NMPC-LBF: Nonlinear MPC with Learned Barrier Function for Decentralized Safe Navigation of Multiple Robots in Unknown Environments.
IEEE/RJS International Conference on Intelligent RObots and Systems (IROS)(2022)
摘要
In this paper, we present a decentralized control approach based on a Nonlinear Model Predictive Control (NMPC) method that employs barrier certificates for safe navigation of multiple nonholonomic wheeled mobile robots in unknown environments with static and/or dynamic obstacles. This method incorporates a Learned Barrier Function (LBF) into the NMPC design in order to guarantee safe robot navigation, i.e., prevent robot collisions with other robots and the obstacles. We refer to our proposed control approach as NMPC-LBF. Since each robot does not have a priori knowledge about the obstacles and other robots, we use a Deep Neural Network (DeepNN) running in real-time on each robot to learn the Barrier Function (BF) only from the robot's LiDAR and odometry measurements. The DeepNN is trained to learn the BF that separates safe and unsafe regions. We implemented our proposed method on simulated and actual Turtlebot3 Burger robot(s) in different scenarios. The implementation results show the effectiveness of the NMPC-LBF method at ensuring safe navigation of the robots.
更多查看译文
关键词
nonlinear nmpc-lbf,learned barrier function,multiple robots,safe navigation
AI 理解论文
溯源树
样例
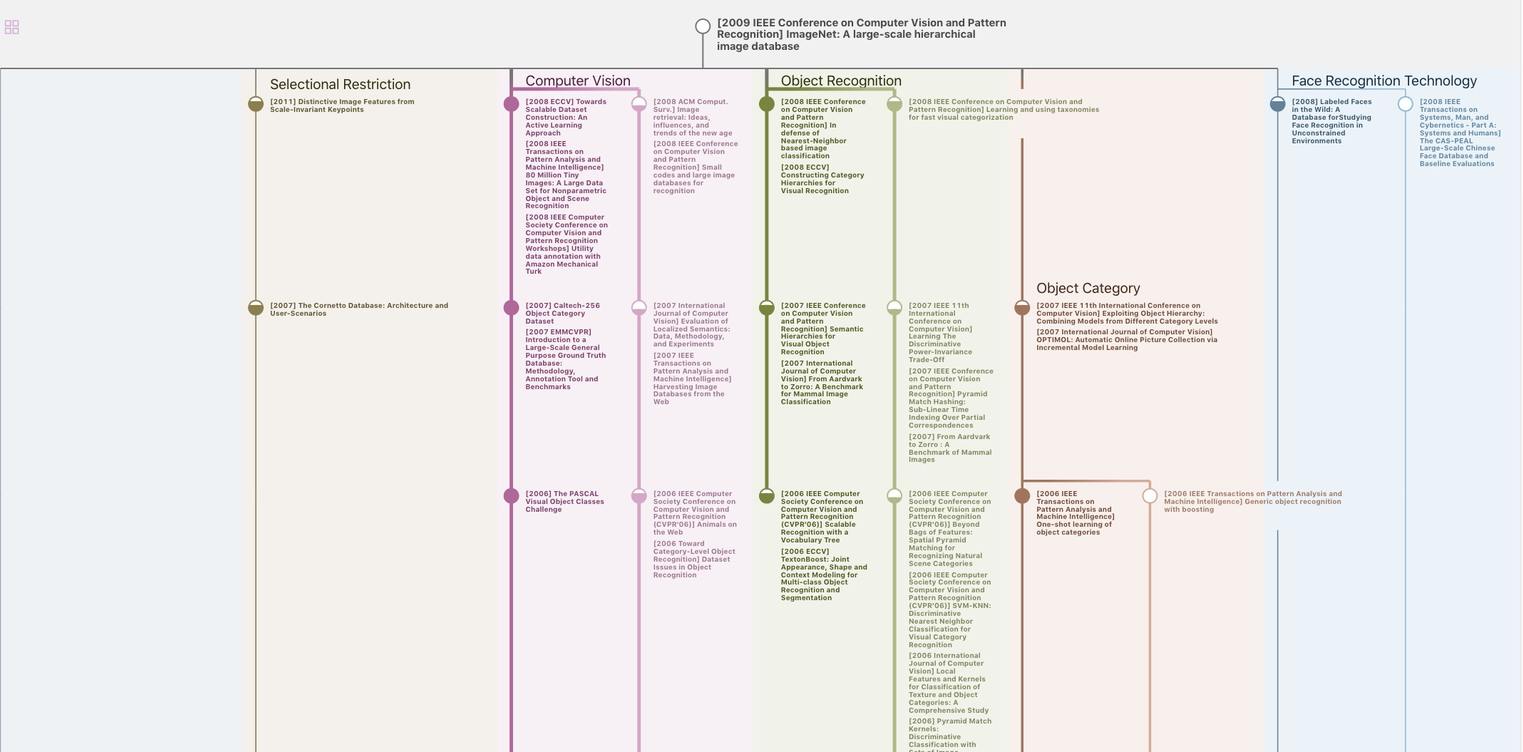
生成溯源树,研究论文发展脉络
Chat Paper
正在生成论文摘要