A "data-feature-policy" solution for multiscale geological-geophysical intelligent reservoir characterization
Second International Meeting for Applied Geoscience & Energy(2022)
摘要
PreviousNext You have accessSecond International Meeting for Applied Geoscience & EnergyA "data-feature-policy" solution for multiscale geological-geophysical intelligent reservoir characterizationAuthors: Wenhao ZhengFei TianQingyun DiJiangyun ZhangHui ZhouWang ZhangZhongxing WangWenhao ZhengChinese Academy of SciencesSearch for more papers by this author, Fei TianChinese Academy of SciencesSearch for more papers by this author, Qingyun DiChinese Academy of SciencesSearch for more papers by this author, Jiangyun ZhangChinese Academy of SciencesSearch for more papers by this author, Hui ZhouPetroChinaSearch for more papers by this author, Wang ZhangChinese Academy of SciencesSearch for more papers by this author, and Zhongxing WangChinese Academy of SciencesSearch for more papers by this authorhttps://doi.org/10.1190/image2022-3748948.1 SectionsAboutPDF/ePub ToolsAdd to favoritesDownload CitationsTrack CitationsPermissions ShareFacebookTwitterLinked InRedditEmail AbstractInnovative interpretation methods based on efficient measurements are the key to establishing accurate “geological-geophysical” mappings. The multi-solvability of petrophysical responses leads to the complex non-linear relationship between multi-scale geophysical data and the classification of strongly heterogeneous geological bodies, which makes it difficult for quantitative reservoir characterization. As multi-source geological-geophysical data have inherent differences in resolution and properties, how to effectively fuse these data becomes a real challenge in the big data era.Based on understanding the intrinsic correlation of different measurements on the given geo-body, a combination of machine learning algorithms was established for multi-scale geological-geophysical intelligent reservoir characterization, which was named “Data-Feature-Policy” solution. (1) Data-level fusion: The dataset was obtained from the conventional logging data of 921m deep carbonate rock in the Tarim Basin. Firstly, the Isolated Forest algorithm was used for quality control to remove outliers, and then, the multi-dimensional parameters were reduced to a 2-dimensional embedding vector by t-SNE algorithm. (2) Feature-level extraction: Based on the Density Peak Clustering algorithm, non-spherical clusters of 2-dimensional embedding vectors were clustered. Then, calibrated by the core-electrical imaging chart, each cluster was labeled electrofacies classification; (3) Policy-level characterization: Based on Deep Belief Network, the geological prediction model was established. It was optimized by a double-loop filtering mechanism that selected the parameter-cluster combination with the highest correct rate, provided that each accuracy is greater than 80%. And the final accuracy rate exceeded 93%. The model was used to quantitatively identify the 3176m carbonate reservoir, automatically count the development thickness and distribution range of reservoir classification, which clarified the reservoir vertical structure and distribution around the wellbore.The “Data-Feature-Policy” solution has the advantages of data-driven, objectivity, and compatibility. It can dig high-dimensional mapping relationships of geological-geophysical data at the feature level, which helps to better understand the multi-variate and multi-attribute data fusion. This effective workflow is suitable to characterize heterogeneous reservoirs intelligently.Note: This paper was accepted into the Technical Program but was not presented at IMAGE 2022 in Houston, Texas.Keywords: data-feature-policy, machine learning, reservoir characterizationPermalink: https://doi.org/10.1190/image2022-3748948.1FiguresReferencesRelatedDetails Second International Meeting for Applied Geoscience & EnergyISSN (print):1052-3812 ISSN (online):1949-4645Copyright: 2022 Pages: 3694 publication data© 2022 Published in electronic format with permission by the Society of Exploration Geophysicists and the American Association of Petroleum GeologistsPublisher:Society of Exploration Geophysicists HistoryPublished: 15 Aug 2022 CITATION INFORMATION Wenhao Zheng, Fei Tian, Qingyun Di, Jiangyun Zhang, Hui Zhou, Wang Zhang, and Zhongxing Wang, (2022), "A "data-feature-policy" solution for multiscale geological-geophysical intelligent reservoir characterization," SEG Technical Program Expanded Abstracts : 3272-3276. https://doi.org/10.1190/image2022-3748948.1 Plain-Language Summary Keywordsdata-feature-policymachine learningreservoir characterizationPDF DownloadLoading ...
