Data-Driven Network Performance Prediction for B5G Networks: A Graph Neural Network Approach
2022 IEEE Ninth International Conference on Communications and Electronics (ICCE)(2022)
摘要
Extreme connectivity, dynamic resource provisioning and demand of quality assurance in 5G and Beyond 5G(B5G) networks calls for advance network modeling solutions. We need functional network models that are able to produce accurate prediction of Key Performance Indicators (KPI) such as latency, overall delay, jitter or packet loss at low cost. Graph Neural Networks (GNN) have already shown great potential for network performance prediction, because of their ability to understand the network configurations. In this paper, we focus on improving the generalization capabilities of GNN in relatively complex IP transport network scenarios of future generation networks. We take RouteNet GNN as a reference model and present an alternative GNN. We train both models with relatively smaller network scenarios while for evaluation we use complex and large network configurations. After hyper-parameter tuning for RouteNet and proposed GNN, the results show that our model outperforms baseline architecture in evaluation phase. The validation losses for scenarios not seen during training phase, are significantly lower than the RouteNet.
更多查看译文
关键词
Deep Learning,GNN,5G,B5G,Network Modeling
AI 理解论文
溯源树
样例
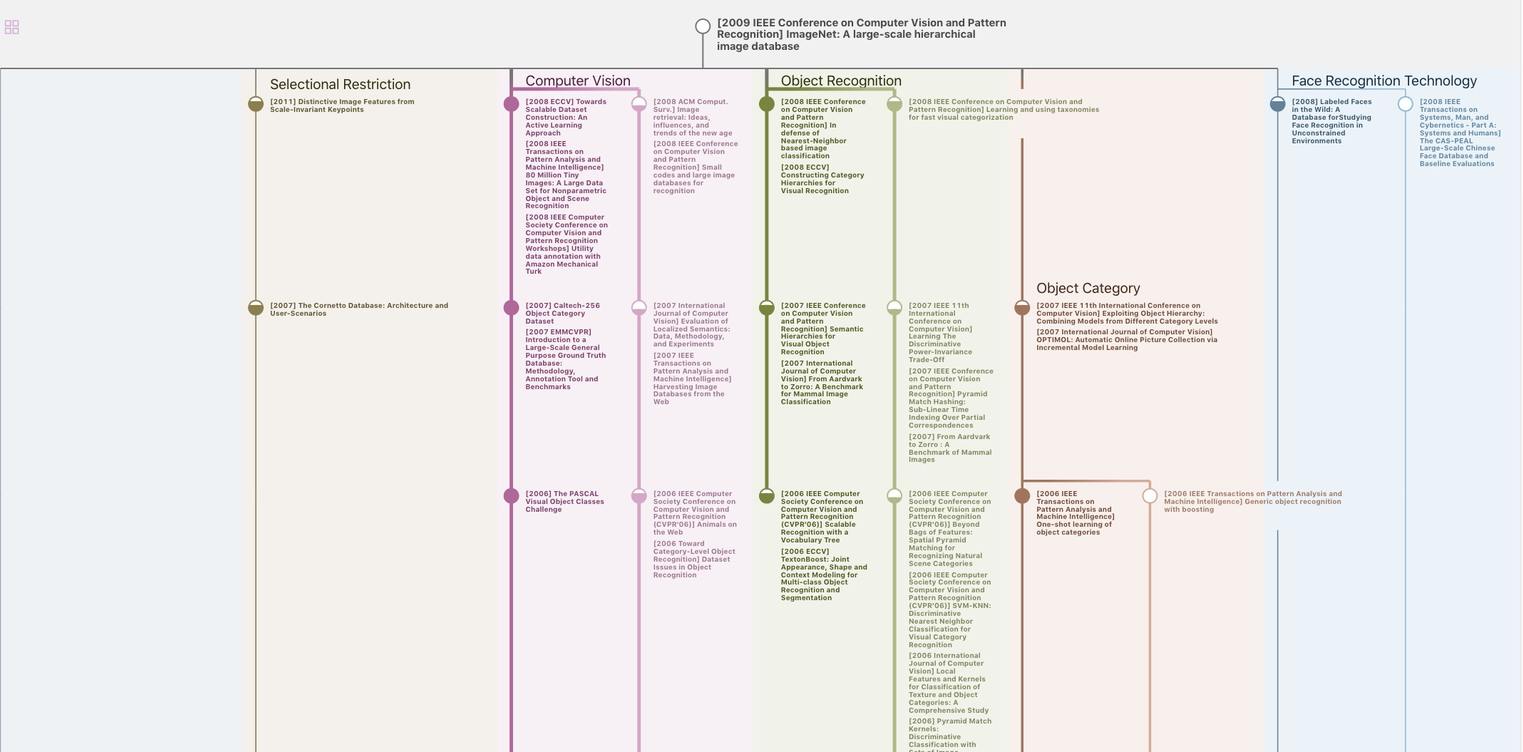
生成溯源树,研究论文发展脉络
Chat Paper
正在生成论文摘要