Private Query Release via the Johnson-Lindenstrauss Transform.
SODA(2023)
摘要
We introduce a new method for releasing answers to statistical queries with differential privacy, based on the Johnson-Lindenstrauss lemma. The key idea is to randomly project the query answers to a lower dimensional space so that the distance between any two vectors of feasible query answers is preserved up to an additive error. Then we answer the projected queries using a simple noise-adding mechanism, and lift the answers up to the original dimension. Using this method, we give, for the first time, purely differentially private mechanisms with optimal worst case sample complexity under average error for answering a workload of $k$ queries over a universe of size $N$. As other applications, we give the first purely private efficient mechanisms with optimal sample complexity for computing the covariance of a bounded high-dimensional distribution, and for answering 2-way marginal queries. We also show that, up to the dependence on the error, a variant of our mechanism is nearly optimal for every given query workload.
更多查看译文
关键词
private query release,johnson-lindenstrauss
AI 理解论文
溯源树
样例
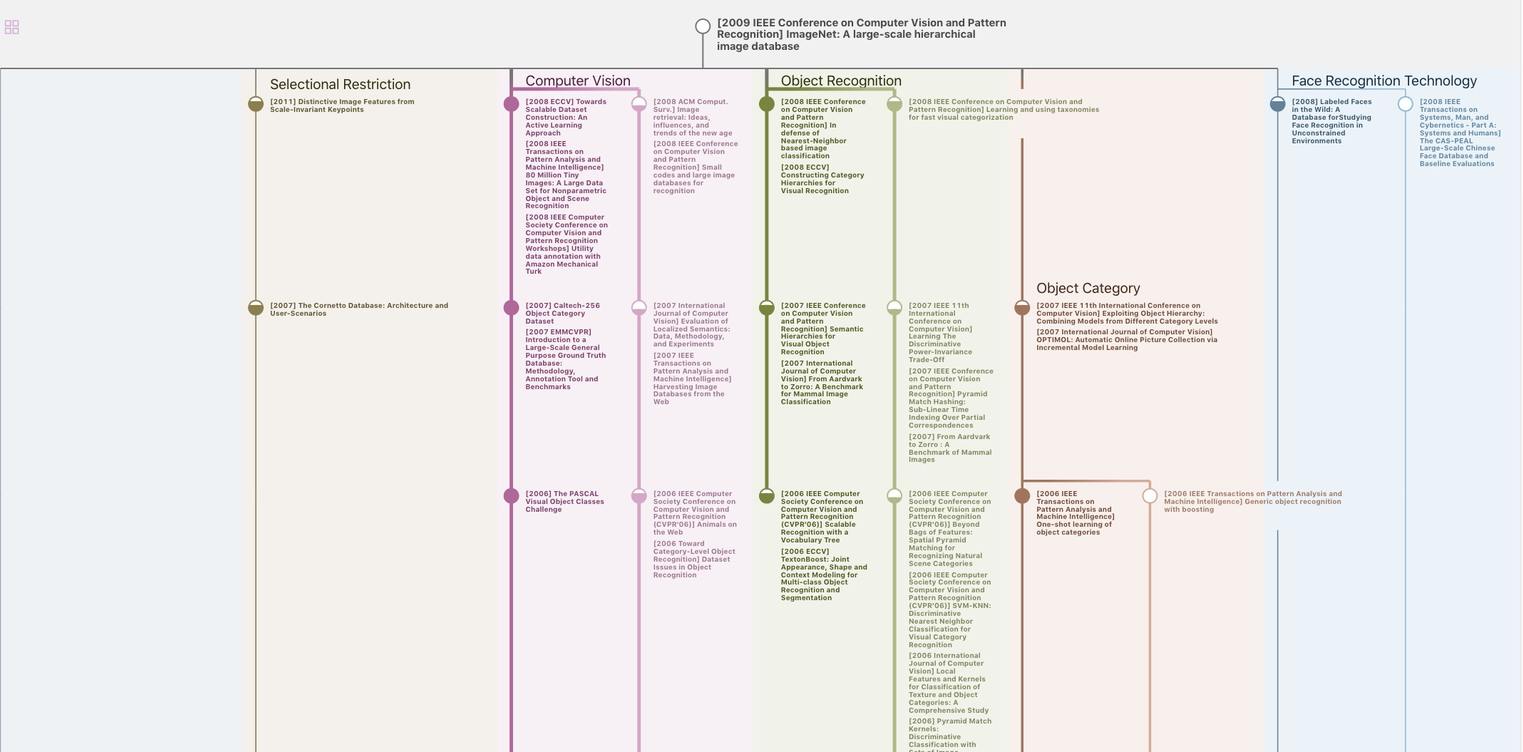
生成溯源树,研究论文发展脉络
Chat Paper
正在生成论文摘要