecting Non-control-flow Hijacking Threats with Deep Autoencoding Gaussian Mixture Model
2022 4th International Conference on Communications, Information System and Computer Engineering (CISCE)(2022)
摘要
Non-control-flow hijacking attacks have been an emerging threat for current computer system security. Attackers leverage data manipulation in memory to corrupt the integrity of the target program without invalidating the normal control-flow during execution. Consequently, current sandox-based methods towards mitigating control-oriented attacks cannot identify non-control-flow hijacking attacks. In this paper, we proposed a data-driven methology to distinguish the abnormity for non-control-flow hijacking detection. We adopt a deep autoencoding gaussian mixture model architecture that leverages the hardware events and corresponding contextual information during execution. The proposed framework has shown an outstanding performance through comparative experiments with previous literatured works.
更多查看译文
关键词
non-control-flow hijacking,deep autoencoding gaussian mixture model,malware detection,unsupervised learning
AI 理解论文
溯源树
样例
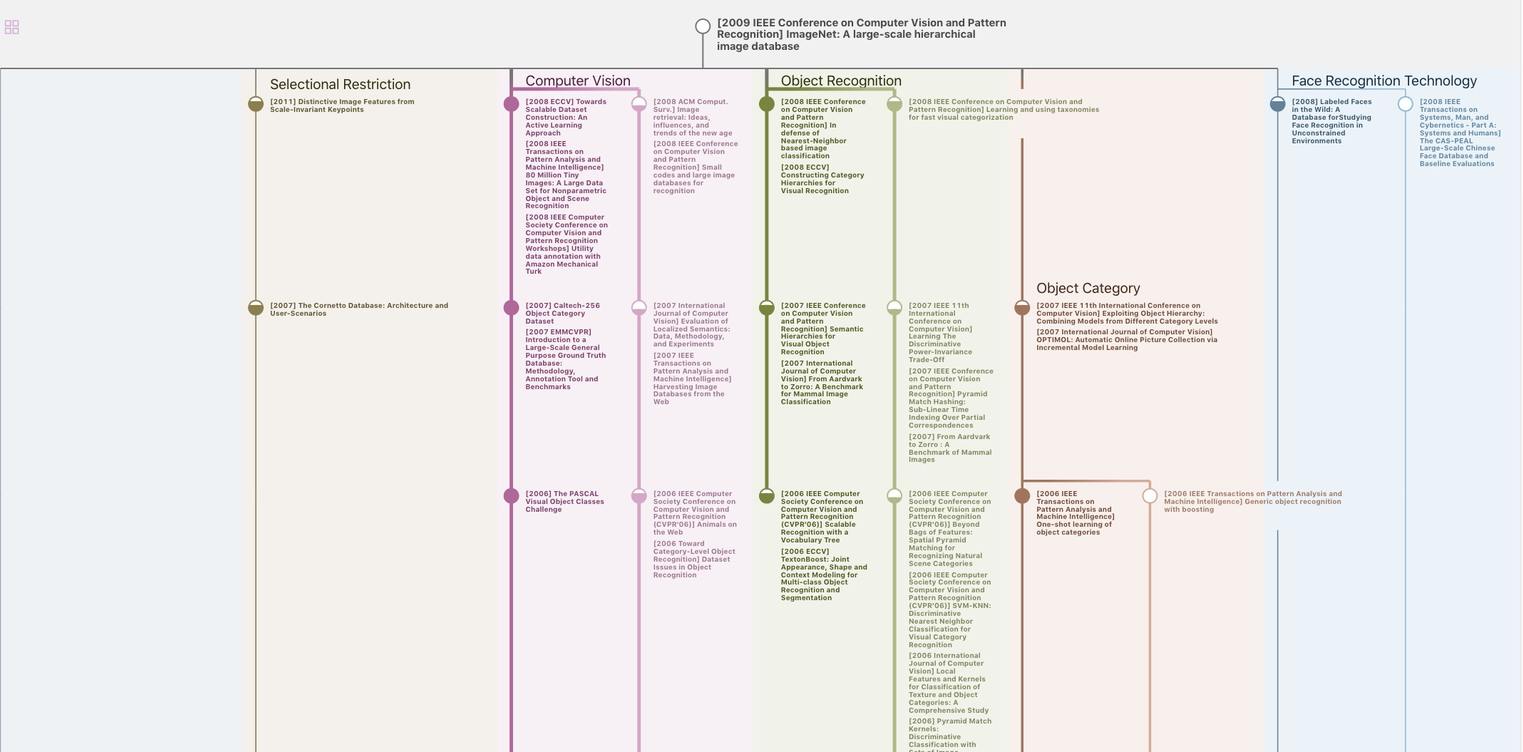
生成溯源树,研究论文发展脉络
Chat Paper
正在生成论文摘要