An integrated approach to test for missing not at random
arxiv(2022)
摘要
Missing data can lead to inefficiencies and biases in analyses, in particular when data are missing not at random (MNAR). It is thus vital to understand and correctly identify the missing data mechanism. Recovering missing values through a follow up sample allows researchers to conduct hypothesis tests for MNAR, which are not possible when using only the original incomplete data. Investigating how properties of these tests are affected by the follow up sample design is little explored in the literature. Our results provide comprehensive insight into the properties of one such test, based on the commonly used selection model framework. We determine conditions for recovery samples that allow the test to be applied appropriately and effectively, i.e. with known Type I error rates and optimized with respect to power. We thus provide an integrated framework for testing for the presence of MNAR and designing follow up samples in an efficient cost-effective way. The performance of our methodology is evaluated through simulation studies as well as on a real data sample.
更多查看译文
关键词
test,missing
AI 理解论文
溯源树
样例
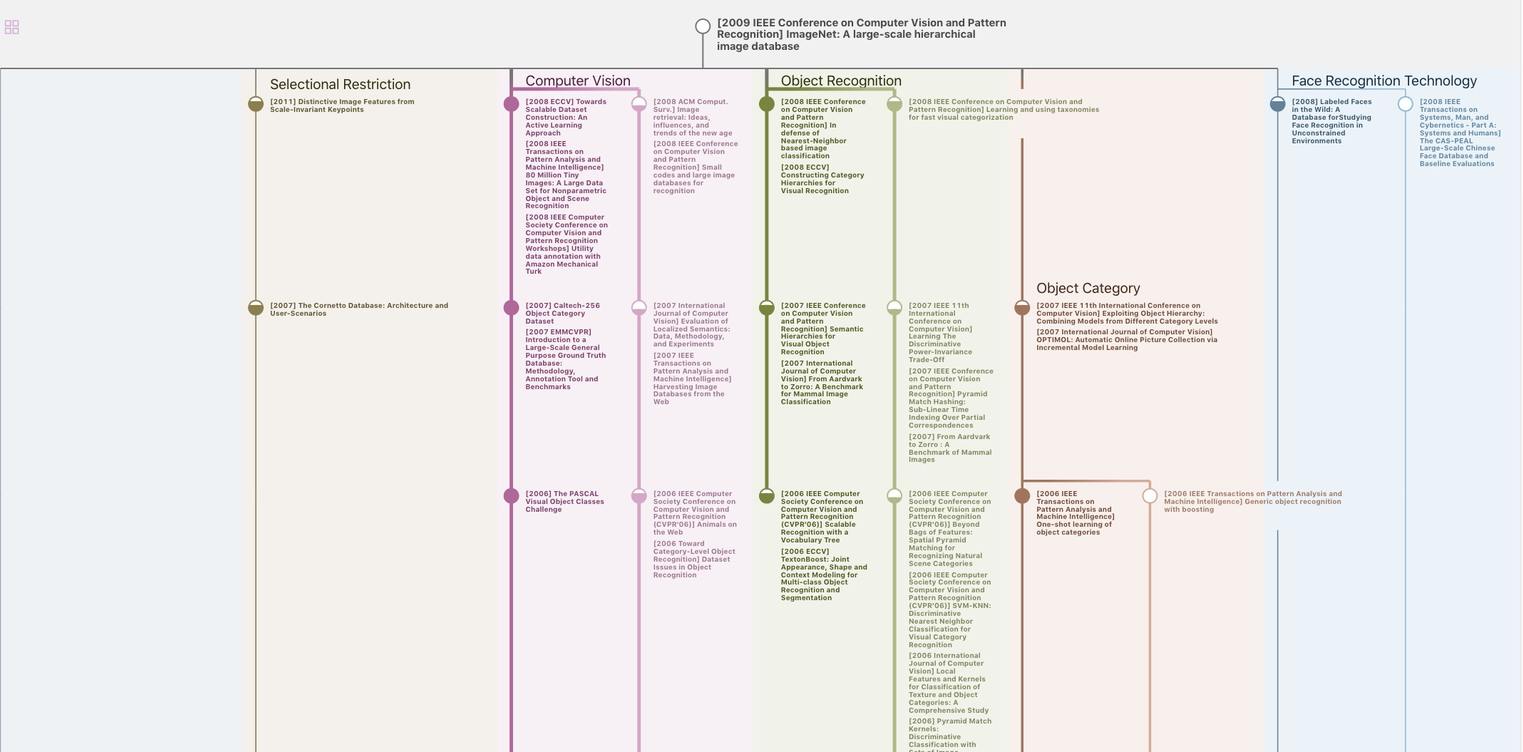
生成溯源树,研究论文发展脉络
Chat Paper
正在生成论文摘要