更多查看译文
关键词
reservoir characterization,data-feature-policy,geological-geophysical
AI 理解论文
溯源树
样例
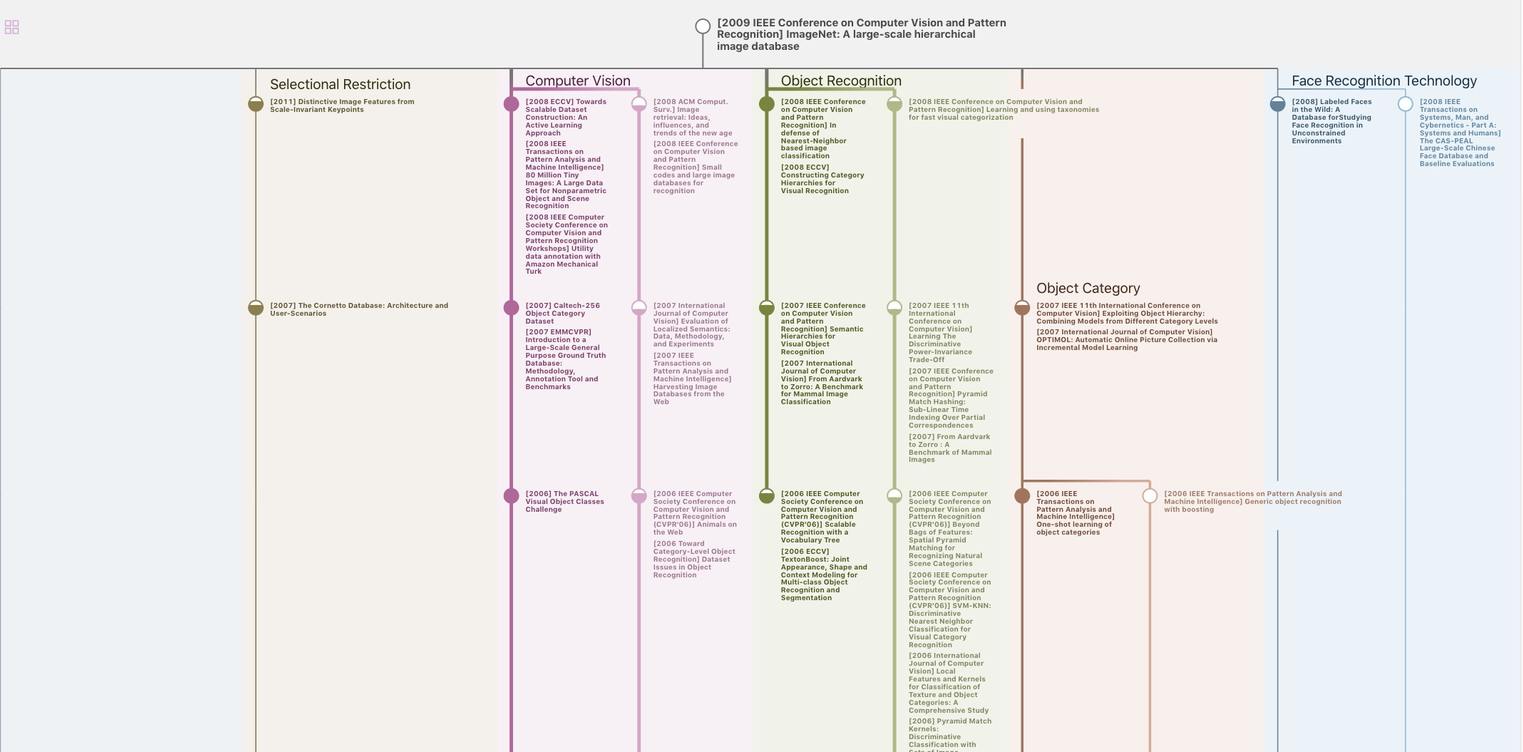
生成溯源树,研究论文发展脉络
Chat Paper
正在生成论文摘要