ODformer: Spatial-Temporal Transformers for Long Sequence Origin-Destination Matrix Forecasting Against Cross Application Scenario
arxiv(2022)
摘要
Origin-Destination (OD) matrices record directional flow data between pairs of OD regions. The intricate spatiotemporal dependency in the matrices makes the OD matrix forecasting (ODMF) problem not only intractable but also non-trivial. However, most of the related methods are designed for very short sequence time series forecasting in specific application scenarios, which cannot meet the requirements of the variation in scenarios and forecasting length of practical applications. To address these issues, we propose a Transformer-like model named ODformer, with two salient characteristics: (i) the novel OD Attention mechanism, which captures special spatial dependencies between OD pairs of the same origin (destination), greatly improves the ability of the model to predict cross-application scenarios after combining with 2D-GCN that captures spatial dependencies between OD regions. (ii) a PeriodSparse Self-attention that effectively forecasts long sequence OD matrix series while adapting to the periodic differences in different scenarios. Generous experiments in three application backgrounds (i.e., transportation traffic, IP backbone network traffic, crowd flow) show our method outperforms the state-of-the-art methods.
更多查看译文
关键词
forecasting,spatial-temporal,origin-destination
AI 理解论文
溯源树
样例
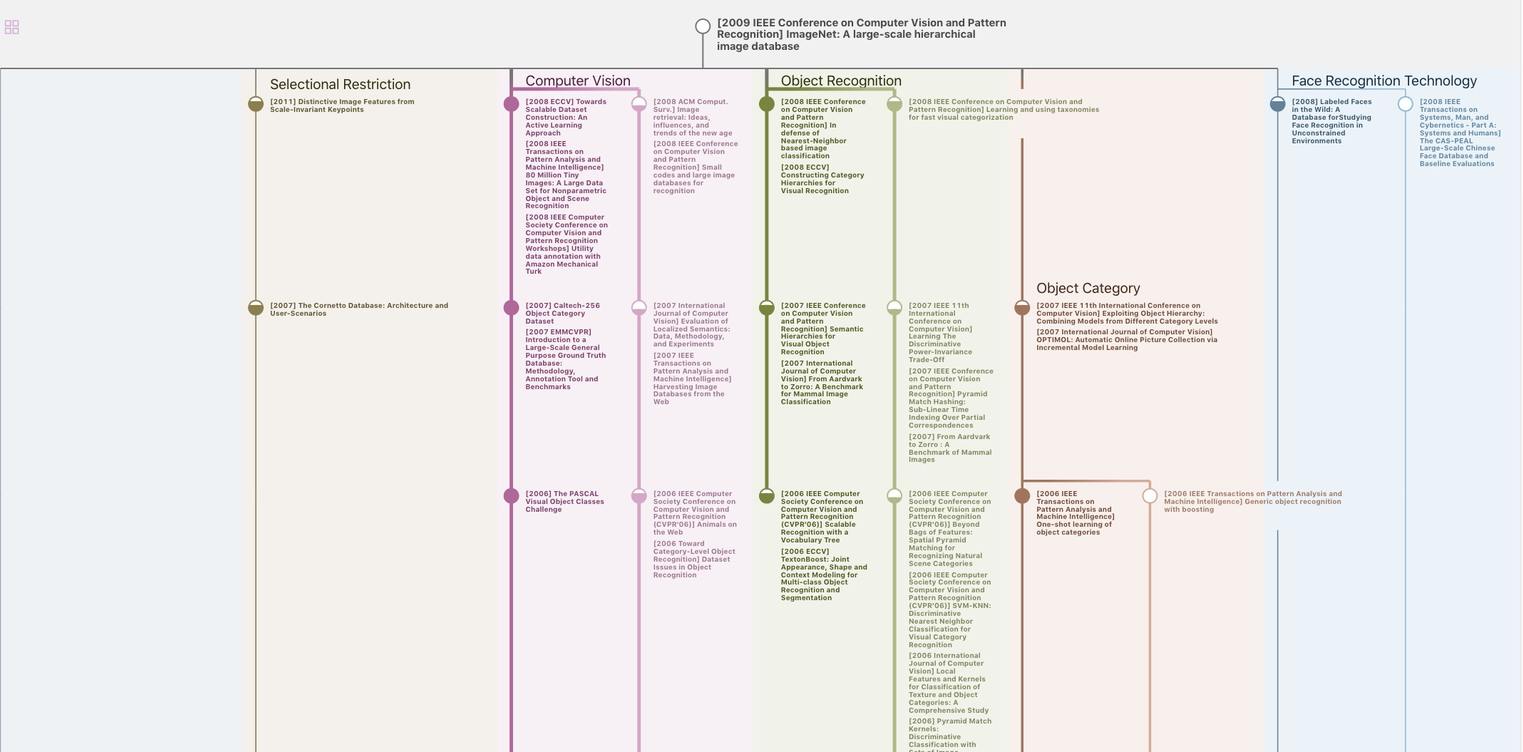
生成溯源树,研究论文发展脉络
Chat Paper
正在生成论文摘要