Image Varifolds on Meshes for Mapping Spatial Transcriptomics
arxiv(2022)
摘要
Advances in the development of largely automated microscopy methods such as MERFISH for imaging cellular structures in mouse brains are providing spatial detection of micron resolution gene expression. While there has been tremendous progress made in the field Computational Anatomy (CA) to perform diffeomorphic mapping technologies at the tissue scales for advanced neuroinformatic studies in common coordinates, integration of molecular- and cellular-scale populations through statistical averaging via common coordinates remains yet unattained. This paper describes the first set of algorithms for calculating geodesics in the space of diffeomorphisms, what we term Image-Varifold LDDMM,extending the family of large deformation diffeomorphic metric mapping (LDDMM) algorithms to accommodate the "copy and paste" varifold action of particles which extends consistently to the tissue scales. We represent the brain data as geometric measures, termed as {\em image varifolds} supported by a large number of unstructured points, % (i.e., not aligned on a 2D or 3D grid), each point representing a small volume in space % (which may be incompletely described) and carrying a list of densities of {\em features} elements of a high-dimensional feature space. The shape of image varifold brain spaces is measured by transforming them by diffeomorphisms. The metric between image varifolds is obtained after embedding these objects in a linear space equipped with the norm, yielding a so-called "chordal metric."
更多查看译文
关键词
mapping spatial transcriptomics,meshes
AI 理解论文
溯源树
样例
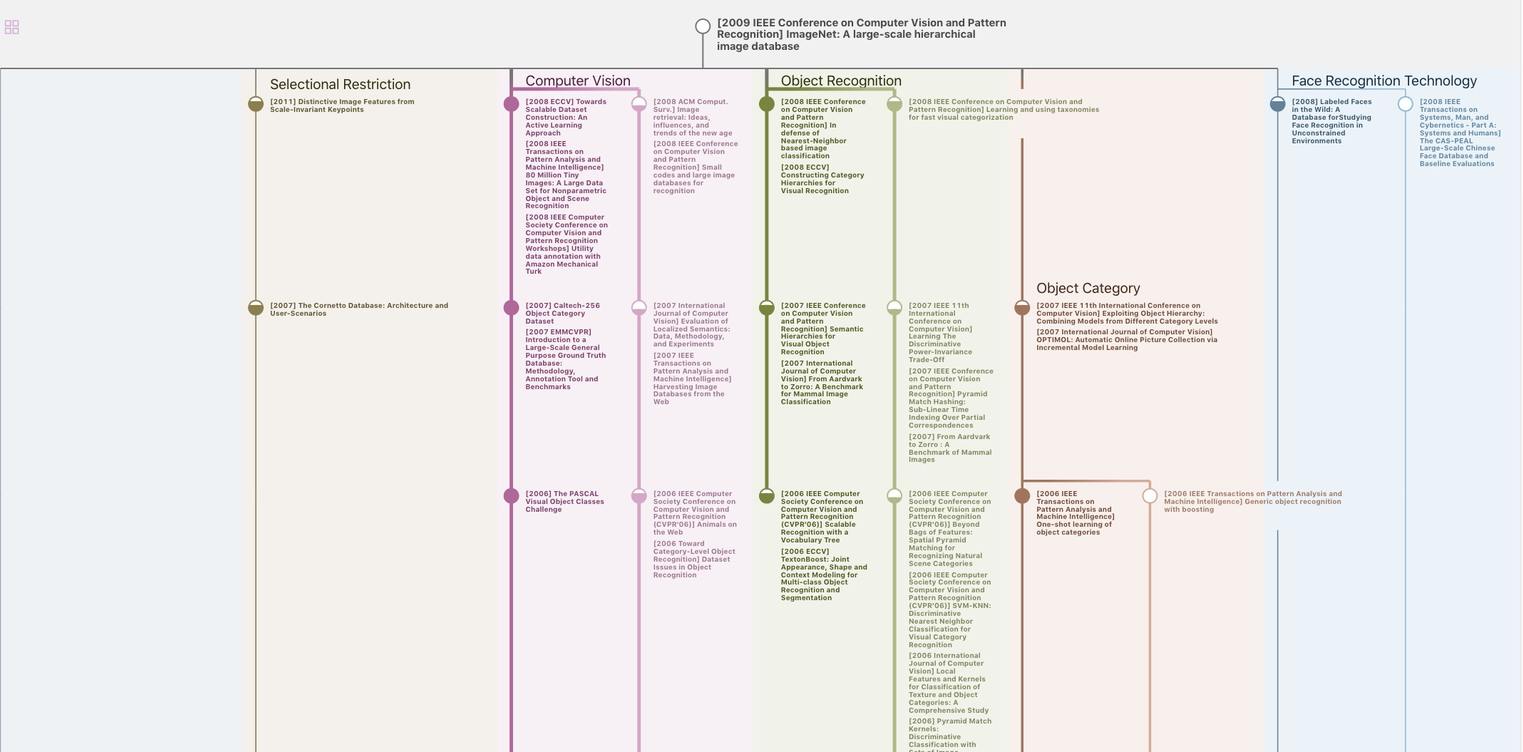
生成溯源树,研究论文发展脉络
Chat Paper
正在生成论文摘要